Lipoprotein(a) and cardiovascular disease: prediction, attributable risk fraction and estimating benefits from novel interventions
medRxiv (Cold Spring Harbor Laboratory)(2020)
摘要
AbstractBackgroundLipoprotein (a) (Lp(a)) is a CVD risk factor amenable to intervention and might help guide risk prediction.ObjectivesTo investigate the population attributable fraction due to elevated Lp(a) and its utility in risk prediction.MethodsUsing a prospective cohort study, 413,724 participants from UK Biobank, associations of serum Lp(a) with composite fatal/nonfatal CVD (n=10,065 events), fatal CVD (n=3247), coronary heart disease (n=16,649), ischaemic stroke (n=3191), and peripheral vascular disease (n=2716) were compared using Cox models. Predictive utility was determined by C-index changes. The population attributable fraction was estimated.ResultsMedian Lp(a) was 19.7nmol/L (interquartile interval 7.6-75.3nmol/L). 20.8% had Lp(a) values >100nmol/L; 9.2% had values >175nmol/L. After adjustment for classical risk factors, in participants with no baseline CVD and not taking a statin, 1 standard deviation increment in log Lp(a) was associated with a HR for fatal/nonfatal CVD of 1.09 (95%CI 1.07-1.11). Associations were similar for fatal CVD, coronary heart disease, and peripheral vascular disease. Adding Lp(a) to a prediction model containing traditional CVD risk factors improved the C-index by +0.0017 (95% CI 0.0009, 0.0026). We estimated that having Lp(a) values >100nmol/L accounts for 5.7% of CVD events in the whole cohort. We modelled that an ongoing trial to lower Lp(a) in patients with CVD and Lp(a) above ∼175nmol/L may reduce CVD risk by 20.3%, assuming causality, and an achieved Lp(a) reduction of 80%.ConclusionsPopulation screening for elevated Lp(a) may help to predict CVD and target Lp(a) lowering drugs, if such drugs prove efficacious, to those with markedly elevated levels.
更多查看译文
关键词
cardiovascular disease,attributable risk fraction,interventions
AI 理解论文
溯源树
样例
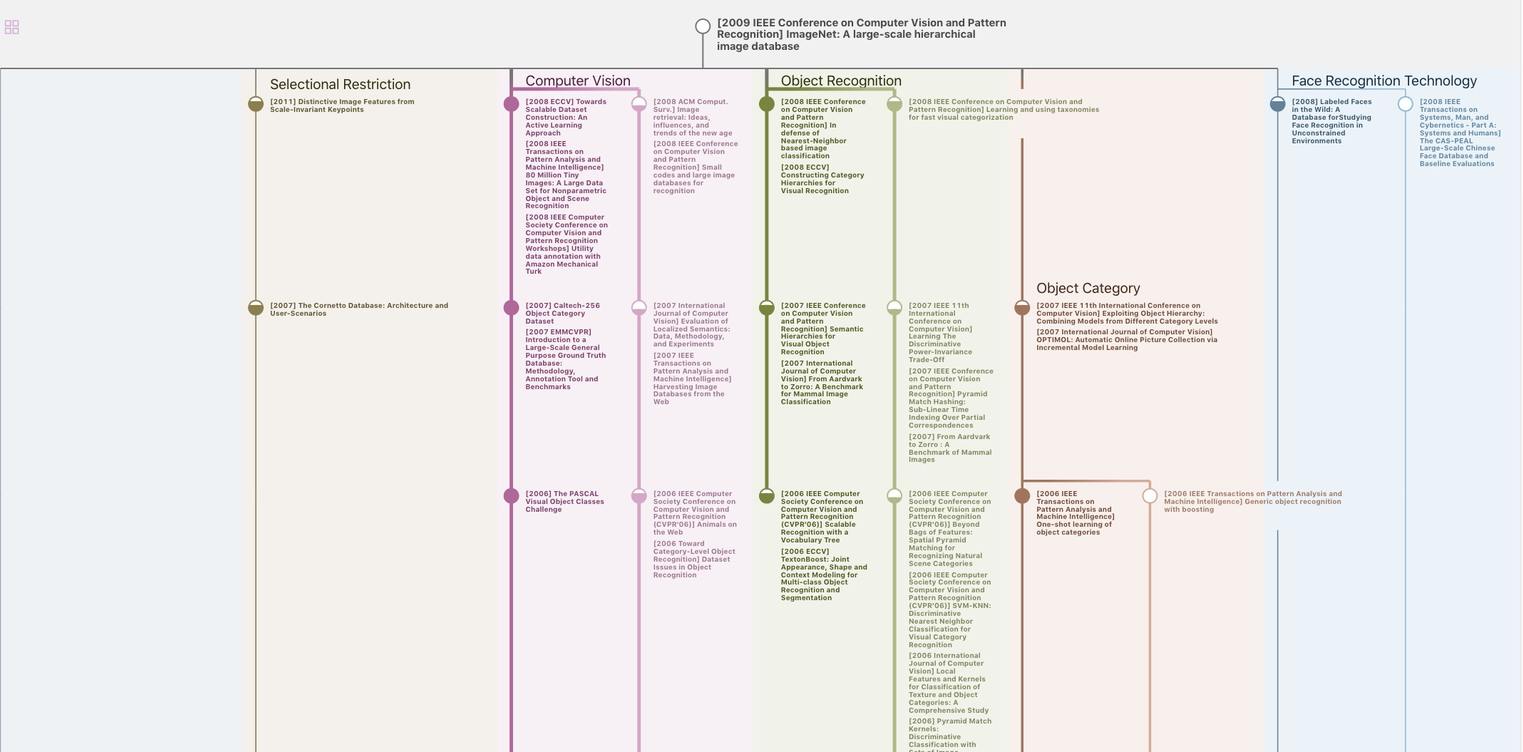
生成溯源树,研究论文发展脉络
Chat Paper
正在生成论文摘要