Fusion and mining of glacier surface flow velocity time series
crossref(2020)
摘要
<p>Glacier response to climate change results in natural hazards, sea level rise and changes in freshwater resources. To evaluate this response, glacier surface flow velocity constitutes a crucial parameter to study. Nowadays, more and more velocity maps at regional or global scales issued from satellite SAR and/or optical images tend to be available online or on-demand. Such amount of data requires appropriate data fusion strategies in order to generate displacement time series with improved precision and spatio-temporal coverage. The improved displacement time series can then be used by advanced multi-temporal analysis approaches for further physical interpretations of the phenomenon under observation. In this work, time series of Sentinel-2 (10~m resolution, every 5 days), Landsat-8 (15~m resolution, every 16 days) and Venus (5~m resolution, every 2 days) images acquired between January 2017 and September 2018, over the Fox glacier in the Southern Alps of New Zealand are investigated. Velocities are generated with an offset tracking technique using an automatic processing chain for every possible repeat cycles (2 days-100 days and 300 days to 400 days). Thousands of velocity maps are available, and they are subject to both uncertainty and data gaps. In order to produce a displacement time series as precise/complete as possible , we propose three fusion strategies: 1) use all the available Sentinel-2 displacement maps with different time spans. The goal is to construct a time series of displacement with respect to a common master by means of an inversion 2) take only Sentinel-2 displacement maps with as small time spans as possible, at the same time, keep as much as possible redundancy in the network to be able to construct a common master displacement time series by inversion 3) follow the previous strategy but use all available displacement maps from 3 sensors, with different temporal sampling and measurement precision taken into account. Afterwards, the common master displacement time series will be analysed by a data mining approach in order to extract unusual spatio-temporal patterns in the time series.</p>
更多查看译文
AI 理解论文
溯源树
样例
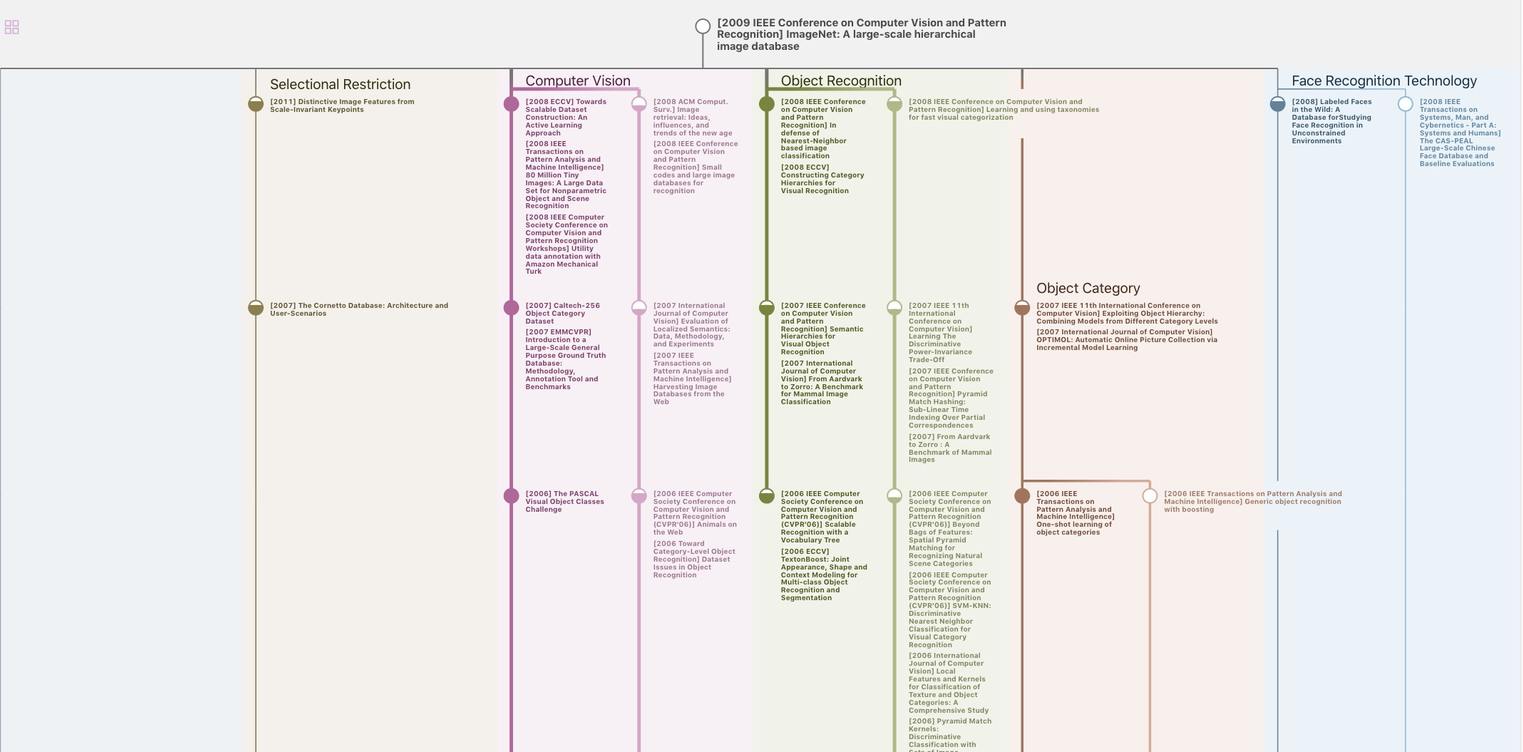
生成溯源树,研究论文发展脉络
Chat Paper
正在生成论文摘要