Spatial interpolation of daily precipitation using random forest
crossref(2020)
摘要
<p>Regression kriging is one of the most popular spatial interpolation techniques. Its main strength is that it exploits both spatial autocorrelation as well as information contained in environmental covariates. While regression kriging is still dominant, in the past few years machine learning, especially random forest, is increasingly being used for mapping. Machine learning is more flexible than multiple linear regression and can thus make better use of environmental covariates. But machine learning typically ignores spatial autocorrelation. Several attempts have been made to include spatial autocorrelation in random forest, by adding distances to observation locations and other geometries to the set of covariates. But none of these studies have tried the obvious solution to include the nearest observations themselves and the distances to the nearest observations as covariates. In this study we tried this solution by introducing and testing Random Forest for Spatial Interpolation (RFSI). RFSI trains a random forest model on environmental covariates as well as nearest observations and their distances from the prediction point. We applied and evaluated RFSI for mapping daily precipitation in Catalonia for the 2016-2018 period. We trained four models (random forests, RFsp, pooled regression kriging and RFSI) using 63,927 daily precipitation observations from 87 GHCN-stations located in Catalonia. Maximum and minimum daily temperatures and IMERG precipitation estimates (derived from the GPM mission) were used as environmental covariates for all four models. Results based on 5-fold cross validation showed that RFSI (R-square 69.4%, RMSE 3.8 mm) significantly outperformed all random forest (R-square 50.6%, RMSE 3.8 mm), RFsp (R-square 55.5%, RMSE 4.6 mm) and pooled regression kriging (R-square 65.3%, RMSE 4.0 mm). Finetuning RFSI could potentially improve prediction accuracy even more. In addition to improved prediction accuracy, RFSI has the advantage that it uses much fewer spatial covariates than RFsp.</p>
更多查看译文
AI 理解论文
溯源树
样例
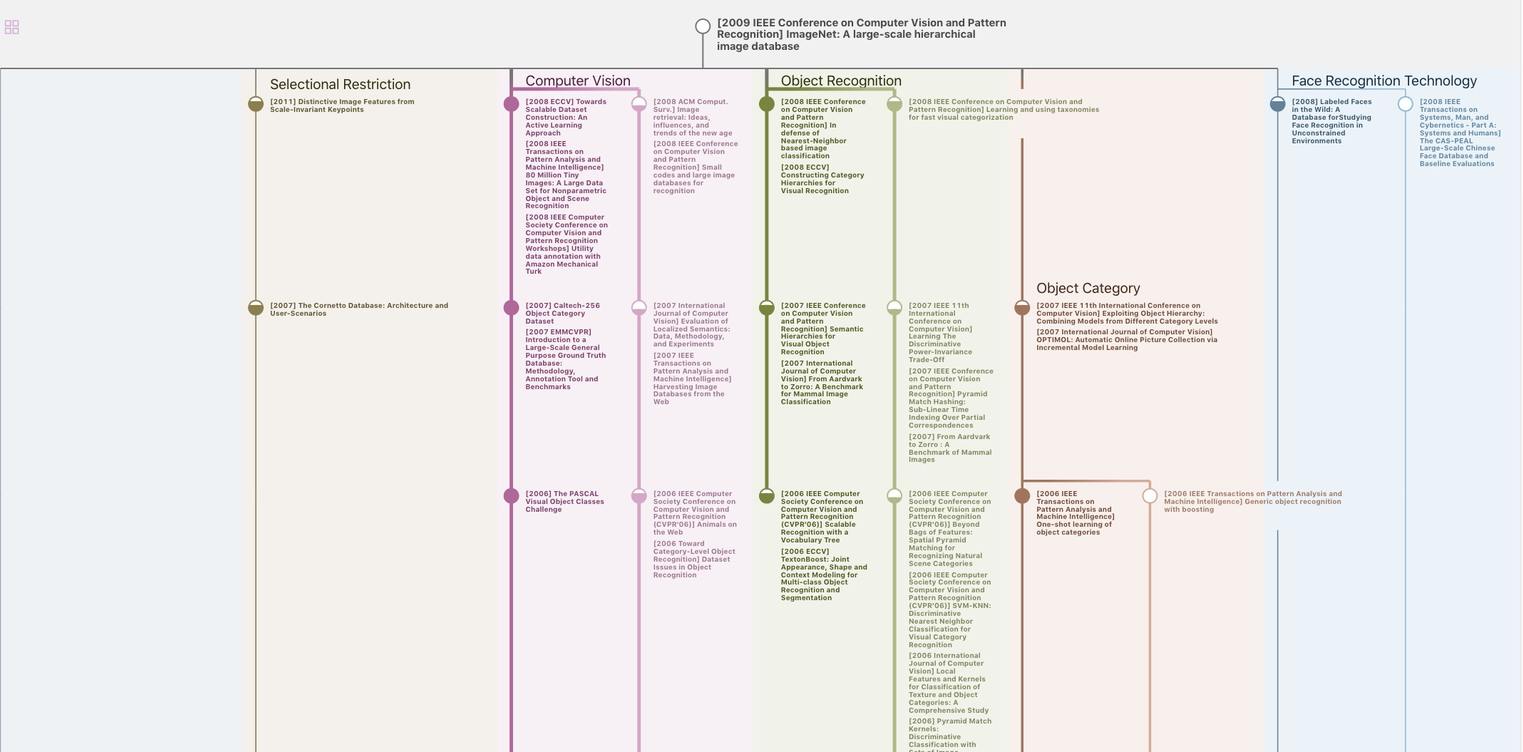
生成溯源树,研究论文发展脉络
Chat Paper
正在生成论文摘要