State tagging for improved earth and environmental data quality assurance
crossref(2020)
摘要
<p>Long-term monitoring data that considers a wide array of environmental variables provides key insights to environmental change because responses of ecosystem functions and services to environmental drivers are inherently long-term and strongly interlinked. To ensure that the data are reliable for analysis and interpretation, they must undergo quality assurance procedures. However, the expected or acceptable range of data values vary greatly as the state of the ecosystem changes. Current quality assurance procedures for environmental data take no consideration of the system state at which each measurement is made, and provide the user with little contextual information on the probable cause for a measurement to be flagged out of range. We propose the use of data science techniques to tag each measurement with an identified system state. The term “state” here is defined loosely and they are identified using k-means clustering, an unsupervised machine learning method. The meaning of the states is open to specialist interpretation. Once the states are identified, state-dependent prediction intervals can be calculated for each observational variable. This approach provides the user with more contextual information to resolve out-of-range flags and derive prediction intervals for observational variables that considers the changes in system states. Our highly flexible and efficient approach is applicable to any point data time series in earth and environmental sciences, regardless of their sub-discipline. Such advantage is particularly relevant when conducting simultaneous analysis of multiple processes and feedbacks, where a wide variety of data is used.</p><p>We illustrate our approach using the moth and butterfly data from the UK Environmental Change Network (ECN), where meteorological variables are used to define system states. A web application is publicly available to allow users to explore the method on various ECN site, while a generic is also available for users to upload their own data files. Our work contributes to the ongoing development of a better data science framework that allows researchers and other stakeholders to find and use the data they need more readily and reliably.</p><p> </p>
更多查看译文
AI 理解论文
溯源树
样例
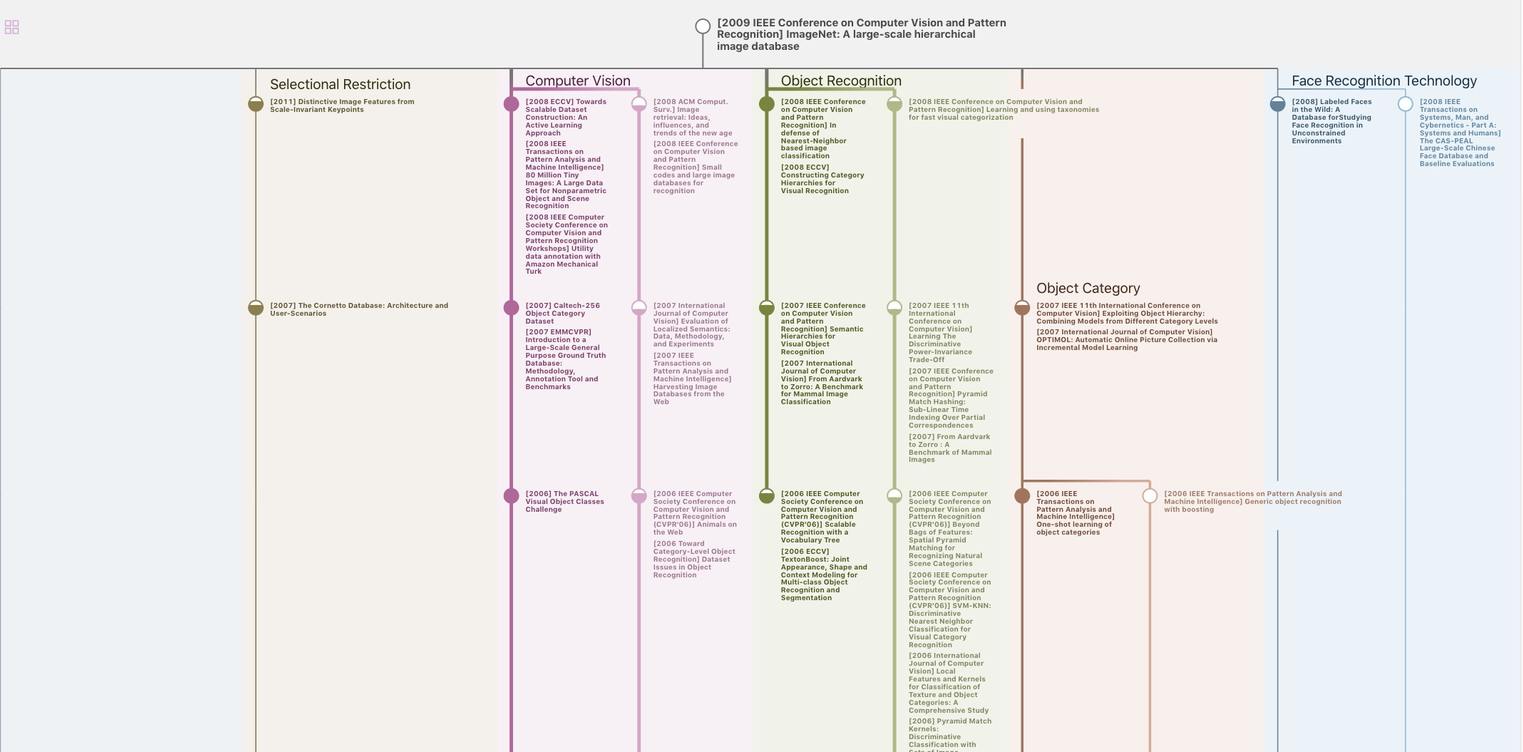
生成溯源树,研究论文发展脉络
Chat Paper
正在生成论文摘要