High-Dimensional Expected Shortfall Regression
arXiv (Cornell University)(2023)
摘要
The expected shortfall is defined as the average over the tail below (or above) a certain quantile of a probability distribution. The expected shortfall regression provides powerful tools for learning the relationship between a response variable and a set of covariates while exploring the heterogeneous effects of the covariates. In the health disparity research, for example, the lower/upper tail of the conditional distribution of a health-related outcome, given high-dimensional covariates, is often of importance. Under sparse models, we propose the lasso-penalized expected shortfall regression and establish non-asymptotic error bounds, depending explicitly on the sample size, dimension, and sparsity, for the proposed estimator. To perform statistical inference on a covariate of interest, we propose a debiased estimator and establish its asymptotic normality, from which asymptotically valid tests can be constructed. We illustrate the finite sample performance of the proposed method through numerical studies and a data application on health disparity.
更多查看译文
关键词
regression,high-dimensional
AI 理解论文
溯源树
样例
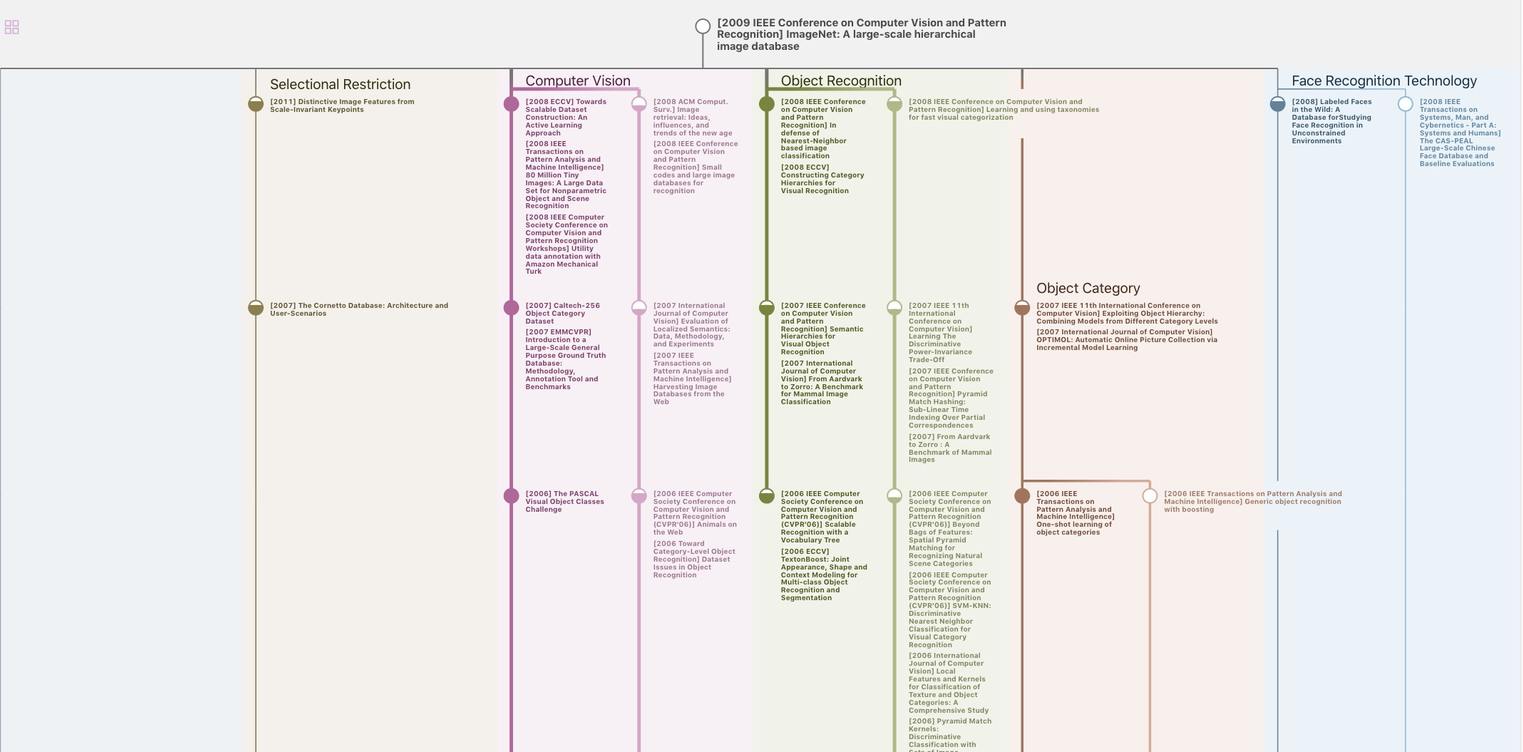
生成溯源树,研究论文发展脉络
Chat Paper
正在生成论文摘要