Contrastive Label Disambiguation for Self-Supervised Terrain Traversability Learning in Off-Road Environments
CoRR(2023)
摘要
Discriminating the traversability of terrains is a crucial task for autonomous driving in off-road environments. However, it is challenging due to the diverse, ambiguous, and platform-specific nature of off-road traversability. In this paper, we propose a novel self-supervised terrain traversability learning framework, utilizing a contrastive label disambiguation mechanism. Firstly, weakly labeled training samples with pseudo labels are automatically generated by projecting actual driving experiences onto the terrain models constructed in real time. Subsequently, a prototype-based contrastive representation learning method is designed to learn distinguishable embeddings, facilitating the self-supervised updating of those pseudo labels. As the iterative interaction between representation learning and pseudo label updating, the ambiguities in those pseudo labels are gradually eliminated, enabling the learning of platform-specific and task-specific traversability without any human-provided annotations. Experimental results on the RELLIS-3D dataset and our Gobi Desert driving dataset demonstrate the effectiveness of the proposed method.
更多查看译文
关键词
traversability learning,contrastive label disambiguation,self-supervised,off-road
AI 理解论文
溯源树
样例
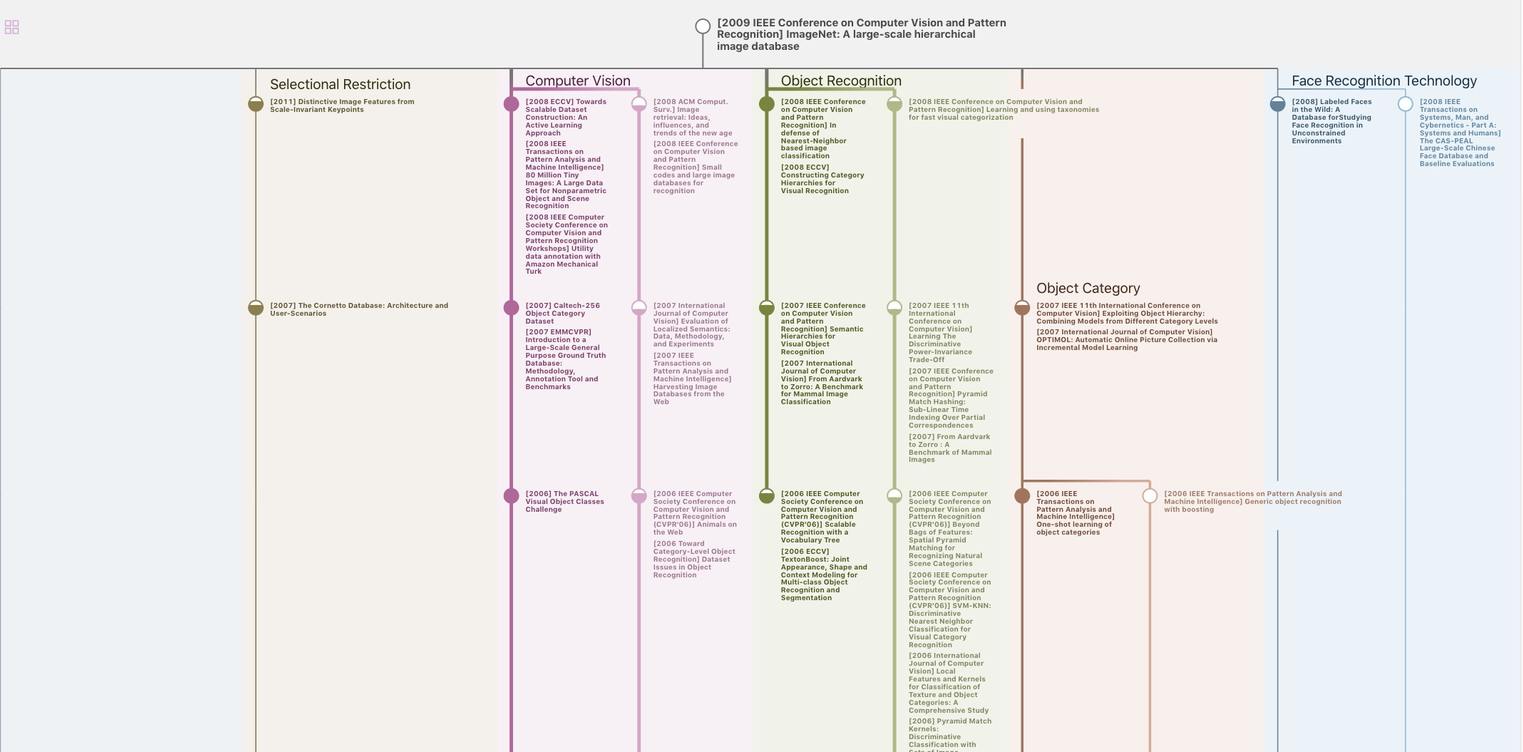
生成溯源树,研究论文发展脉络
Chat Paper
正在生成论文摘要