TGRL: An Algorithm for Teacher Guided Reinforcement Learning
ICML 2023(2023)
摘要
Learning from rewards (i.e., reinforcement learning or RL) and learning to imitate a teacher (i.e., teacher-student learning) are two established approaches for solving sequential decision-making problems. To combine the benefits of these different forms of learning, it is common to train a policy to maximize a combination of reinforcement and teacher-student learning objectives. However, without a principled method to balance these objectives, prior work used heuristics and problem-specific hyperparameter searches to balance the two objectives. We present a $\textit{principled}$ approach, along with an approximate implementation for $\textit{dynamically}$ and $\textit{automatically}$ balancing when to follow the teacher and when to use rewards. The main idea is to adjust the importance of teacher supervision by comparing the agent's performance to the counterfactual scenario of the agent learning without teacher supervision and only from rewards. If using teacher supervision improves performance, the importance of teacher supervision is increased and otherwise it is decreased. Our method, $\textit{Teacher Guided Reinforcement Learning}$ (TGRL), outperforms strong baselines across diverse domains without hyper-parameter tuning.
更多查看译文
关键词
reinforcement,tgrl,teacher,learning
AI 理解论文
溯源树
样例
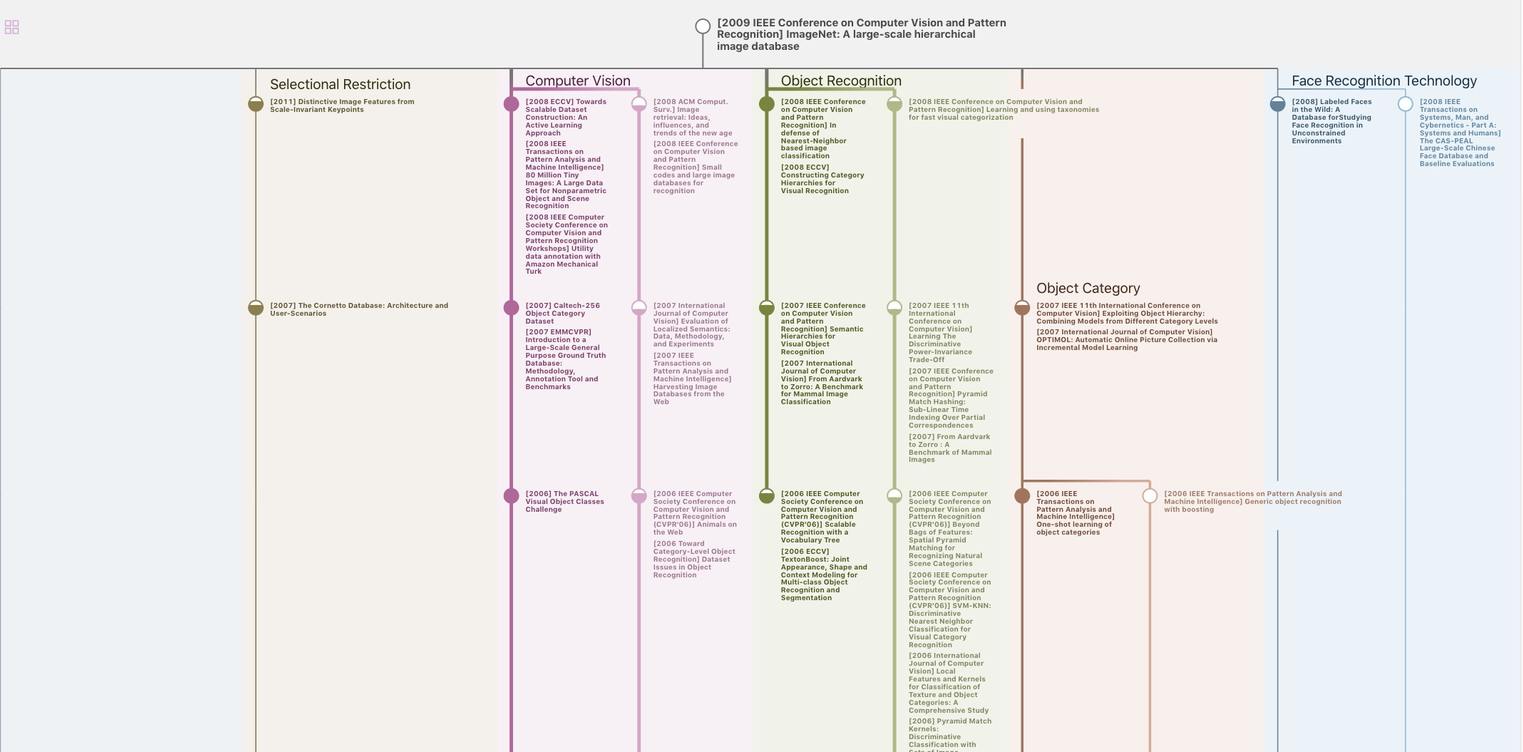
生成溯源树,研究论文发展脉络
Chat Paper
正在生成论文摘要