The Importance of Group Specification in Computational Modelling of Behaviour
crossref(2020)
摘要
**published as part of "Machine Learning for Health (ML4H) at NeurIPS 2020: https://arxiv.org/abs/2011.01725**Introduction: Hierarchical model fitting has become commonplace in cognitive neuroscience. However, these techniques require us to formalise assumptions about the data-generating process at the group level, which may not be known. In computational psychiatry, we frequently encounter situations with multiple distinct groups of subjects, which makes this specification non-trivial. Specifically, we must choose whether to assume all subjects are drawn from a common population, or to model them as deriving from separate populations. These assumptions have profound implications for computational psychiatry, as they will undoubtedly affect the resulting inference (parameter recovery) and conflate or mask true group-level differences. Methods: We used simulations to test these assumptions on synthetic multi-group behavioural data from commonly used multi-armed bandit tasks. We first demonstrate the influence of hierarchical vs non hierarchical approaches on parameter recovery. We then examine recovery of group differences in parameter values under different modelling approaches: (1) modelling groups under a common shared group-level prior (assuming all participants are generated from a common distribution, and are likely to share common characteristics); (2) modelling separate groups based on symptomatology or diagnostic labels, resulting in separate group-level priors. We evaluate the robustness of these approaches to variations in data quality and in prior specification.Results: We confirm, firstly, that hierarchical approaches lead to better parameter recovery. Secondly, we argue that fitting groups separately (Model 2) provides the most robust inference across all conditions and perturbations. Thirdly, we confirm these assumptions have less of an impact with higher numbers of trials and subjects. Finally, we show that the advice to fit groups separately can fall down when extreme assumptions are made about group variance.Conclusions: Our results suggest that when dealing with data from multiple clinical groups, researchers should use a hierarchical approach to parameter fitting, but account for hypothesised group differences in the model fitting procedure. In other words, under most normal circumstances, researchers should fit patient and control groups separately.
更多查看译文
AI 理解论文
溯源树
样例
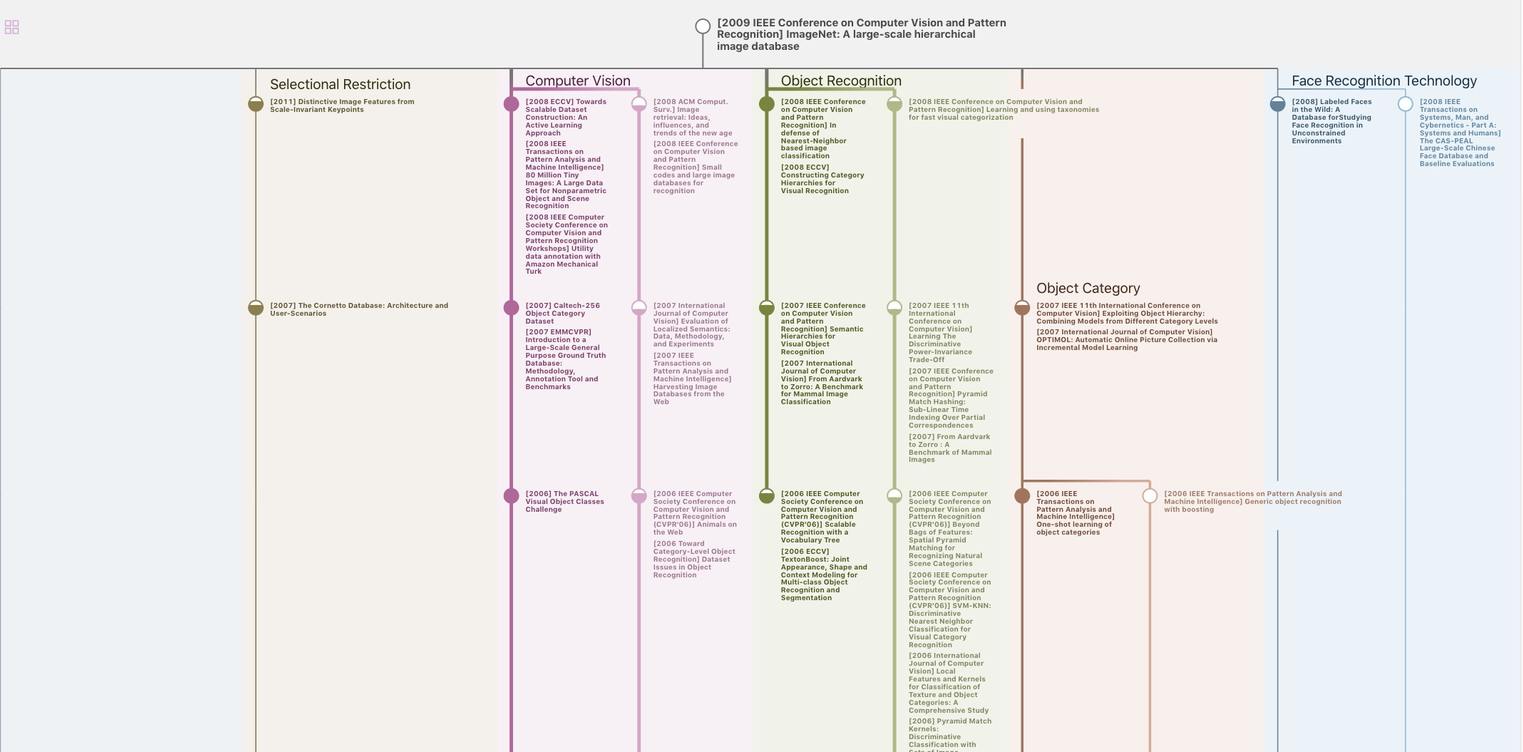
生成溯源树,研究论文发展脉络
Chat Paper
正在生成论文摘要