Human Performance Consequences of Normative and Contrastive Explanations: an Experiment in Machine Learning for Reliability Maintenance.
ARTIFICIAL INTELLIGENCE(2023)
关键词
Human-AI interaction,Explainable AI,Automation reliance behavior,Automation transparency
AI 理解论文
溯源树
样例
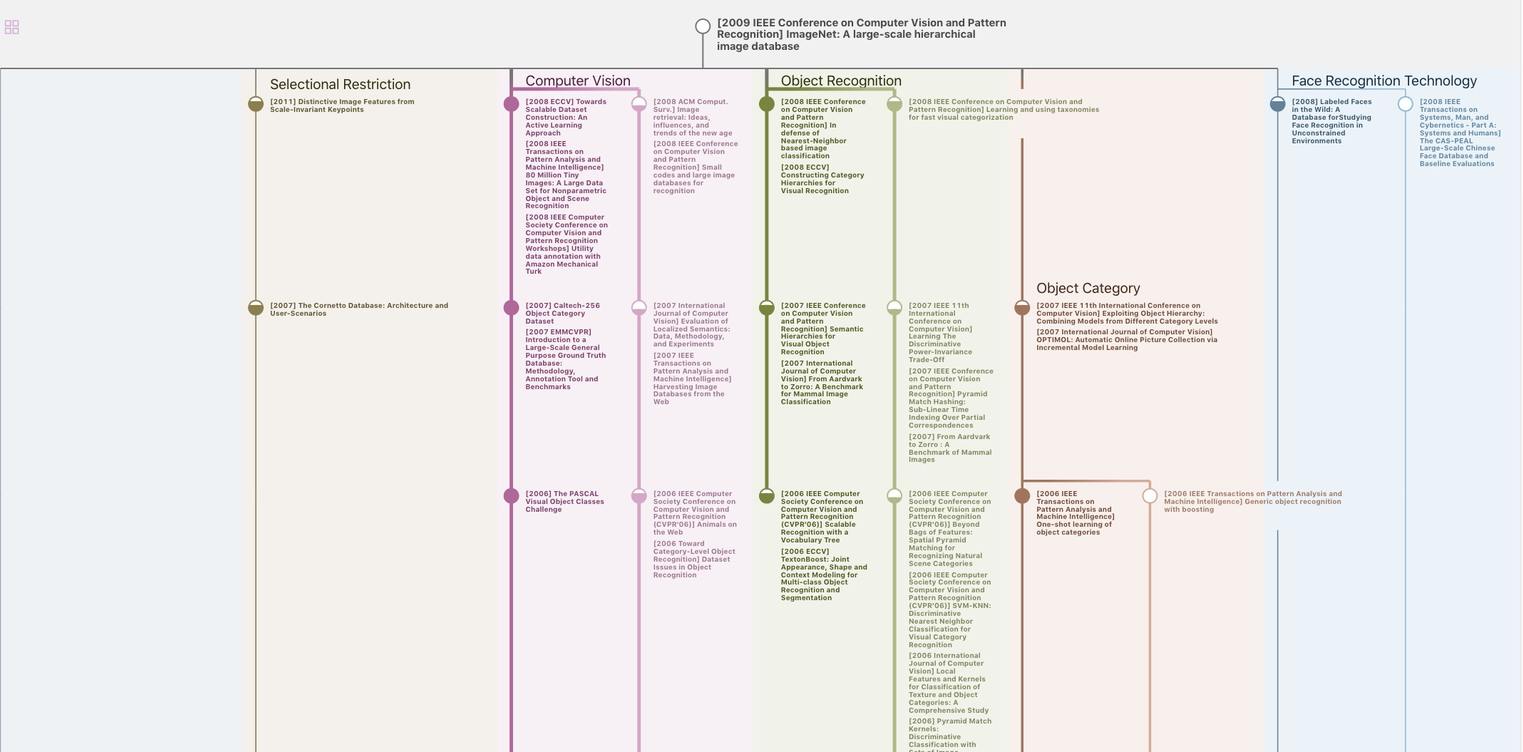
生成溯源树,研究论文发展脉络
Chat Paper
正在生成论文摘要