Hepatocellular carcinoma histopathological images grading with a novel attention-sharing hybrid network based on multi-feature fusion
Biomedical Signal Processing and Control(2023)
摘要
Throughout history until today, hepatocellular carcinoma (HCC) remains one of the most serious illnesses worldwide due to its high mortality rates. One of the most essential steps to diagnose HCC and its differentiated degree is histopathological image analysis, which is implemented by experienced pathologists. However, this method is time-consuming and difficult for young pathologists to implement. Nowadays, few researchers have proposed approaches based on computer-aided systems to automatically grade HCC due to the complexity of hepatocellular carcinoma histopathological images. Therefore, this paper proposes a novel dual channel attention-sharing hybrid network (DCAH-Net) based on multi-feature fusion to automatically grade HCC histopathological images to assist pathologists in diagnosing HCC. To solve the problem that large scale histopathological images cannot be utilized to train networks directly, patch-based method is utilized in this work to divide large scale image into patches. To effectively extract local and global features, convolutional neural networks and transformers are deployed in the DCAH-Net. A novel attention-sharing mechanism containing a simplified outlook attention and a feature projection block is proposed in DCAH-Net to provide detailed information for both channels. The performance of DCAH-Net compared with existing methods achieves the highest accuracy, macro recall, macro precision and macro F1-score of 88.36%, 82.14%, 83.00% and 82.30% on HCC histopathological dataset. In addition, LC25000 and BreakHis dataset are utilized to validate the generalization ability of DCAH-Net. The results obtained show that DCAH-Net has great potential in other medical classification tasks.
更多查看译文
关键词
hepatocellular carcinoma,histopathological images,fusion,attention-sharing,multi-feature
AI 理解论文
溯源树
样例
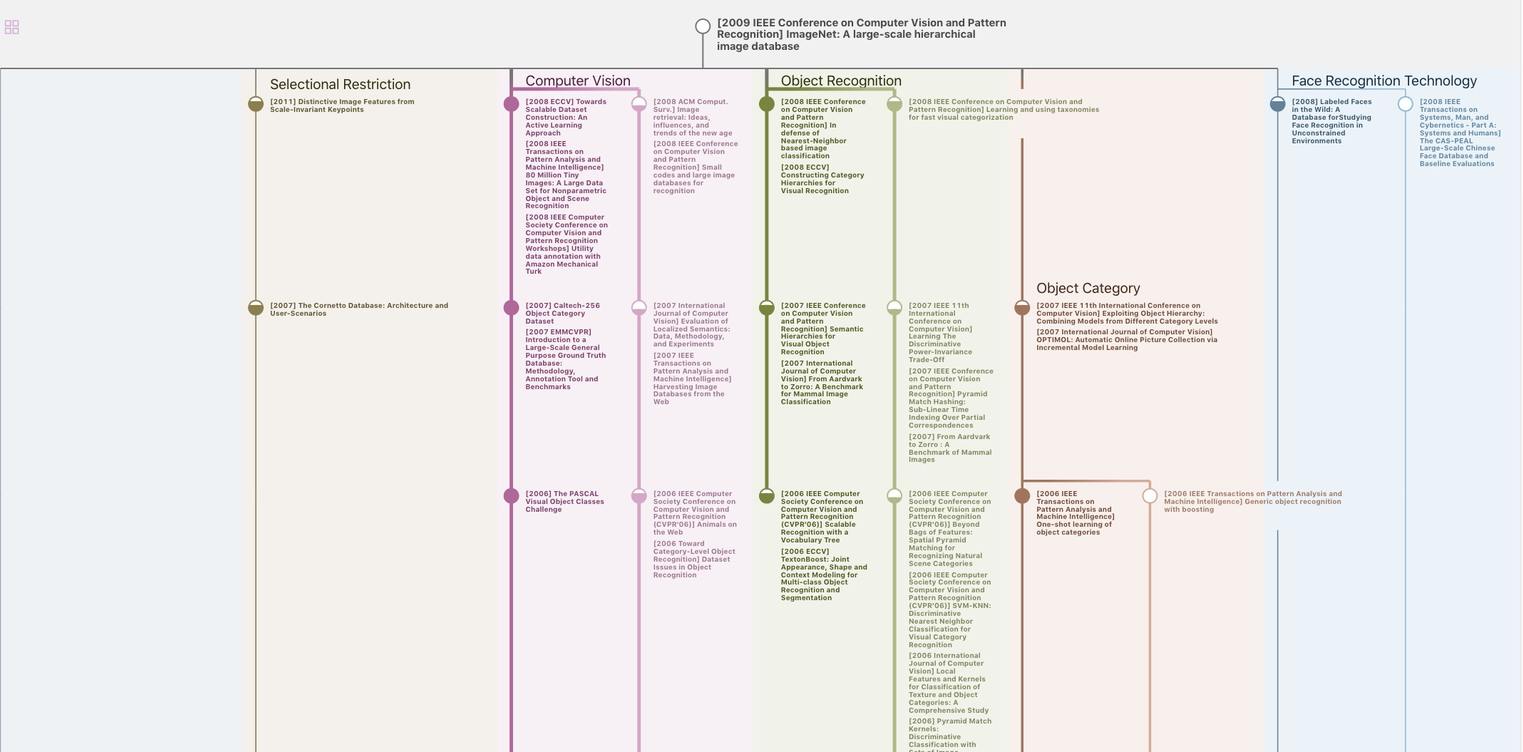
生成溯源树,研究论文发展脉络
Chat Paper
正在生成论文摘要