Risk Factors of Hyperglycemia After Treatment With the AKT Inhibitor Ipatasertib in the Prostate Cancer Setting: A Machine Learning-Based Investigation
JCO CLINICAL CANCER INFORMATICS(2023)
摘要
PURPOSE Hyperglycemia is a major adverse event of phosphatidylinositol 3-kinase/AKT inhibitor class of cancer therapeutics. Machine learning (ML) methodologies can identify and highlight how explanatory variables affect hyperglycemia risk. METHODS Using data from clinical trials of the AKT inhibitor ipatasertib (IPAT) in the metastatic castrate-resistant prostate cancer setting, we trained an XGBoost ML model to predict the incidence of grade >= 2 hyperglycemia (HGLY >= 2). Of the 1,364 patients included in our analysis, 19.4% (n = 265) of patients had HGLY >= 2 events with a median time of first onset of 28 days (range, 0-753 days), and 30.0% (n = 221) of patients on an IPAT regimen had at least one HGLY >= 2 event compared with 7.0% (n = 44) of patients on placebo. RESULTS An 11-variable XGBoost model predicted HGLY >= 2 events well with an AUROC of 0.83 +/- 0.02 (mean +/- standard deviation). Using SHapley Additive exPlanations analysis, we found IPAT exposure and baseline HbA1c levels to be the strongest predictors of HGLY >= 2, with additional predictivity of baseline measurements of fasting glucose, magnesium, and high-density lipoproteins. CONCLUSION The findings support using patients' prediabetic status as a key factor for hyperglycemia monitoring and/or trial exclusion criteria. Additionally, the model and relationships between explanatory variables and HGLY >= 2 described herein can help identify patients at high risk for hyperglycemia and develop rational risk mitigation strategies.
更多查看译文
AI 理解论文
溯源树
样例
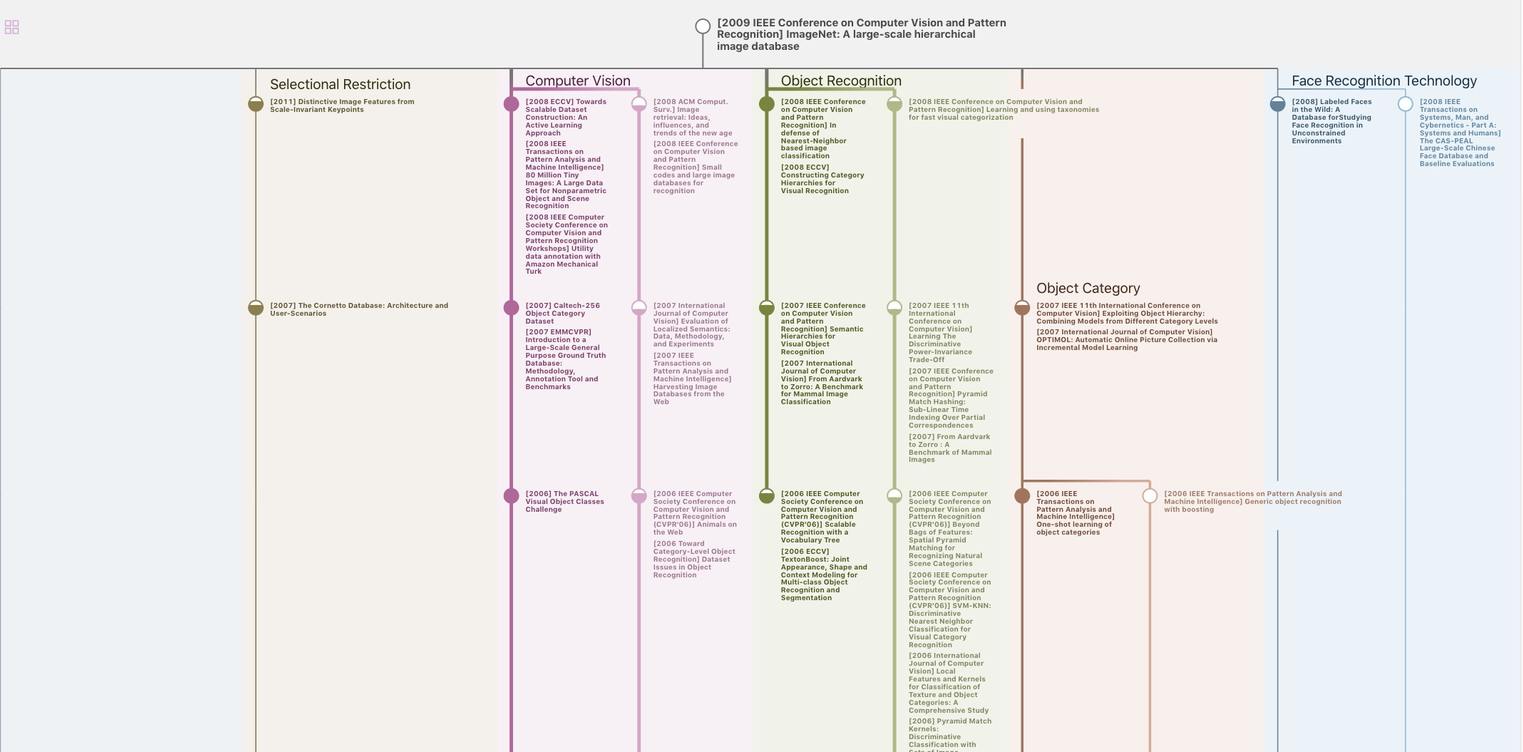
生成溯源树,研究论文发展脉络
Chat Paper
正在生成论文摘要