Predicting the Effect of Voids Generated During RTM on the Low-Velocity Impact Behaviour by Machine Learning-Based Surrogate Models
Composites Part B, Engineering(2023)
摘要
The main objective of the present paper is to demonstrate the feasibility of machine-learning-based surrogate models for predicting low-velocity impact behaviour considering void content and location generated during the resin transfer moulding process. Generating reliable experimental datasets for training those models is almost impossible, therefore an adapted finite element model was implemented providing reliable results to generate the synthetic datasets. The optimum hyperparameter combination for training the Random Forest model was found based on the grid search technique. The accuracy of the classification, single-output regression and multi-output regression models was sufficient. It was concluded that the multi-output regression model, which predicts the force-time, displacement-time, and energy-time curves, provides the best information, and is sufficiently accurate (R2 > 0.995) and fast (5 s per sample) as an online structural performance monitoring tool.
更多查看译文
关键词
Polymer-matrix composites (PMCs),Impact behaviour,Surrogate model,Resin transfer moulding (RTM)
AI 理解论文
溯源树
样例
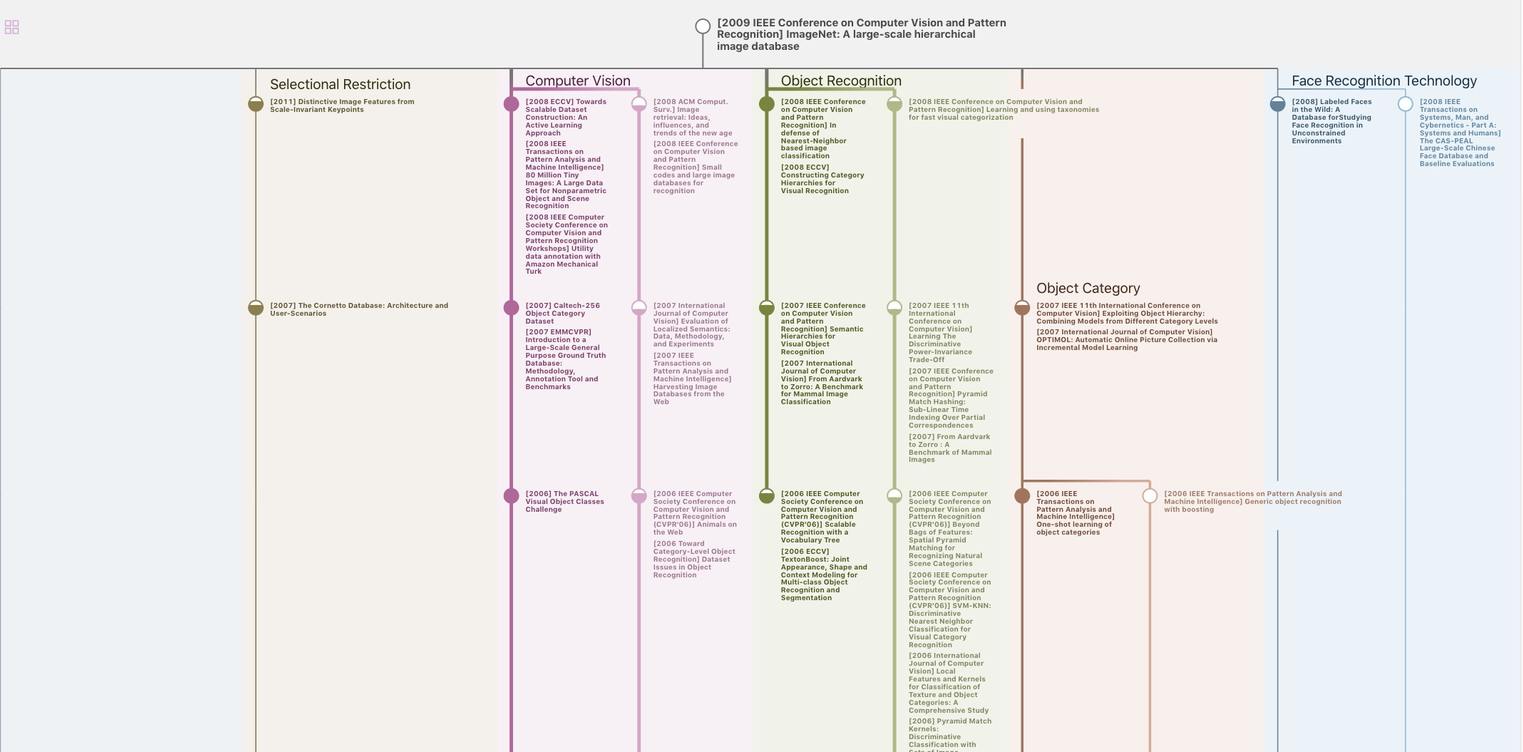
生成溯源树,研究论文发展脉络
Chat Paper
正在生成论文摘要