Unsupervised real image super-resolution via knowledge distillation network
Computer Vision and Image Understanding(2023)
摘要
Super-resolution convolutional neural networks recently have demonstrated high-quality restoration for single images. Despite existing methods have achieved remarkable performance based on synthetic datasets, the performance is poor on real-world or natural data. To address this issue, zero-shot super-resolution (ZSSR) has been proposed for adaptive learning. However, ZSSR is unable to keep the simulated degradation process consistent with the degradation kernel of the real degradation process. Furthermore, the learned mapping of ZSSR is different from the desired mapping. In this paper, an unsupervised image super-resolution via knowledge distillation network (USRKDN) is proposed. Specifically, the proposed degradation module generates an image-specific degradation kernel and corresponding degenerated images. Moreover, the knowledge distillation module is proposed to solve the issue that the mapping cannot be completely equivalent, which transfers the learned map by knowledge distillation. The full convolution module is also explored to help the reconstruction of information. Extensive experimental results on synthetic and real datasets demonstrate the effectiveness of USRKDN. In addition, USRKDN is proven to be good at reconstructing image details in real scenes, which provides an effective method for generating information learning tasks with fewer samples.
更多查看译文
关键词
Super-resolution, Knowledge distillation, Degradation module, Convolutional neural network
AI 理解论文
溯源树
样例
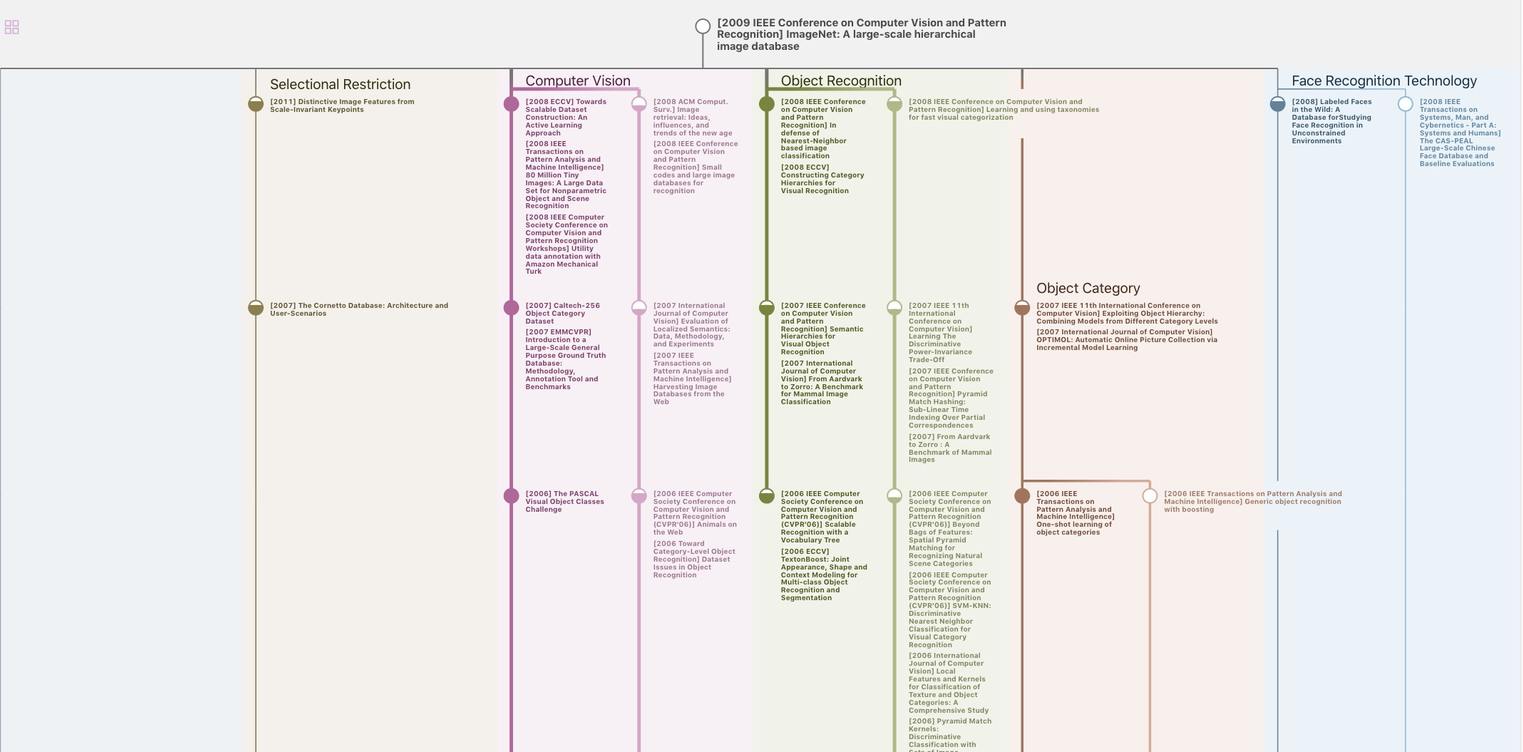
生成溯源树,研究论文发展脉络
Chat Paper
正在生成论文摘要