Cross-domain few-shot action recognition with unlabeled videos
Computer Vision and Image Understanding(2023)
摘要
Current few-shot action recognition approaches have achieved impressive performance using only a few labeled examples. However, they usually assume the base (train) and target (test) videos typically come from the same domain, which may limit their further applications. In this paper, we introduce a new practical task, termed as cross-domain few-shot action recognition, and hypothesize there is a domain shift between the base and target videos and the unlabeled target videos are available. To address this task, we further propose a Self-supervised learning Enhanced tEmporal Network (SEEN), which incorporates temporal modeling and self -supervised learning techniques to learn more transferable representations. Concretely, the temporal modeling mechanism aims to learn long-range temporal semantics from the features output by the backbone, and the self-supervised learning focuses on exploring the underlying data patterns to reduce domain shifts under the few-shot setting, which can help to improve the generalization ability. Therefore, the proposed SEEN can capture broader variations of the feature distributions and is more appropriate for the cross-domain few-shot action recognition task. Extensive experiments on multiple cross-domain benchmarks show that our SEEN consistently outperforms several strong baseline methods by a convincing margin.
更多查看译文
关键词
Few-shot action recognition,Cross-domain,Self-supervised learning,Temporal modeling
AI 理解论文
溯源树
样例
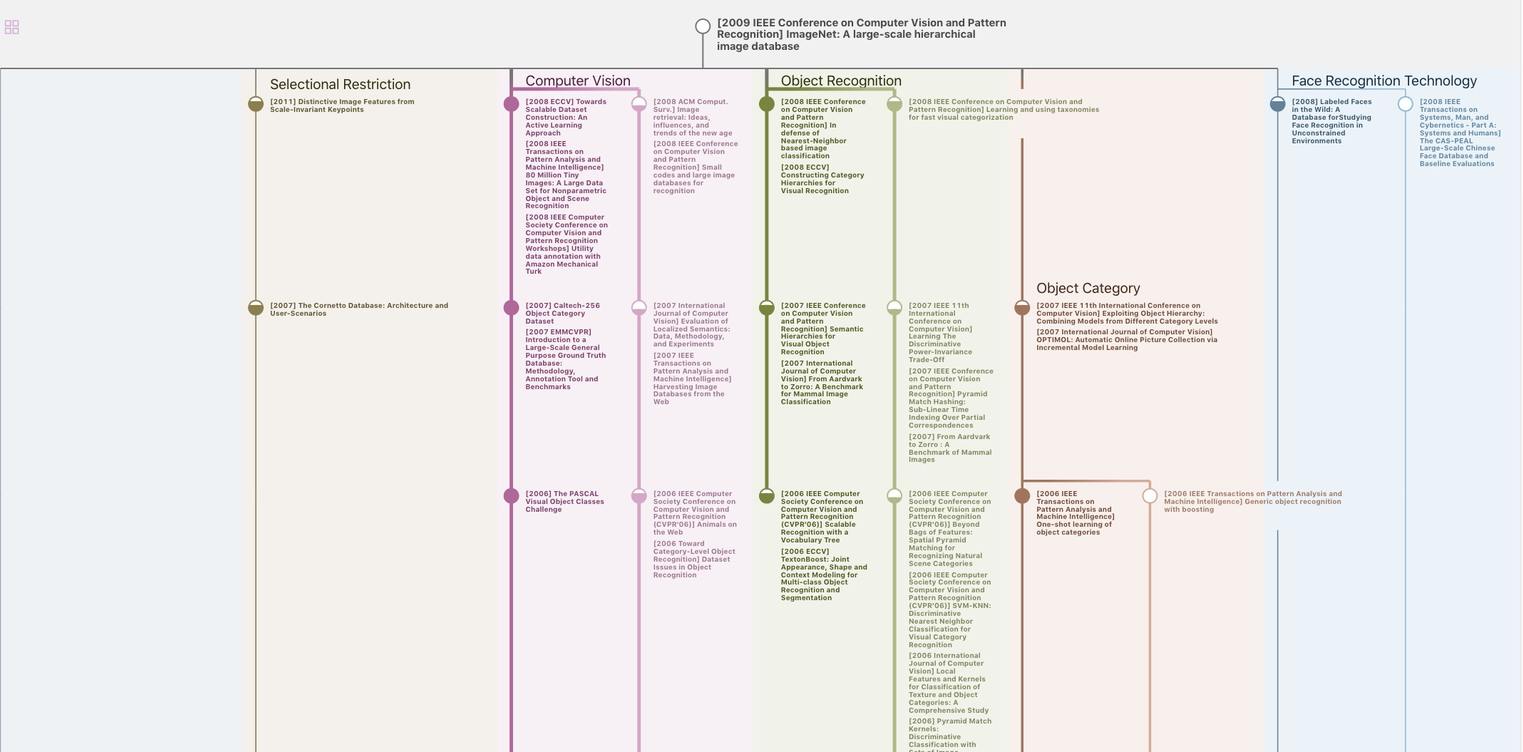
生成溯源树,研究论文发展脉络
Chat Paper
正在生成论文摘要