Unsupervised Domain Adaptation for Semantic Segmentation Via Cross-Region Alignment
Computer vision and image understanding(2023)
摘要
Semantic segmentation requires a lot of training data, which necessitates costly annotation. There have been many studies on unsupervised domain adaptation (UDA) from one domain to another, e.g., from computer graphics to real images. However, there is still a gap in accuracy between UDA and supervised training on native domain data. It is arguably attributable to the class-level misalignment between the source and target domain data. To cope with this, we propose a method that applies adversarial training to align two feature distributions in the target domain. It uses a self-training framework to split the image into two regions (i.e., trusted and untrusted), which form two distributions to align in the feature space. We term this approach cross-region adaptation (CRA) to distinguish it from the previous methods of aligning different domain distributions, which we call cross-domain adaptation (CDA). CRA can be applied after any CDA method. Experimental results show that this always improves the accuracy of the combined CDA method.
更多查看译文
关键词
Domain adaptation,Semantic segmentation,CNN
AI 理解论文
溯源树
样例
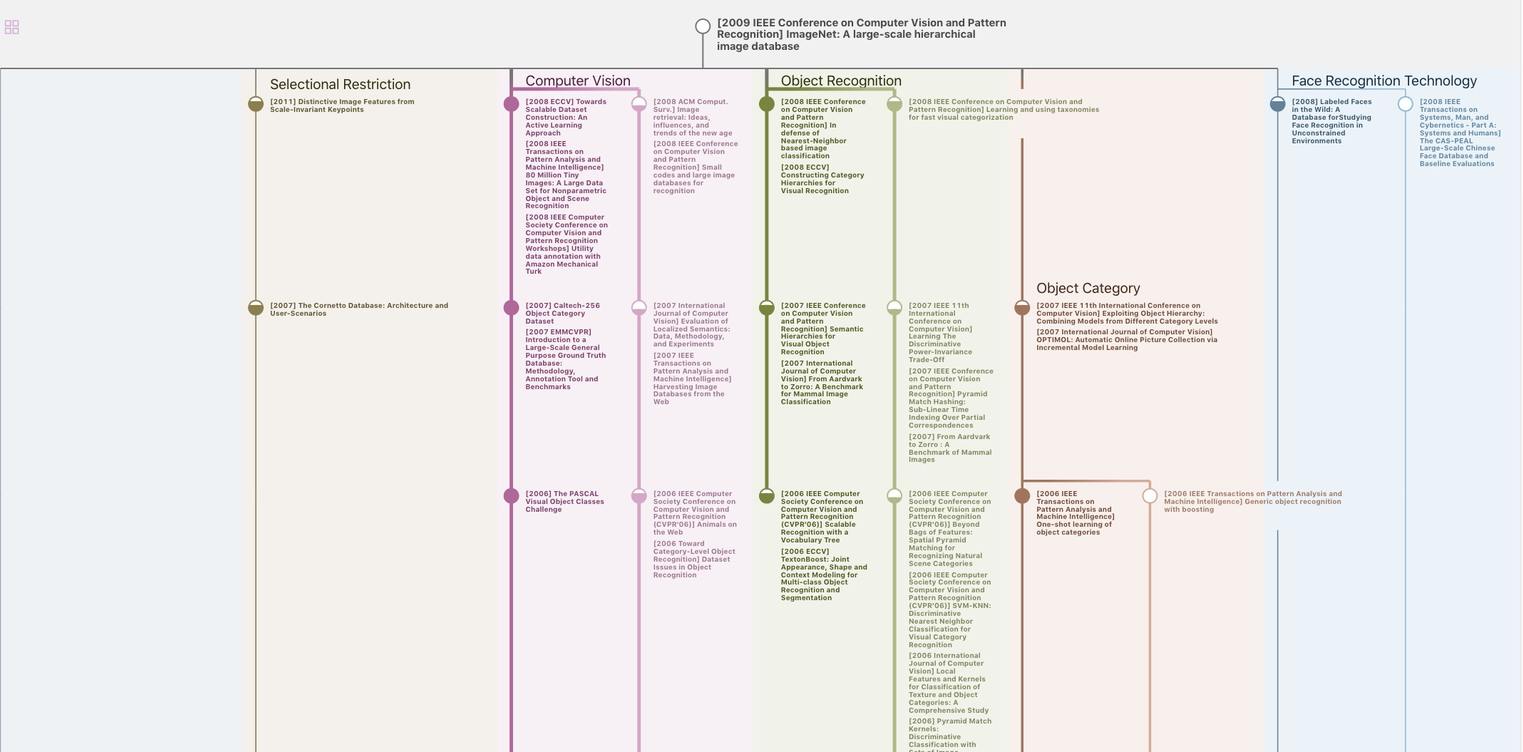
生成溯源树,研究论文发展脉络
Chat Paper
正在生成论文摘要