Human skeletons and change detection for efficient violence detection in surveillance videos
Computer Vision and Image Understanding(2023)
摘要
In our constantly monitored world, surveillance cameras play a crucial role in curbing crime and violence in public spaces by serving as a deterrent. To enhance their effectiveness, there is a growing need for automated tools that can detect crimes in real time. In this paper, we propose a novel deep learning architecture that accurately and efficiently detects violent crimes in surveillance videos. We rely on what we believe are the most essential pieces of information to detect violence, namely: human bodies and their interaction. To this end, we employ human pose extractors and change detectors as the input of our proposal. Subsequently, we combine them using a novel method, which relies on additions instead of multiplications to guarantee the transmission of information even when one of the inputs provides a zero-valued signal; outperforming other combination alternatives of the literature. Finally, to account for both spatial and temporal information, we use a convolutional alternative of the standard LSTM, the ConvLSTM. The experiments performed on several benchmark datasets demonstrate the efficacy and efficiency of our proposal, achieving state-of-the-art results with much fewer trainable parameters. We release the code to replicate the proposed architecture at https://github.com/atmguille/Violence-Detection-With-Human-Skeletons.
更多查看译文
关键词
Machine vision and scene understanding, Violence detection, Pose estimation, Deep Learning, ConvLSTM
AI 理解论文
溯源树
样例
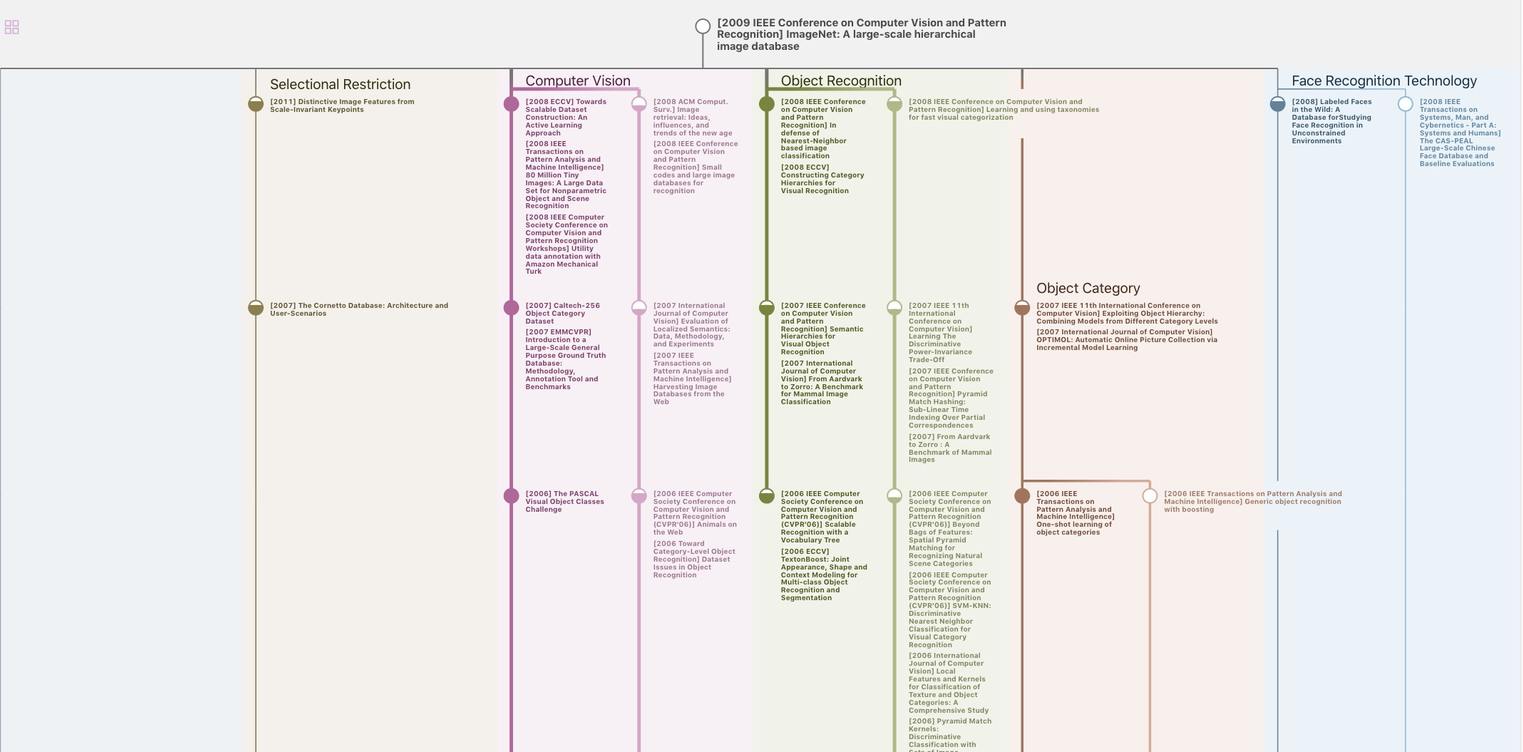
生成溯源树,研究论文发展脉络
Chat Paper
正在生成论文摘要