A data-efficient zero-shot and few-shot Siamese approach for automated diagnosis of left ventricular hypertrophy
Computers in Biology and Medicine(2023)
摘要
Left ventricular hypertrophy (LVH) is a life-threatening condition in which the muscle of the left ventricle thickens and enlarges. Echocardiography is a test performed by cardiologists and echocardiographers to diagnose this condition. The manual interpretation of echocardiography tests is time-consuming and prone to errors. To address this issue, we have developed an automated LVH diagnosis technique using deep learning. However, the availability of medical data is a significant challenge due to varying industry standards, privacy laws, and legal constraints. To overcome this challenge, we have proposed a data-efficient technique for automated LVH classification using echocardiography. Firstly, we collected our own dataset of normal and LVH echocardiograms from 70 patients in collaboration with a clinical facility. Secondly, we introduced novel zero-shot and few-shot algorithms based on a modified Siamese network to classify LVH and normal images. Unlike traditional zero-shot learning approaches, our proposed method does not require text vectors, and classification is based on a cutoff distance. Our model demonstrates superior performance compared to stateof-the-art techniques, achieving up to 8% precision improvement for zero-shot learning and up to 11% precision improvement for few-shot learning approaches. Additionally, we assessed the inter-observer and intra-observer reliability scores of our proposed approach against two expert echocardiographers. The results revealed that our approach achieved better inter-observer and intra-observer reliability scores compared to the experts.
更多查看译文
关键词
Echocardiography,Siamese network,Left ventricular hypertrophy
AI 理解论文
溯源树
样例
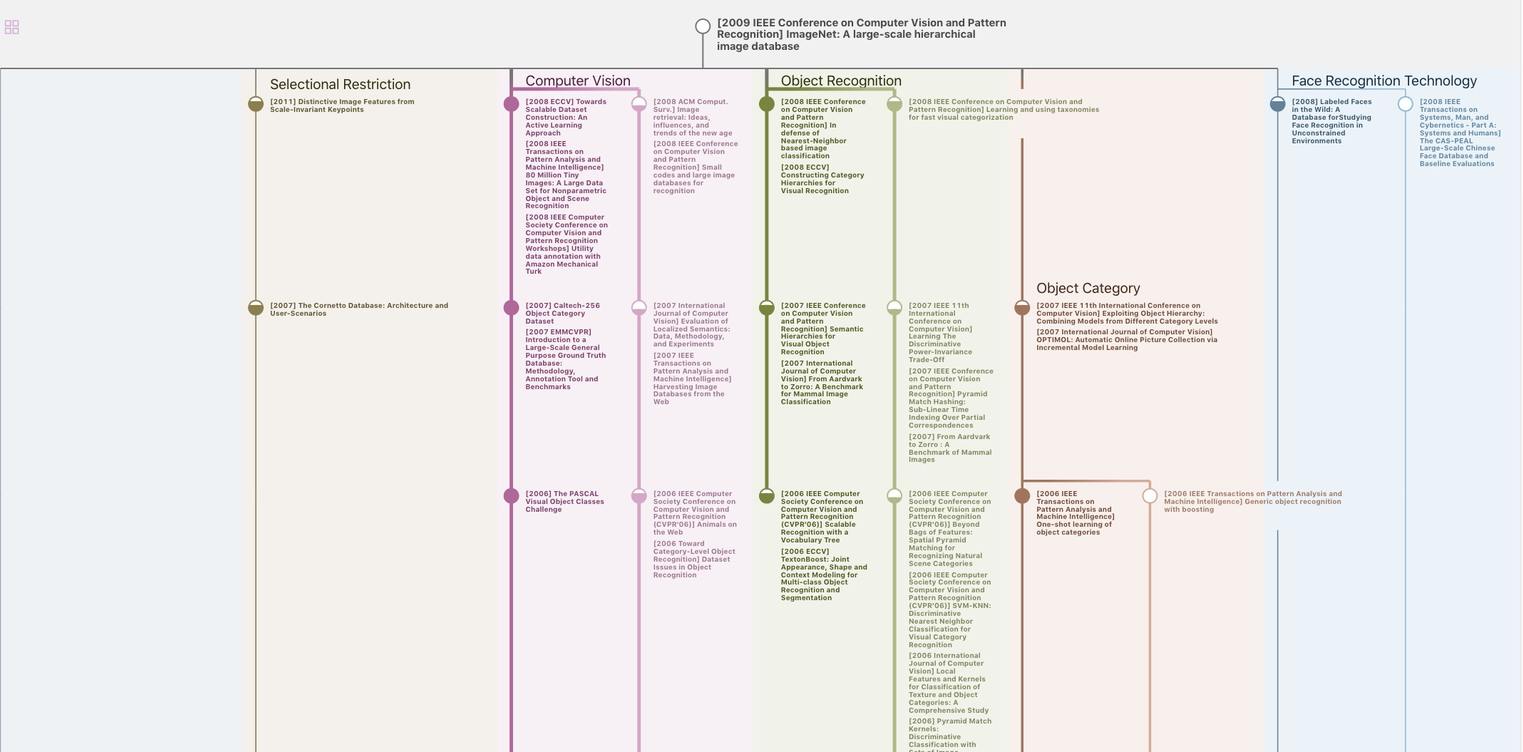
生成溯源树,研究论文发展脉络
Chat Paper
正在生成论文摘要