Bayesian parameter estimation for the inclusion of uncertainty in progressive damage simulation of composites
Composite Structures(2023)
摘要
Despite gradual progress over the past decades, the simulation of progressive damage in composite laminates remains a challenging task, in part due to inherent uncertainties of material properties. This paper combines three computational methods - finite element analysis (FEA), machine learning and Markov Chain Monte Carlo - to estimate the probability density of FEA input parameters while accounting for the variation of mechanical properties. First, 15,000 FEA simulations of open-hole tension tests are carried out with randomly varying input parameters by applying continuum damage mechanics material models. This synthetically generated data is then used to train and validate a neural network consisting of five hidden layers and 32 nodes per layer to develop a highly efficient surrogate model. With this surrogate model and the incorporation of statistical test data from experiments, the application of Markov Chain Monte Carlo algorithms enables Bayesian parameter estimation to learn the probability density of input parameters for the simulation of progressive damage evolution in fibre reinforced composites. This methodology is validated against various open-hole tension test geometries enabling the determination of virtual design allowables.
更多查看译文
关键词
Finite element analysis,Machine learning,Markov chain Monte Carlo,Open-hole tension
AI 理解论文
溯源树
样例
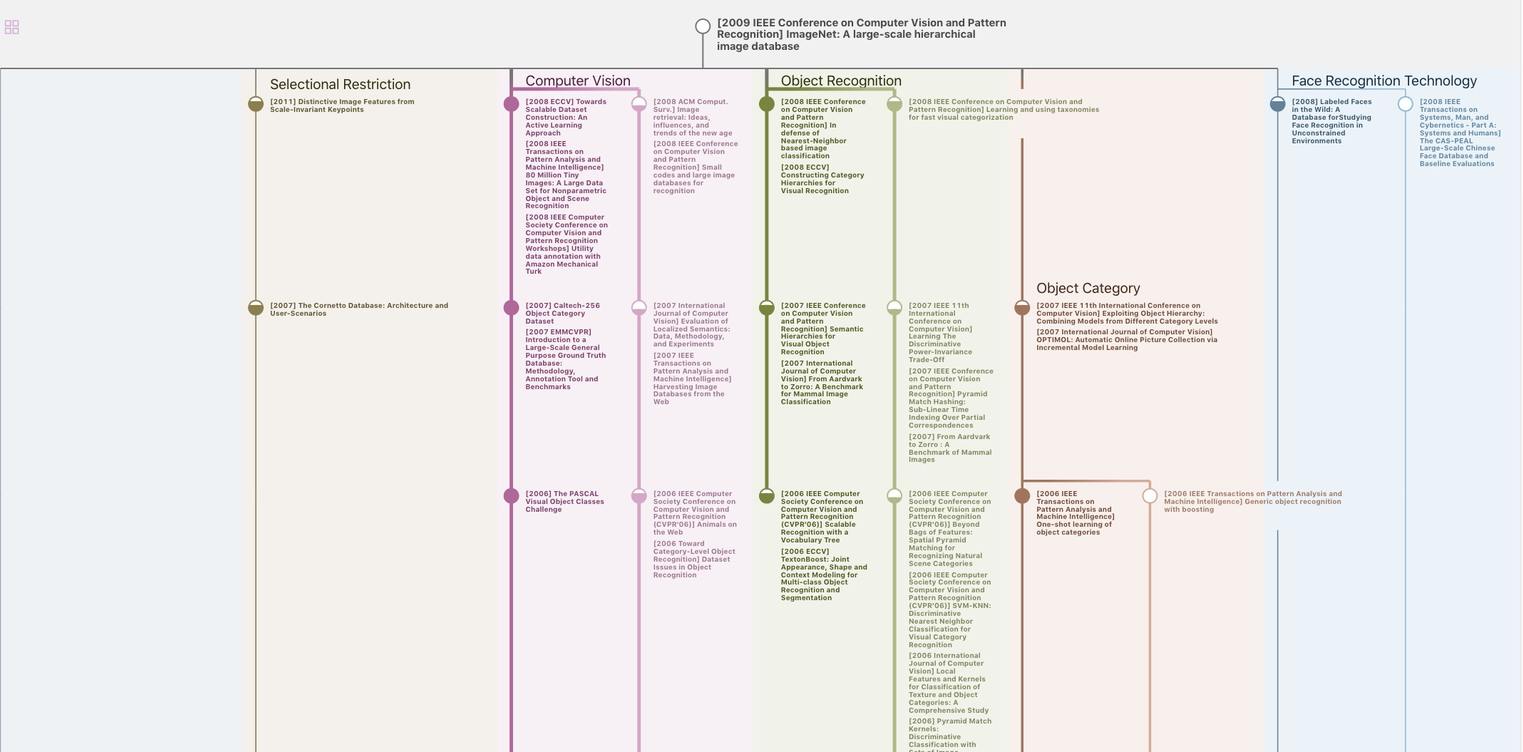
生成溯源树,研究论文发展脉络
Chat Paper
正在生成论文摘要