Data-driven modeling-based digital twin of supercritical coal-fired boiler for metal temperature anomaly detection
Energy(2023)
摘要
The flexible operation of coal-fired boilers deteriorates the heat transfer capacity of tubes, leading to localized overheating, tube cracking, and even boiler failure. This study presents a digital twin (DT) for detecting the metal temperature anomaly of a coal-fired boiler by predicting the metal temperature time series using data-driven methods. A data-based model adopting an extreme gradient boosting algorithm tuned by a particle swarm optimization algorithm is proposed to predict the metal temperature with an output sequence of 6 min. The predicted metal temperature is then transformed into an interval based on statistical distribution and confidence level to improve the robustness of the model. Moreover, a histogram is used to determine the benchmark of metal temperature anomaly with respect to varying operating conditions. The proposed DT is applied to a 600-MW on-duty supercritical coal-fired boiler. Results indicate that the proposed DT can accurately predict metal temperature and is useful for detecting metal temperature anomalies in advance.
更多查看译文
关键词
Coal-fired boiler, Metal temperatures prediction, Data driven, Flexible operation
AI 理解论文
溯源树
样例
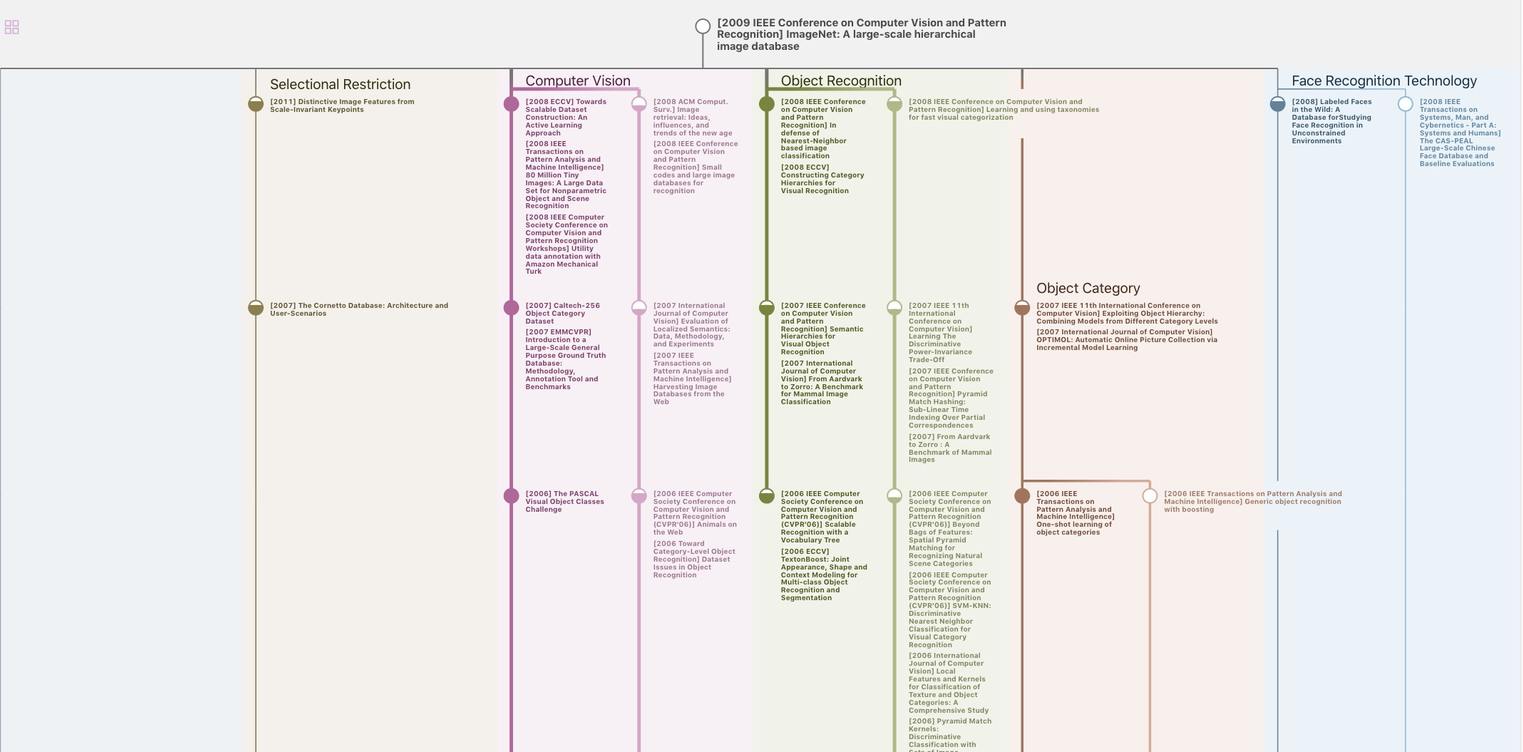
生成溯源树,研究论文发展脉络
Chat Paper
正在生成论文摘要