A machine learning approach for time series forecasting with application to debt risk of the Montenegrin electricity industry
Energy Reports(2023)
摘要
Level of customer electricity debts is a relevant information for the electricity production company, as it represents uncollected revenue for the provided service. Higher level of debts may affect the provider’s financial stability and the ability to invest and maintain their network. On the other hand, high levels of debt may indicate larger macroeconomic problems, such as the lower standard of the citizens or high unemployment. In this paper, a Machine Learning approach for electricity debt prediction was applied, using Support Vector Regression method and data from the Montenegrin electricity provider. The obtained results indicate an excellent model performance, proving that the chosen method is an outstanding choice for this task, compared to other machine learning methods. The forecast of electricity user debts using machine learning techniques adds a new research area to the existing research, as the previous literature mostly concentrated on the prediction of electric load, consumption, and demand. The risk of default may be larger in lower income nations, as is the case in this research, therefore risk prediction and mitigation are crucial for the power supplier in these countries. The results obtained in this research on the test set are 1.63% and 0.854, for Relative Error and R2, respectively, showing excellent predictive performance. Additionally, correlation coefficient is close to 1 in cross-validation and on unknown data. Thus, it can be confirmed that the debt prediction was efficient.
更多查看译文
关键词
Electricity debt prediction,Support vector regression,Time series forecasting,Montenegro
AI 理解论文
溯源树
样例
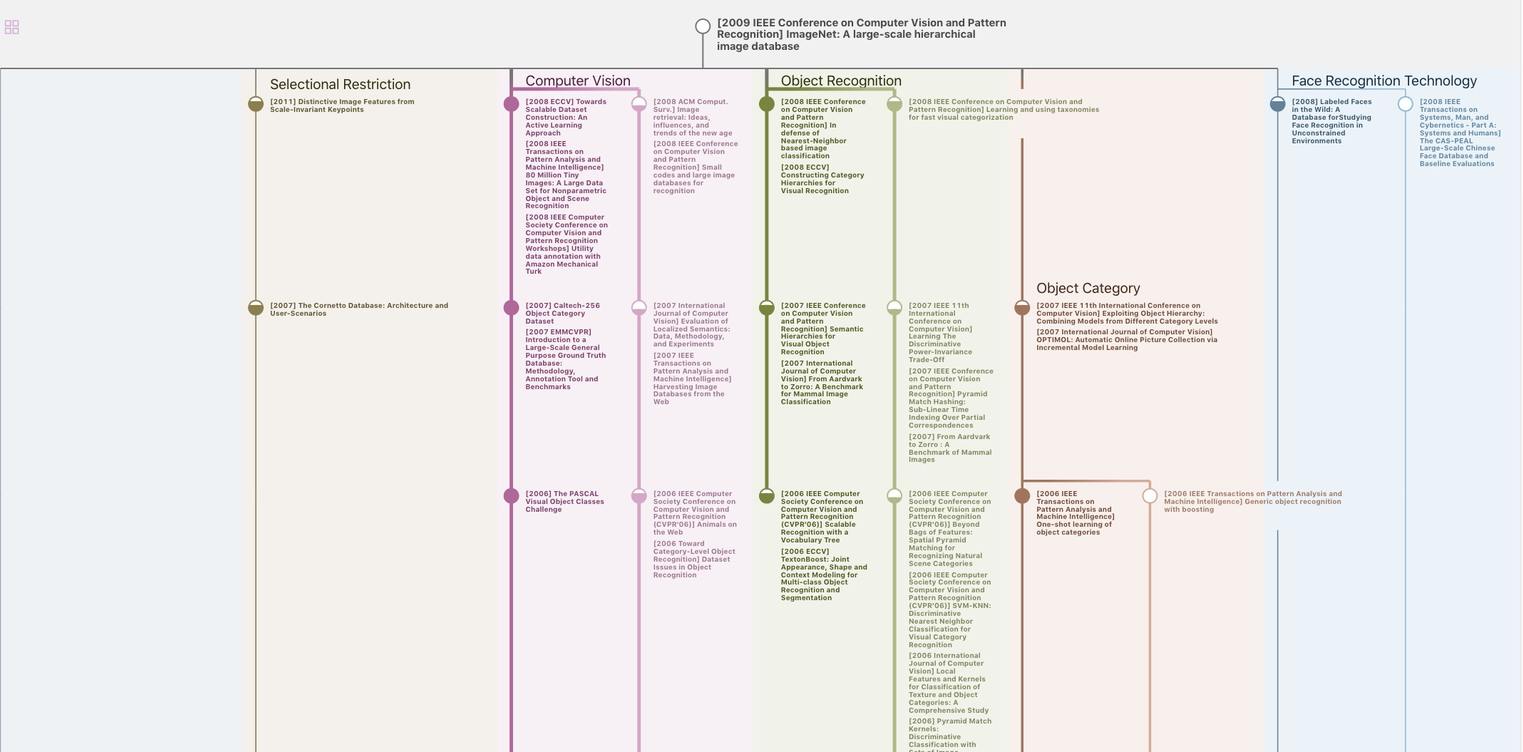
生成溯源树,研究论文发展脉络
Chat Paper
正在生成论文摘要