Extended natural neighborhood for SMOTE and its variants in imbalanced classification
Engineering Applications of Artificial Intelligence(2023)
Abstract
Imbalanced data classification is a challenging issue encountered in many practical applications. Synthetic minority oversampling technique (SMOTE) and its variants are popular resampling methods. However, in most of these methods, the neighborhood determined by k-nearest neighbor (kNN) cannot reflect the local distribution precisely, leading to the generation of noisy examples. To solve this problem, we propose a neighborhood concept without parameter k called extended natural neighbor (ENaN), which is derived from natural neighbor (NaN). ENaN unites kNN and reverse kNN to determine neighbors adaptively according to the sample distribution. Compared to NaN, ENaN explores broad neighborhoods, which facilitates to improve the quality of generated examples. ENaN-based SMOTE (ENaNSMOTE) can improve the sample distribution obtained by SMOTE and NaNSMOTE. Extensive experiments using 30 synthetic and 20 real-world datasets prove the effectiveness of ENaN in SMOTE and its variants.
MoreTranslated text
Key words
Imbalanced classification,SMOTE,Extended natural neighbor,k-nearest neighbor,Reverse k-nearest neighbor
AI Read Science
Must-Reading Tree
Example
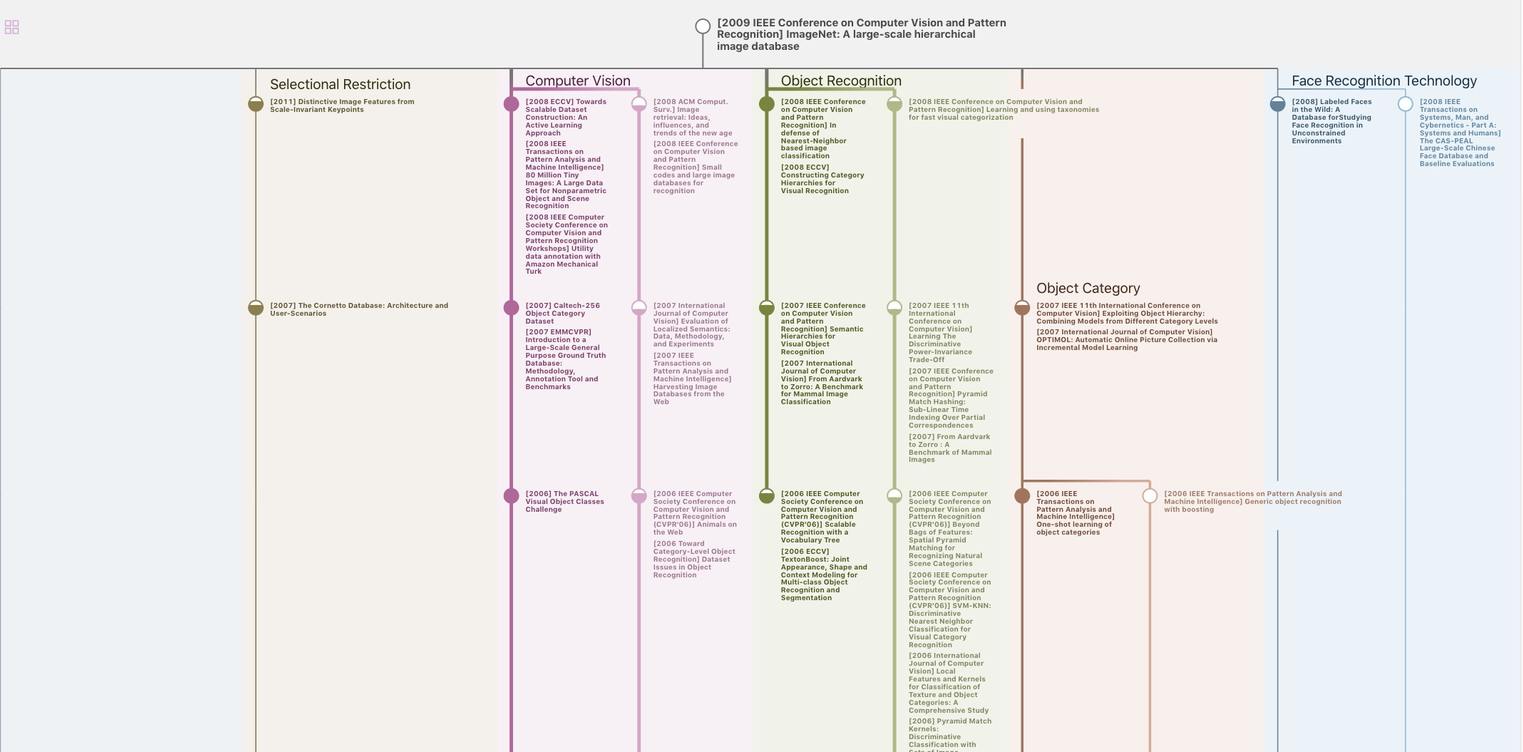
Generate MRT to find the research sequence of this paper
Chat Paper
Summary is being generated by the instructions you defined