Reducing the device complexity for 3D human pose estimation: A deep learning approach using monocular camera and IMUs
Engineering Applications of Artificial Intelligence(2023)
摘要
Camera and inertial measurement unit (IMU) based three-dimensional (3D) human pose estimation flourished in the last decade. However, existing methods are difficult to apply to clinical environments because of the many required devices. In this study, we attempt to reduce the devices and maintain their robustness. Occ-Corrector, a semantic convolution-based neural network, was proposed to estimate 3D human poses robustly in occlusion cases involving a single camera with the help of a few IMUs. It includes a simple Sensor-Reshape, which helps to fuse IMU information with the camera more effectively, and a new strategy of alternating the loss function, allowing the model to improve its accuracy in predicting challenging poses. By conducting an inverse analysis of the weight matrix, the importance of each IMU was investigated for device simplification purposes. The proposed method was verified by using the Total Capture dataset with two hypothetical occlusion conditions, and the result showed that only five IMUs can make the accuracy stable in different occlusion situations.
更多查看译文
关键词
3D human pose estimation, Data fusion, Occlusion, Monocular camera, Inertial measurement unit
AI 理解论文
溯源树
样例
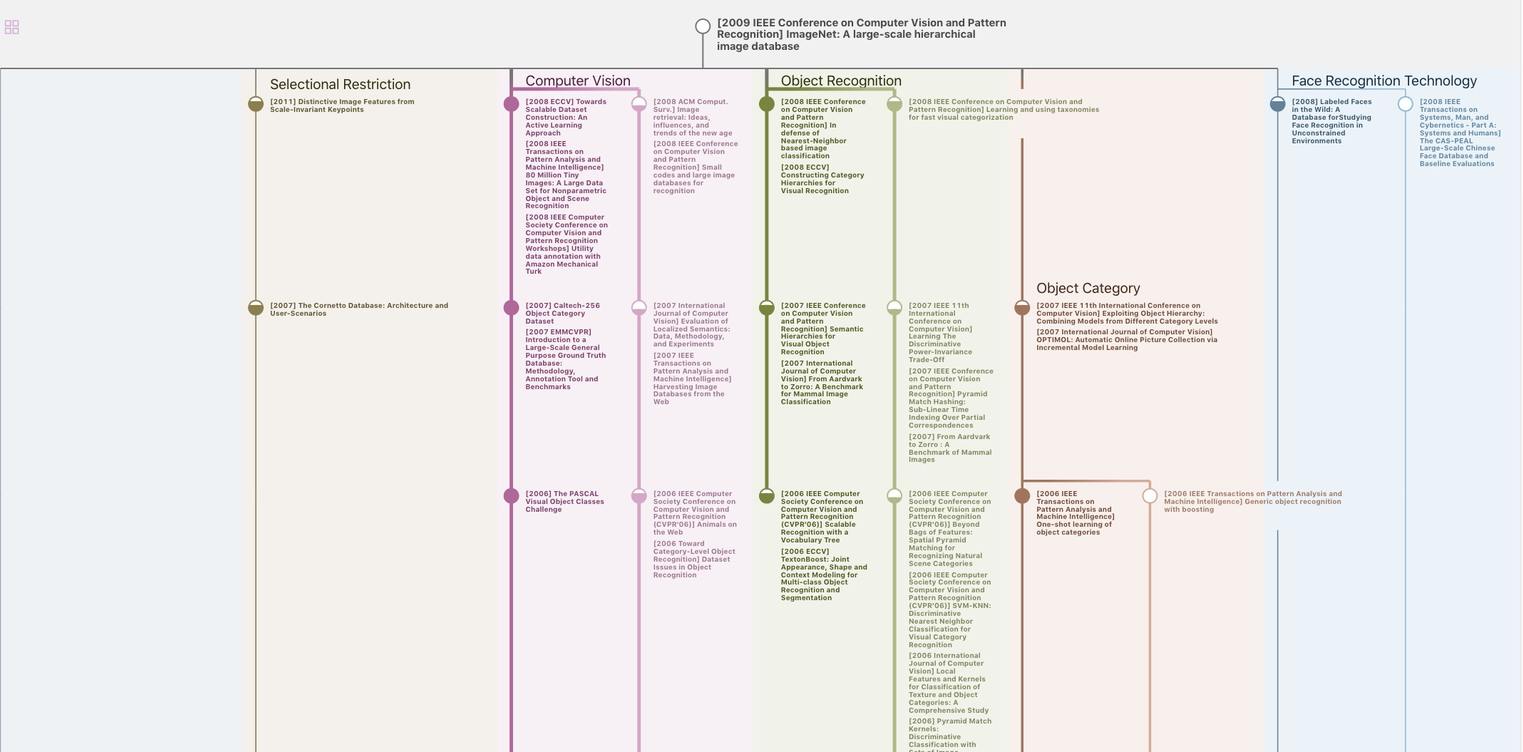
生成溯源树,研究论文发展脉络
Chat Paper
正在生成论文摘要