Forecasting through deep learning and modal decomposition in two-phase concentric jets
Expert Systems with Applications(2023)
摘要
This work aims to improve fuel chamber injectors’ performance in turbofan engines, thus implying improved performance and reduction of pollutants. This requires the development of models that allow real-time prediction and improvement of the fuel/air mixture. However, the work carried out to date involves using experimental data (complicated to measure) or the numerical resolution of the complete problem (computationally prohibitive). The latter involves the resolution of a system of partial differential equations (PDE). These problems make difficult to develop a real-time prediction tool. Therefore, in this work, we propose using machine learning in conjunction with (complementarily cheaper) single-phase flow numerical simulations in the presence of tangential discontinuities to estimate the mixing process in two-phase flows. In this meaning we study the application of two proposed neural network (NN) models11The code of one model and two data sets are available at https://github.com/RAbadiaH/forecasting-through-deep-learning-and-modal-decomposition-in-multi-phase-concentric-jets.git. as PDE surrogate models. Where the future dynamics is predicted by the NN, given some preliminary information. We show the low computational cost required by these models, both in their training and inference phases. We also show how NN training can be improved by reducing data complexity through a modal decomposition technique called higher order dynamic mode decomposition (HODMD), which identifies the main structures inside flow dynamics and reconstructs the original flow using only these main structures. This reconstruction has the same number of samples and spatial dimension as the original flow, but with a less complex dynamics and preserving its main features. The core idea of this work is to test the limits of applicability of deep learning models to data forecasting in complex fluid dynamics problems. Generalization capabilities of the models are demonstrated by using the same NN architectures to forecast the future dynamics of four different two-phase flows.
更多查看译文
关键词
modal decomposition,deep learning,two-phase
AI 理解论文
溯源树
样例
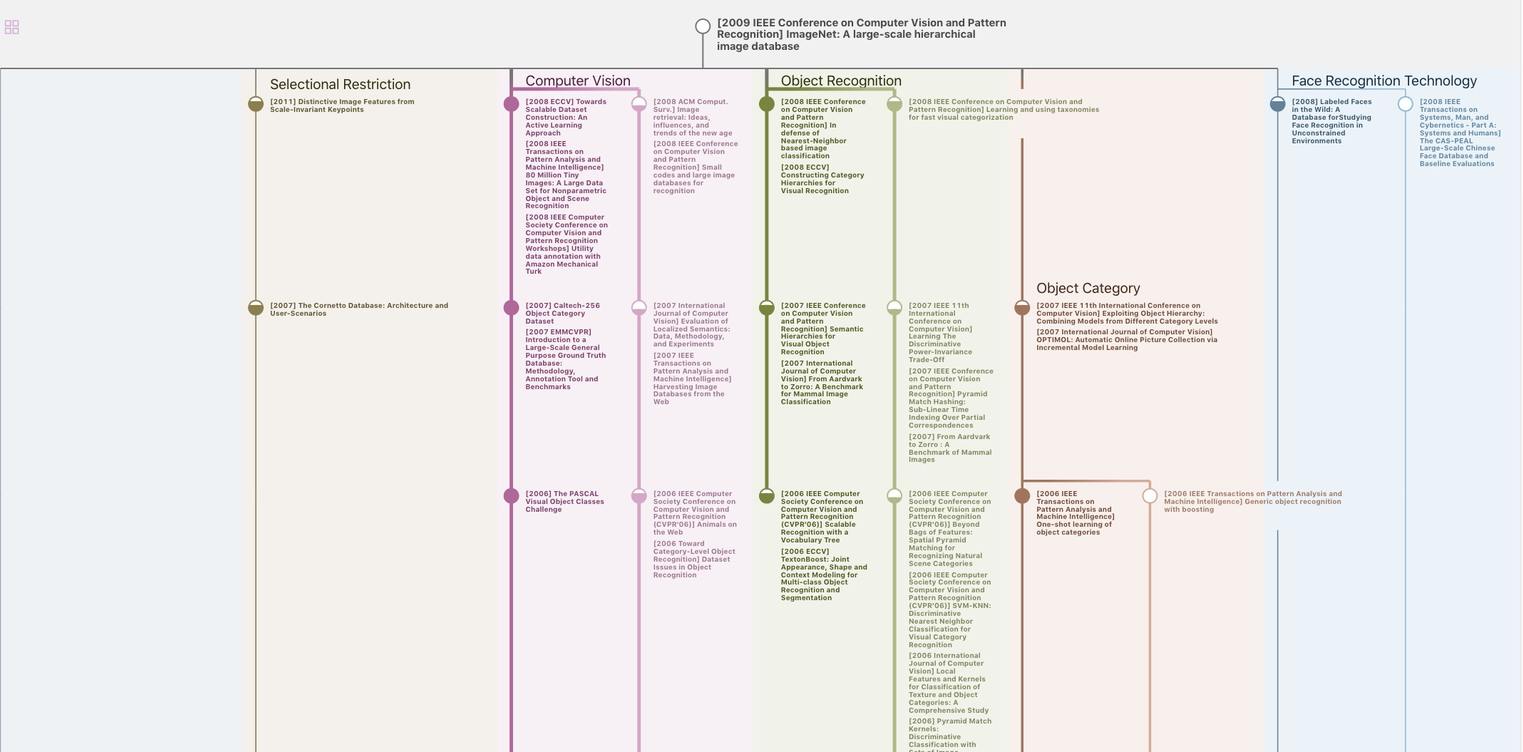
生成溯源树,研究论文发展脉络
Chat Paper
正在生成论文摘要