An Effective Heterogeneous Information Network Representation Learning Framework.
Future Generation Computer Systems(2023)
摘要
Given the heterogeneity of real-world networks and the low efficiency of directly mining networks, heterogeneous information network (HIN) representation learning, which learns low-dimensional embeddings of nodes to represent various structural and semantic information in HINs, becomes a very crucial topic. Existing HIN based representation learning methods either omit the global information of networks, or only consider the first-order neighbors of each node. The two problems reflect the insufficient extraction and exploitation of network information for HIN embedding. To address these problems, we propose a novel Heterogeneous Graph SAmple and aggreGatE framework, named HGSAGE, for learning low-dimensional similarity-preserved embeddings of nodes in HINs. This framework consists of three mechanisms, i.e., the gravity model based on the meta-path reachable graphs mechanism to capture the global information of HINs, the node-level sampling and aggregating mechanism to sample and incorporate features from immediate and mediate neighbors of nodes, and the semantic-level aggregating mechanism to combine embeddings with respect to different meta-paths. Extensive experiments on three real-world heterogeneous networks of different types and scales for multiple tasks show that the proposed framework significantly outperforms the baselines. Moreover, HGSAGE has important application values in this research field.
更多查看译文
关键词
Graph neural networks,Heterogeneous information networks,Network representation learning,HGSAGE
AI 理解论文
溯源树
样例
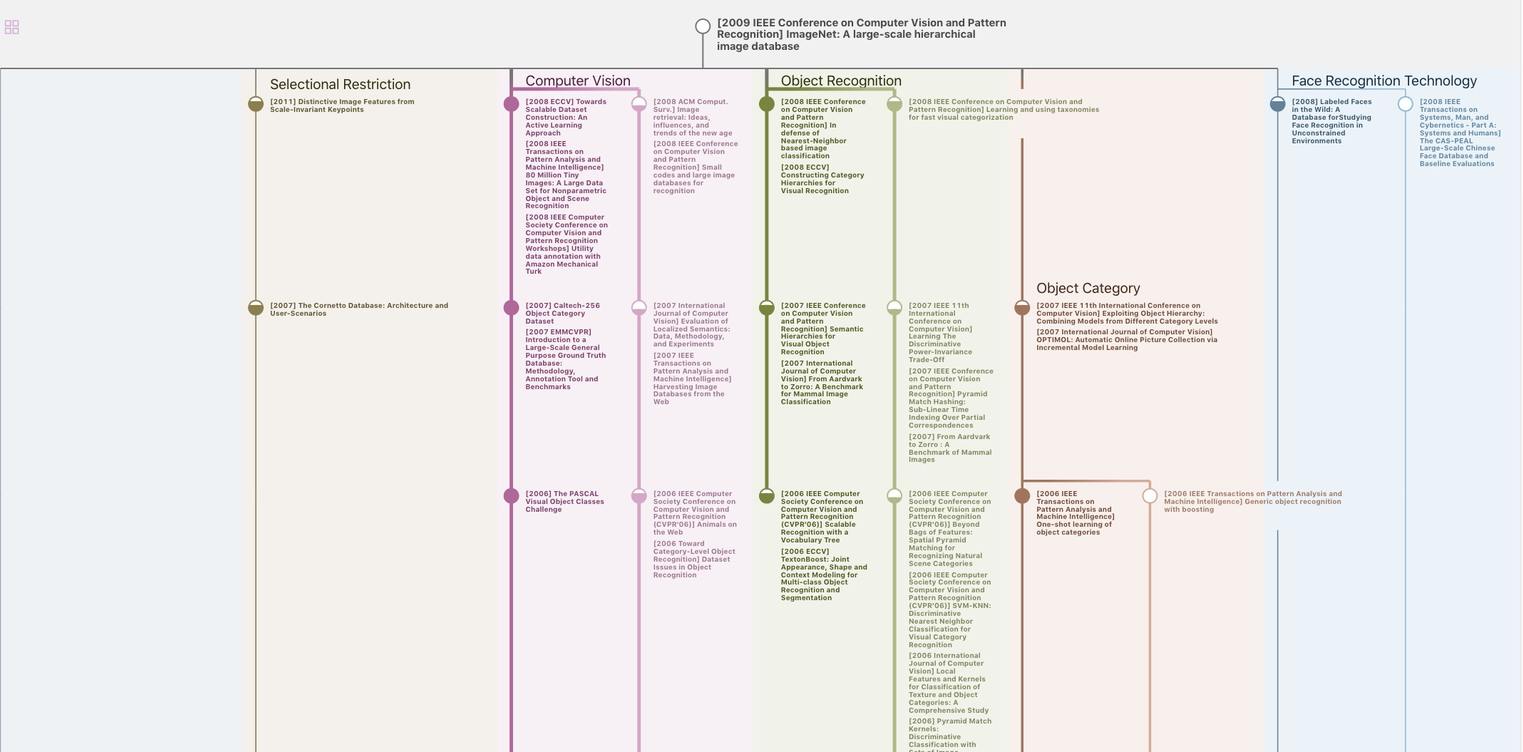
生成溯源树,研究论文发展脉络
Chat Paper
正在生成论文摘要