A hybrid CNN-LSTM machine learning model for rock mechanical parameters evaluation
Geoenergy Science and Engineering(2023)
摘要
With the continuous deepening of hydrocarbon exploration and development, the exploration proportion of unconventional fields has gradually increased, and unconventional energy has become the main component of oil and gas production. Rock mechanical parameters are key to the efficient development of unconventional fields. However, rock mechanical parameters are mostly obtained from expensive measurements such as coring. In this study, a hybrid CNN-LSTM machine learning model is proposed to obtain rock mechanical parameters efficiently through predicting acoustic log in a highly accurate manner. The model incorporates a hybrid multi-channel CNN-LSTM network, which has the advantage of capturing the temporal and spatial features of logs. A group of feature enhancement methods are also proposed and incorporated into the model to improve the reliability of prediction. In this work, the model is utilized to predict acoustic log. Static rock mechanical parameters, which are typically hard to obtain, could be calculated based on dynamic parameters derived from predicted acoustic log. The performance of model is verified by the data of three wells in Jimsar field, and the results show that the introduced model has the highest prediction accuracy for acoustic logs. And the static rock mechanical parameters evaluated via proposed model are subsequently validated against coring data. A good match is achieved, which demonstrates the feasibility of using this model to guide the efficient development of unconventional fields in future.
更多查看译文
关键词
rock,machine learning,cnn-lstm
AI 理解论文
溯源树
样例
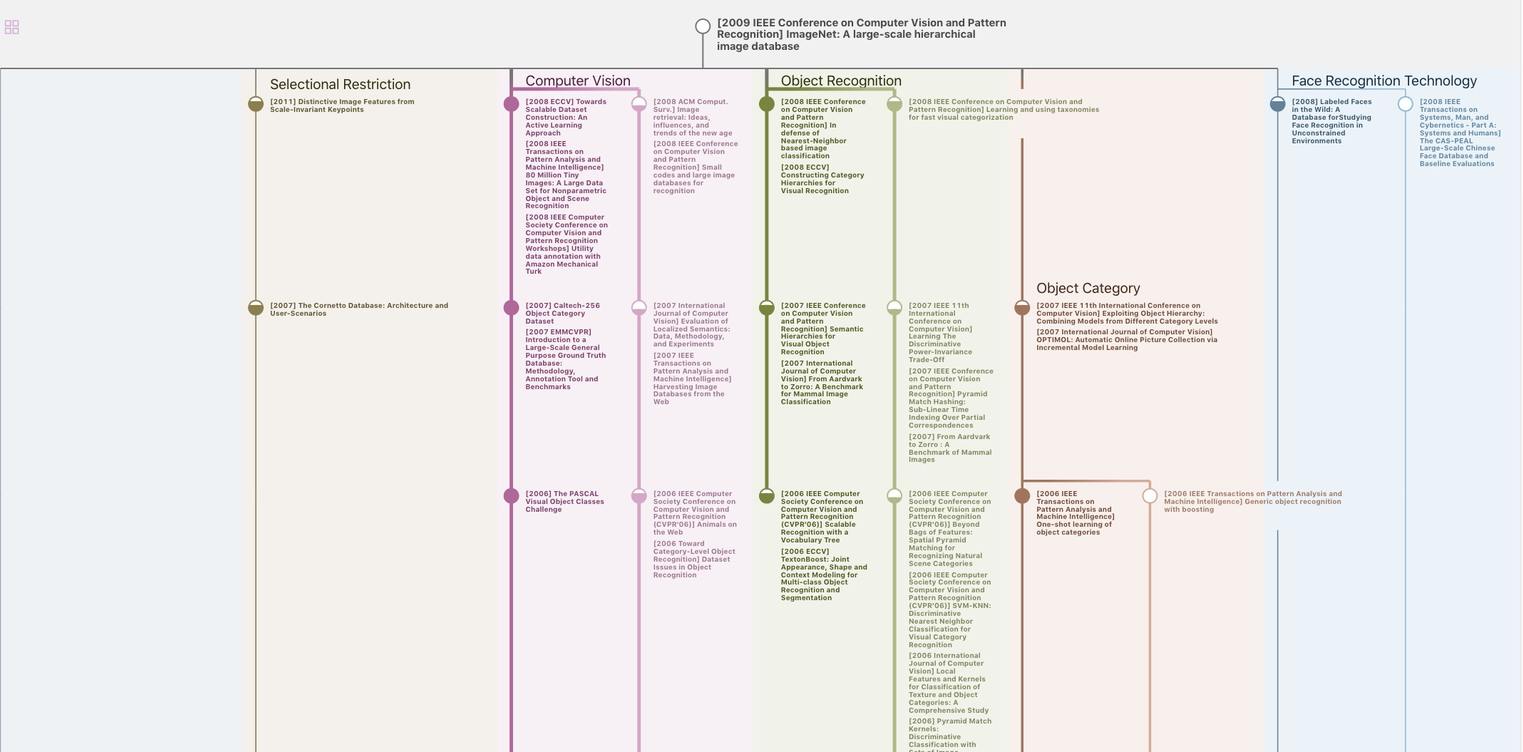
生成溯源树,研究论文发展脉络
Chat Paper
正在生成论文摘要