Integrating the traffic science with representation learning for city-wide network congestion prediction
Information Fusion(2023)
摘要
Recent studies on traffic congestion prediction have paved a promising path towards the reduction of potential economic and environmental loss. However, at the city-wide scale, current approaches face substantial hurdles, such as being unable to support the multiple sensors modalities, insufficient congestion fluctuation and propagation modeling, and weak generalization to heterogeneous traffic network structures. To address these pitfalls, this paper investigates how to integrate the missing urban science domain priors into a general sequential prediction model, and proposes the customized Traffic-informed Transformer (TinT). To prevent receptive field bias, a novel mixture of long and short range information routing mechanism is proposed with the traffic-informed tokenization. To capture the unbalanced traffic flow propagation, an original anisotropic graph aggregation is developed to differentiate the traffic fluctuation based on orientations. Extensive results demonstrated TinT’s outstanding performance over other twelve state-of-the-art models and its broad applicability to multiple data modalities in six well-known cities throughout the world. We released our implementations at: https://github.com/VITA-Group/TinT.
更多查看译文
关键词
Transformer,Traffic prediction,Representation learning,Cyber–physcial system
AI 理解论文
溯源树
样例
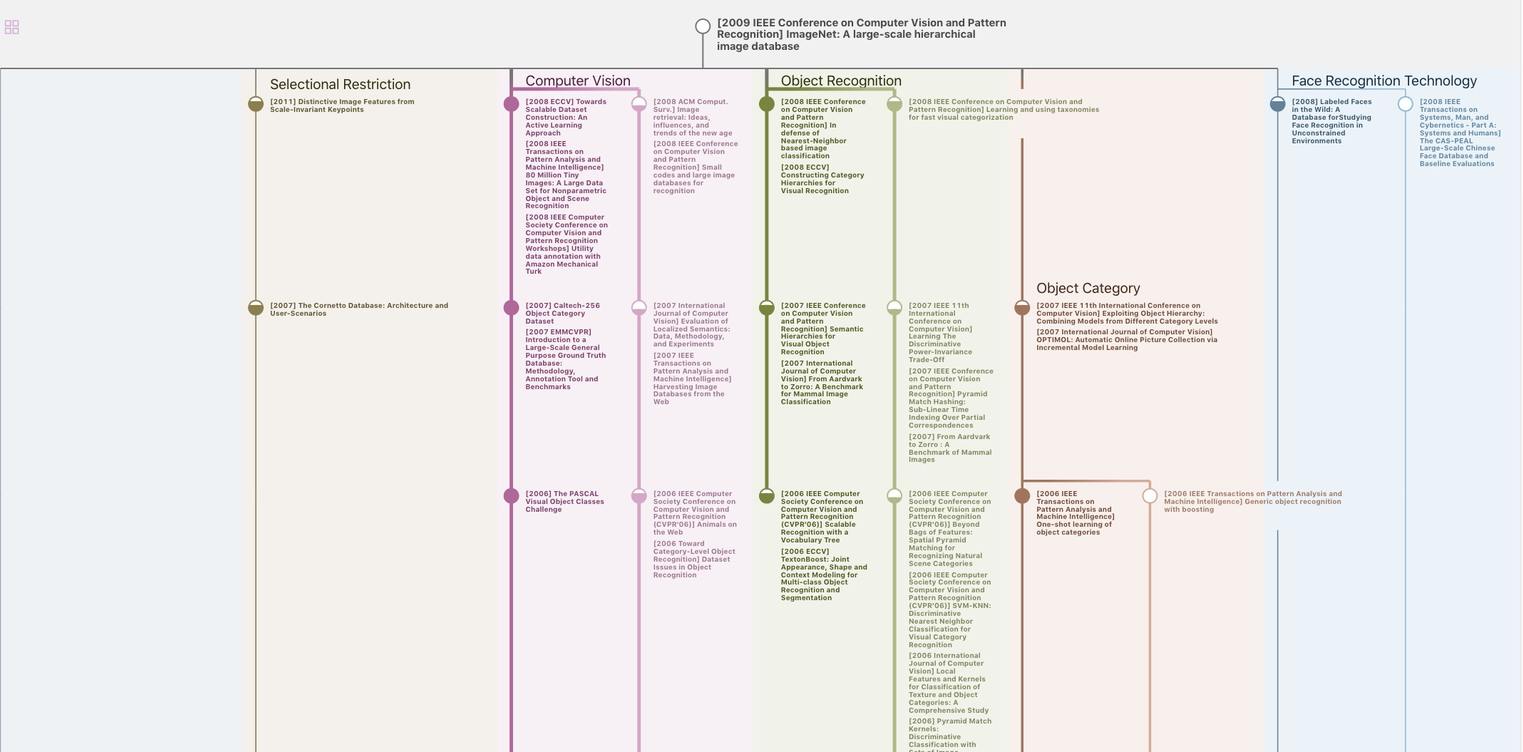
生成溯源树,研究论文发展脉络
Chat Paper
正在生成论文摘要