A novel deep learning model for short-term train delay prediction
Information Sciences(2023)
摘要
As the density of railway operations increases annually, train delays typically result in a greater degree of economic loss. If we can accurately assess the current evolution of delayed trains, railway authorities will be able to make timely decisions and reduce travel anxiety among passengers. In this paper, a deep learning model integrating wavelet transform (WT), fully connected neural network (FCNN), and long short-term memory (LSTM) network is proposed to address the short-term delay prediction problem for trains. Its predictive performance is enhanced by introducing two front train selection schemes, two methods for converting train operating time features into time-series data, and five commonly used wavelet functions. For validation purposes, the model is tested using real-world operational data from two distinct high-speed railway lines in China, and common machine learning algorithms are compared, including random forest (RF), gradient boosting regression tree (GBRT), XGBoosting (XGB), and support vector regression (SVR). The final experimental results demonstrate that our proposed model outperforms the benchmark models and can be effectively applied to practical prediction problems.
更多查看译文
关键词
Deep learning,Train delay prediction,High-speed railway,Wavelet transform,LSTM
AI 理解论文
溯源树
样例
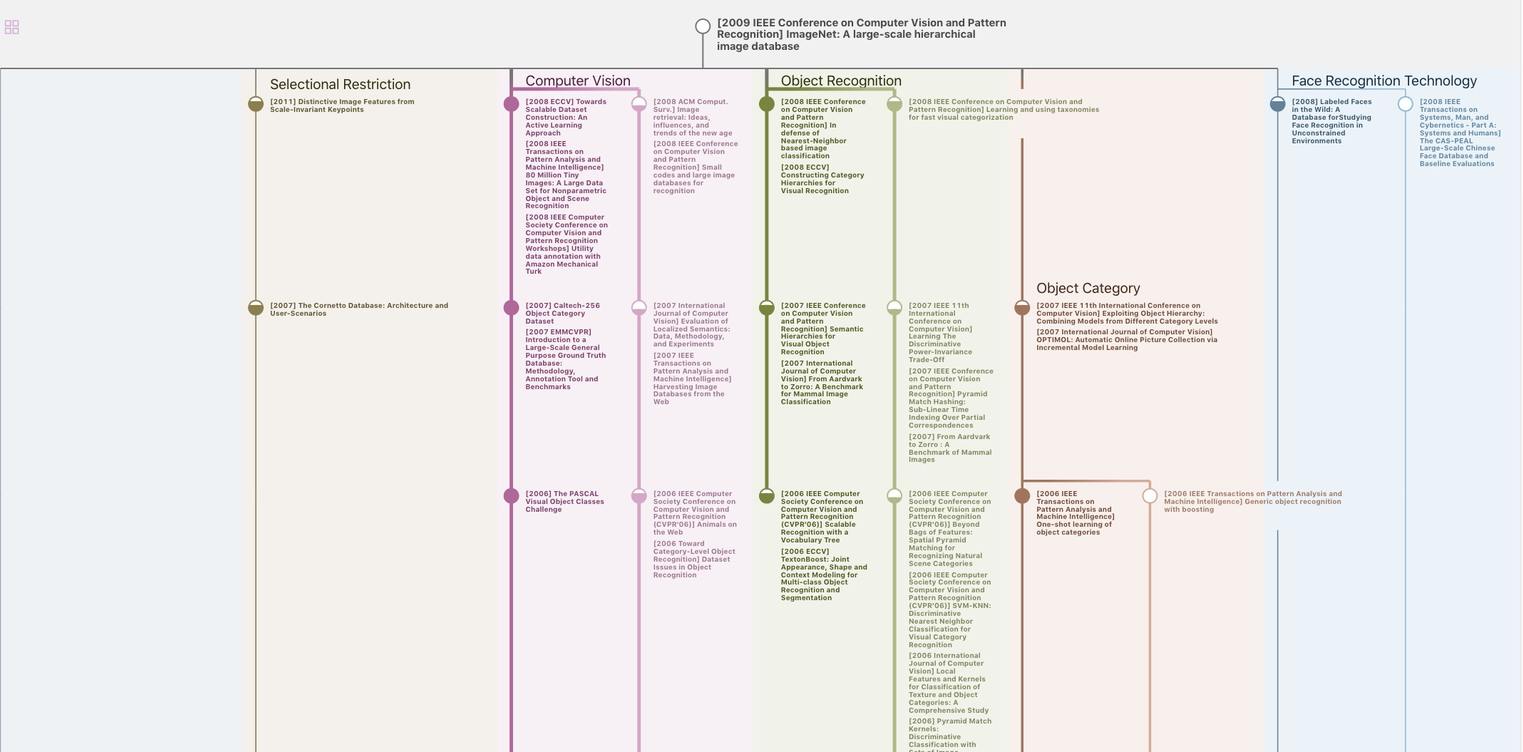
生成溯源树,研究论文发展脉络
Chat Paper
正在生成论文摘要