Three-way decision-based co-detection for outliers
International Journal of Approximate Reasoning(2023)
摘要
Outlier detection is an important research topic in data mining and machine learning. However, existing unsupervised outlier detection methods suffer from irrelevant and redundant attributes in high-dimensional data, and their performance is also limited by their outlier detection models that rely on only one view. In this study, we propose a three-way decision-based co-detection model for unsupervised outlier detection. Specifically, we first improve the local outlier factor (LOF) method by introducing the Gaussian kernel function to make the measure of local reachability density more accurate. Then, we introduce fuzzy rough sets to perform attribute reduction, which further reduces the negative effect of irrelevant and redundant attributes on the measure of sample similarity. Finally, we develop a co-detection model that is trained on the original view and the transformed view generated by principal component analysis and uses the strategy of the three-way decision to collaboratively detect outliers. The results of comparative experiments on the selected UCI datasets show that the proposed model outperforms state-of-the-art methods in terms of AUC-ROC index.
更多查看译文
关键词
Outlier detection,Fuzzy rough sets,Attribute reduction,Three-way decision,Co-detection
AI 理解论文
溯源树
样例
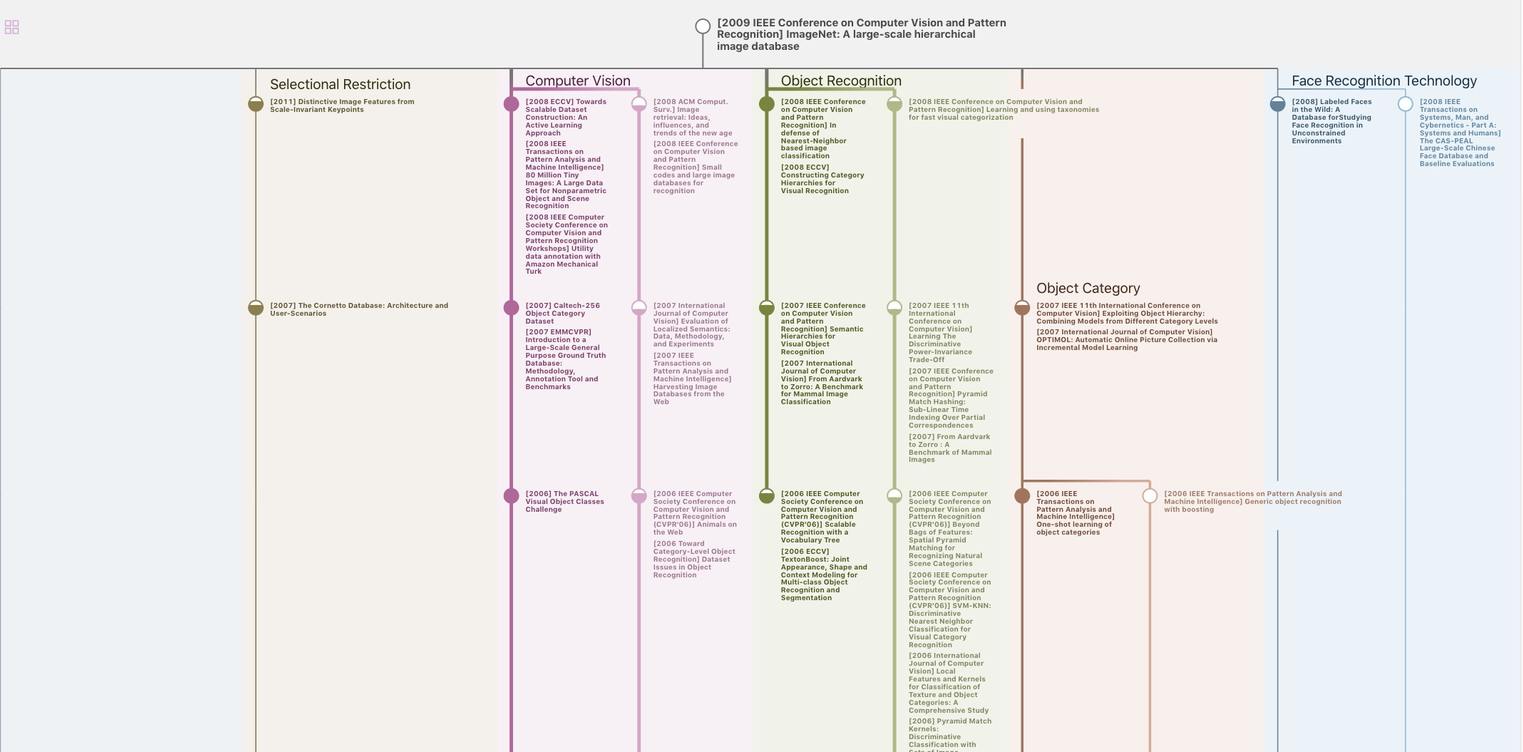
生成溯源树,研究论文发展脉络
Chat Paper
正在生成论文摘要