Predicting battery impedance spectra from 10-second pulse tests under 10 Hz sampling rate.
iScience(2023)
摘要
Onboard measuring the electrochemical impedance spectroscopy (EIS) for lithium-ion batteries is a long-standing issue that limits the technologies such as portable electronics and electric vehicles. Challenges arise from not only the high sampling rate required by the Shannon Sampling Theorem but also the sophisticated real-life battery-using profiles. We here propose a fast and accurate EIS predicting system by combining the fractional-order electric circuit model-a highly nonlinear model with clear physical meanings-with a median-filtered neural network machine learning. Over 1000 load profiles collected under different state-of-charge and state-of-health are utilized for verification, and the root-mean-squared-error of our predictions could be bounded by 1.1 mΩ and 2.1 mΩ when using dynamic profiles last for 3 min and 10 s, respectively. Our method allows using size-varying input data sampled at a rate down to 10 Hz and unlocks opportunities to detect the battery's internal electrochemical characteristics onboard via low-cost embedded sensors.
更多查看译文
关键词
Electrochemical energy storage,Machine learning,Computational materials science
AI 理解论文
溯源树
样例
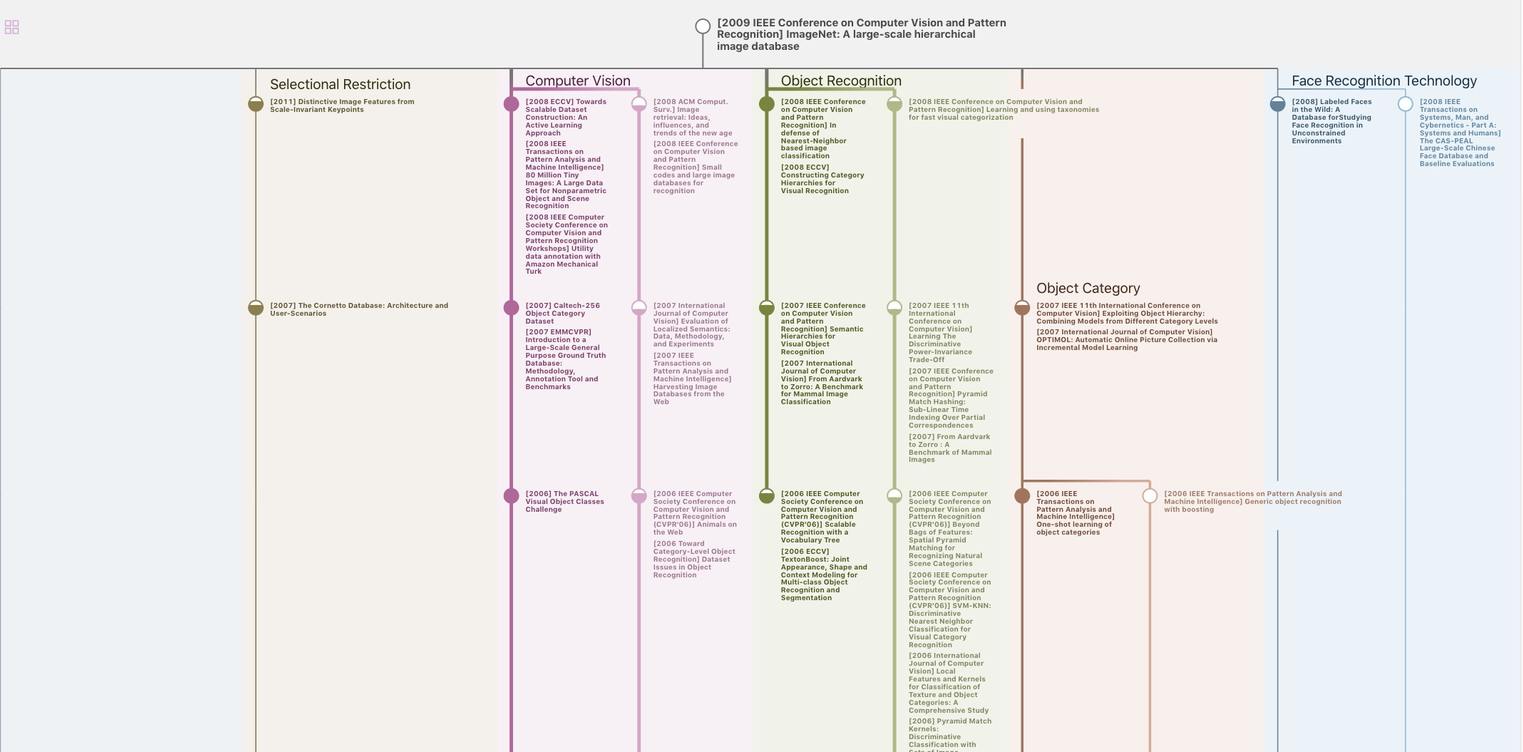
生成溯源树,研究论文发展脉络
Chat Paper
正在生成论文摘要