Post-processed posteriors for sparse covariances
Journal of Econometrics(2023)
摘要
We consider Bayesian inference of sparse covariance matrices and propose a post-processed posterior. This method consists of two steps. In the first step, posterior samples are obtained from the conjugate inverse-Wishart posterior without considering the sparse structural assumption. The posterior samples are transformed in the second step to satisfy the sparse structural assumption through a generalized thresholding function. This non-traditional Bayesian procedure is justified by showing that the post-processed posterior attains the optimal minimax rates under the spectral norm loss in high-dimensional settings. We also propose the post-processed posterior for contaminated data and apply it to the estimation of the sparse idiosyncratic covariance of the approximate factor model. The advantages of our method are demonstrated via a simulation study and a real data analysis with S&P 500 data.
更多查看译文
关键词
Covariance estimation,Bayesian inference,High-dimensional analysis,Minimax analysis,Idiosyncratic covariance
AI 理解论文
溯源树
样例
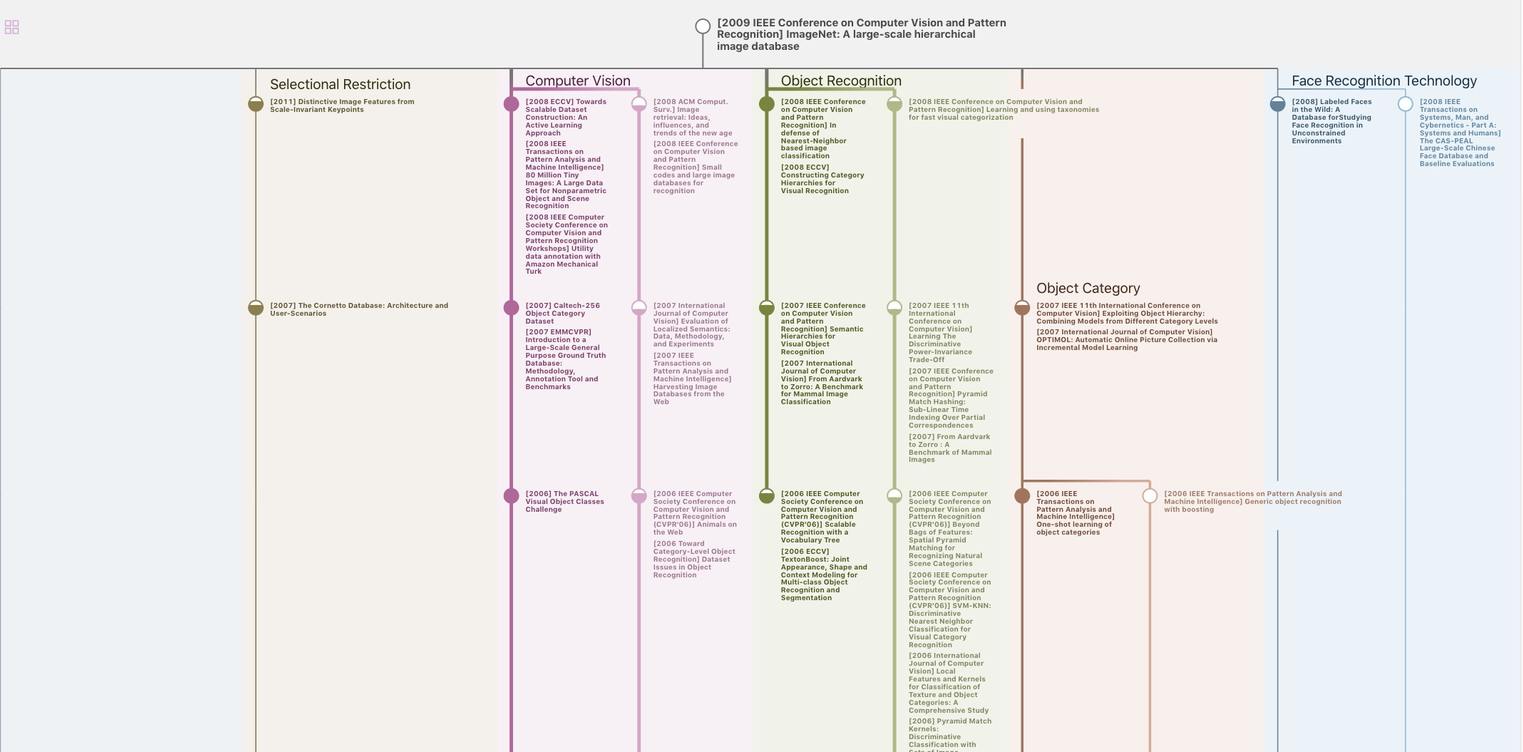
生成溯源树,研究论文发展脉络
Chat Paper
正在生成论文摘要