Ability-aware knowledge distillation for resource-constrained embedded devices
Journal of Systems Architecture(2023)
摘要
Deep Neural Network (DNN) models have notably improved the efficiency of machine learning tasks. However, their high storage and computational costs restrict their deployment on resource-limited embedded devices. Knowledge distillation (KD) has emerged as a promising approach for compressing DNN models. However, two challenges in KD, namely the capacity gap problem and the time-consuming redundancy problem, have hindered its performance and efficiency in compression. To alleviate these challenges, this paper proposes a novel framework, called Ability-Aware Knowledge Distillation (AAKD). AAKD introduces a knowledge sample selection strategy and an adaptive teacher switching strategy based on the dynamic awareness of the student’s ability. This enables the framework to automatically select suitable knowledge samples and teacher networks according to the increasing representation ability of students. Extensive experiments on different datasets and models have demonstrated that AAKD can enhance the performance of compact student models, significantly improve the efficiency of distillation, and lead to higher compression rates.
更多查看译文
关键词
Embedded devices,Model compression,Knowledge distillation
AI 理解论文
溯源树
样例
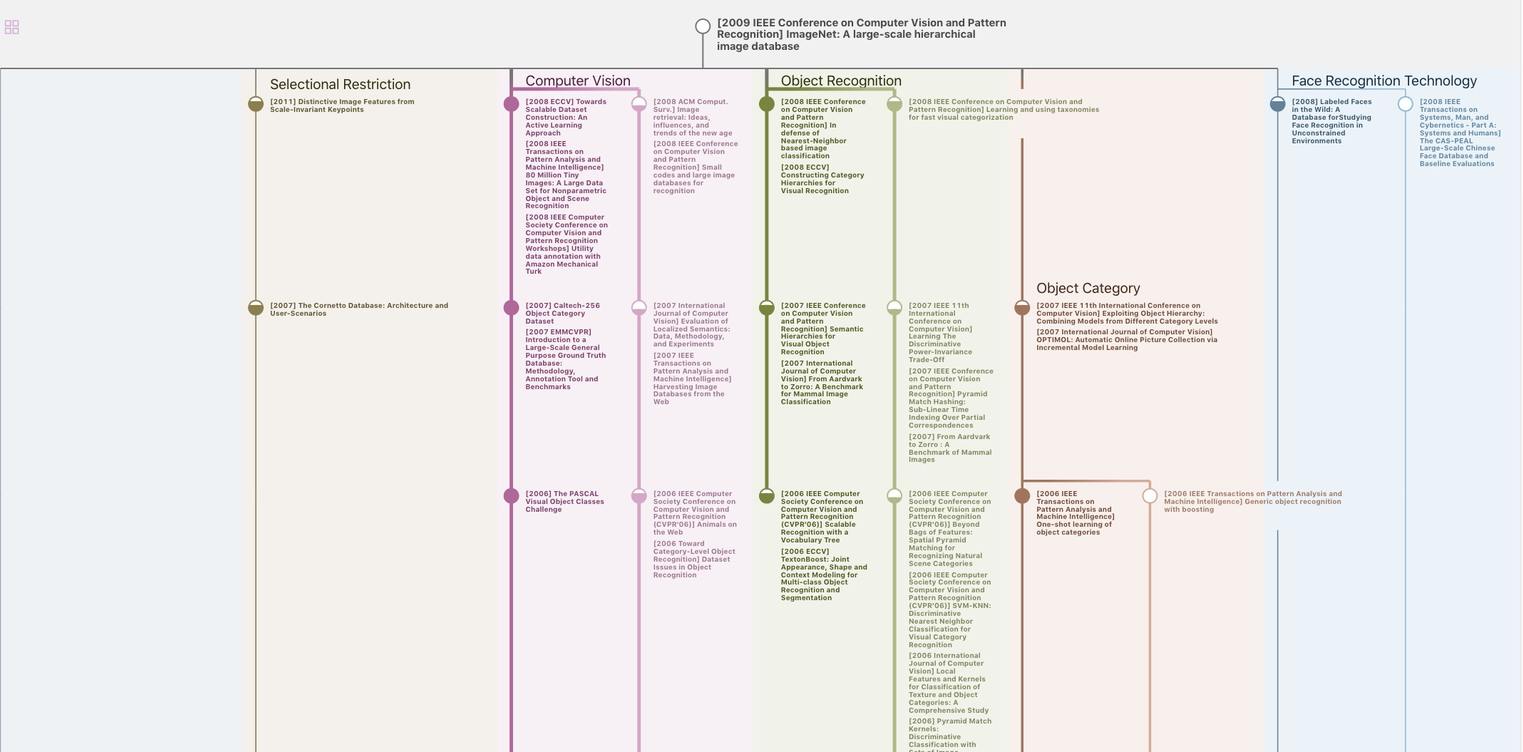
生成溯源树,研究论文发展脉络
Chat Paper
正在生成论文摘要