Combatting the mismatch: Modeling bike-sharing rental and return machine learning classification forecast in Seoul, South Korea
Journal of Transport Geography(2023)
摘要
Bike-sharing is rapidly gaining popularity due to health, transportation, and recreational benefits. As more people use bike-sharing, the burden of reallocating bikes will increase because of the mismatch between outgoing and incoming bikes. Optimizing truck routes, incentivizing users, and crowdsourcing are common suggestions to mitigate rebalancing issues. This research aims to provide a procedure to adjust landscape conditions as an alternative strategy. Comprehensive landscape metrics are quantified by FRAGSTATS analysis. Using public bike-sharing data in Seoul, South Korea, we analyzed spatial and temporal mismatch characteristics. Hot spot analysis was conducted to identify hot and cold spots of bike-sharing use in two scenarios: outgoing and incoming trips. This was used to generate tree-based binary ensemble machine learning classification models. Shapley Additive exPlanations (SHAP) values were calculated between hot and cold spots to understand how landscape characteristics and other determinants affect the mismatch. Our results suggest that climate and bike-sharing related factors significantly affect bike-sharing use. Transportation land use and landscape characteristics like the magnitude of biodiversity, contiguity, shape, area, and edge significantly contribute to labeling. The findings of this study can help bike-sharing operators better navigate their bike-sharing services.
更多查看译文
关键词
classification forecast,machine learning,bike-sharing
AI 理解论文
溯源树
样例
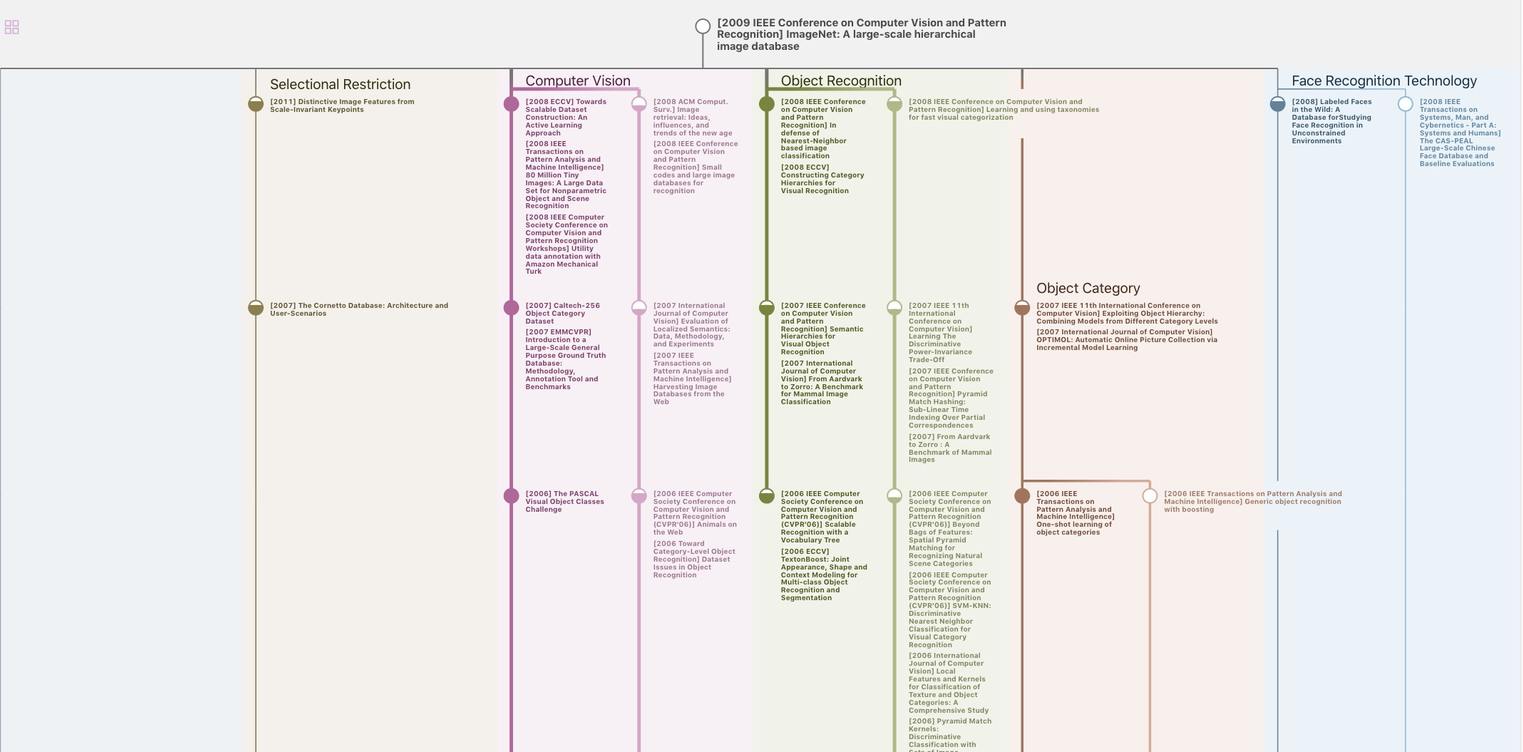
生成溯源树,研究论文发展脉络
Chat Paper
正在生成论文摘要