Hybrid machine learning models for prediction of daily dissolved oxygen
Journal of Water Process Engineering(2023)
摘要
Measuring water quality parameters is a significant step in many hydrological assessments. Dissolved oxygen (DO) is one of these parameters that is an indicator of water quality. Hence, this study offers two novel intelligent models, i.e., the integration of biogeography-based optimization (BBO) and atom search optimization (ASO) with artificial neural network (ANN), to predict the daily DO. These methods are comparatively assessed and validated against several benchmark techniques. Five-year (2014–2019) water quality data of a USGS station called Rock Creek (Station number 01648010) is used for implementing the proposed model. In this sense, the models first learn the DO behavior using 80 % of the data and they then predict the DO for the fifth year. As per the performed sensitivity analysis, the water temperature was selected as the most effective parameter in the DO prediction. Trying different population sizes determined an optimal configuration of the employed models and assessing the accuracy of the results revealed that the proposed models can nicely perceive the DO pattern with around 4 % mean absolute percentage error (MAPE) and 97.5 % correlation. In the testing phase, the BBO-ANN and ASO-ANN models predicted the DO of the fifth year with MAPEs 2.3848 and 2.5170 %, and correlations of 0.99186 and 0.99135, respectively. Moreover, the suggested BBO-ANN and ASO-ANN outperformed some similar hybrids from the existing literature. Lastly, an explicit formula is derived from the BBO-ANN for convenient prediction of the DO.
更多查看译文
关键词
Water quality assessment,Dissolved oxygen,Biogeography-based optimization,Artificial neural network
AI 理解论文
溯源树
样例
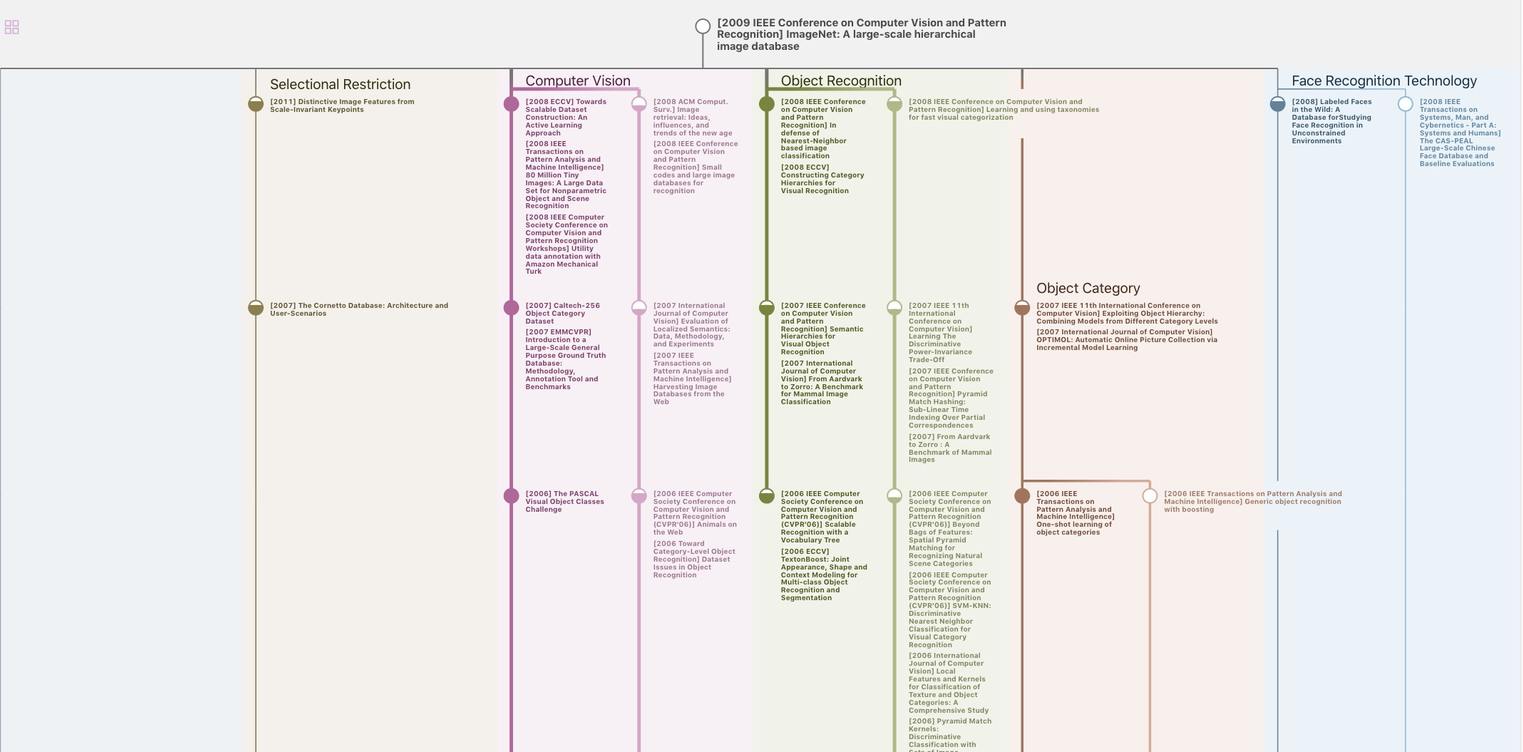
生成溯源树,研究论文发展脉络
Chat Paper
正在生成论文摘要