CLVIN: Complete language-vision interaction network for visual question answering
Knowledge-Based Systems(2023)
摘要
The emergence of the Transformer optimizes the interactive modeling of multimodal information in visual question answering (VQA) tasks, helping machines better understand multimodal information. The existing Transformer-based end-to-end methods have made some achievements in applying the Encoder-Decoder (E-D) mode or realizing complete interaction. However, almost no methods combine the advantages of the two well and give full play to them. Thus, this paper designs a complete language-vision interaction network (CLVIN) for VQA based on the implementation of the quadratic E-D mode. Based on the core framework of the modular co-attention network (MCAN), CLVIN achieves the complete interaction of multimodal information by using the E-D mode again, realizing the rational distribution of the question words’ weight information. In addition, to reduce the additional consumption of time and memory caused by introducing the quadratic E-D mode, this paper proposes a compact method called CLVIN-c through optimizing the underlying implementation of the scaled dot-product attention in Transformer. Finally, a series of experimental results based on the dataset VQA-v2.0 and CLEVR show that CLVIN has a significant performance improvement, and CLVIN-c achieves further optimizations in model size and performance. Code is available at https://github.com/RainyMoo/myvqa.
更多查看译文
关键词
Interactive modeling,Multimodal information,E-D mode,Language-vision interaction,Complete interaction
AI 理解论文
溯源树
样例
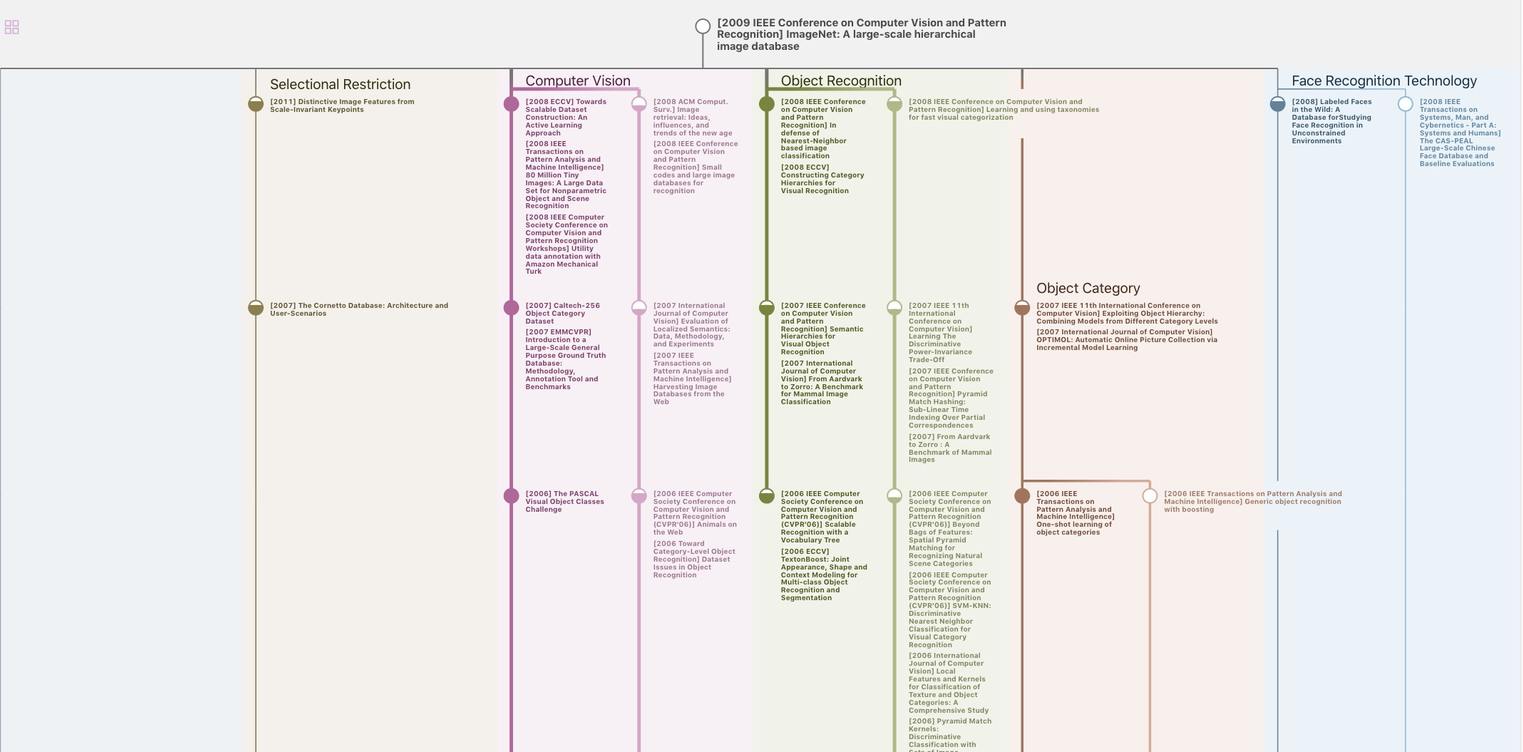
生成溯源树,研究论文发展脉络
Chat Paper
正在生成论文摘要