Internal Defect Detection Quantification and Three-Dimensional Localization Based on Impact Echo and Classification Learning Model
MEASUREMENT(2023)
Southeast Univ | China Railway Construction Suzhou Design & Res Ins
Abstract
Accurately identifying, localizing, and characterizing internal defects is crucial for ensuring the safety and durability of concrete structures. While impact echo (IE) is a highly promising non-destructive testing method for detecting concrete internal defects, previous studies have primarily focused on defect identification with less emphasis on quantification and depth localization of internal defects. In this paper, we propose an intelligent detection method based on IE and deep learning to achieve intelligent identification, area quantification, and depth localization of concrete internal defects. The proposed method includes the following three components: (1) A one-dimensional model combining wavelet packet decomposition and the Gate Recurrent Unit network was proposed to achieve an automatic diagnosis of defect signals inside concrete structures. (2) A method incorporating defect identification probability heatmap and threshold segmentation is employed to quantify the concrete defect area and detect the defect area detection rate. (3) A two-dimensional model combining wavelet transform and convolutional neural network was developed to achieve defect depth localization. The proposed method has been effectively validated in laboratory experiments involving concrete slabs with artificial defects.
MoreTranslated text
Key words
Defect recognition,Impact echo,Deep learning,Threshold segmentation,Region quantization,Depth localization
求助PDF
上传PDF
View via Publisher
AI Read Science
AI Summary
AI Summary is the key point extracted automatically understanding the full text of the paper, including the background, methods, results, conclusions, icons and other key content, so that you can get the outline of the paper at a glance.
Example
Background
Key content
Introduction
Methods
Results
Related work
Fund
Key content
- Pretraining has recently greatly promoted the development of natural language processing (NLP)
- We show that M6 outperforms the baselines in multimodal downstream tasks, and the large M6 with 10 parameters can reach a better performance
- We propose a method called M6 that is able to process information of multiple modalities and perform both single-modal and cross-modal understanding and generation
- The model is scaled to large model with 10 billion parameters with sophisticated deployment, and the 10 -parameter M6-large is the largest pretrained model in Chinese
- Experimental results show that our proposed M6 outperforms the baseline in a number of downstream tasks concerning both single modality and multiple modalities We will continue the pretraining of extremely large models by increasing data to explore the limit of its performance
Upload PDF to Generate Summary
Must-Reading Tree
Example
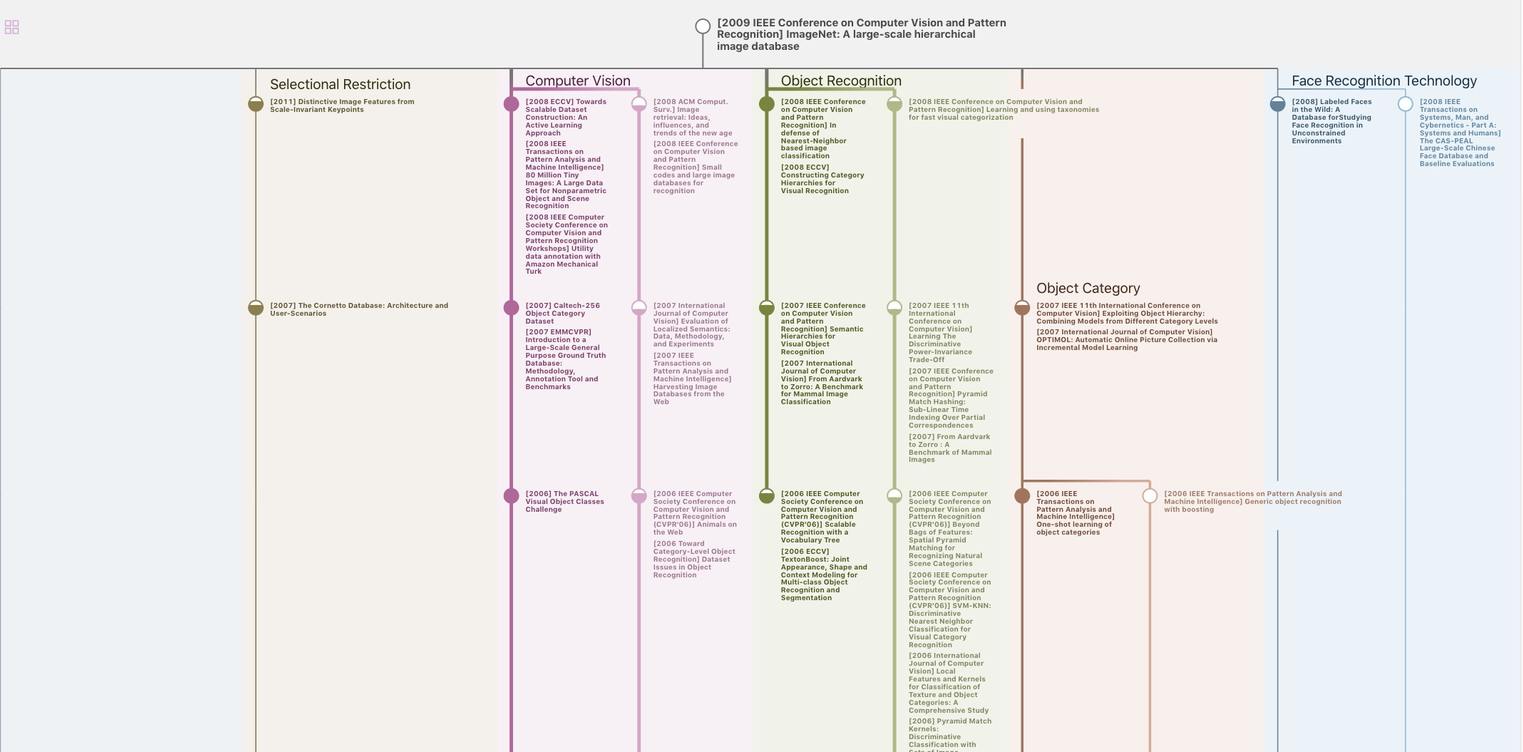
Generate MRT to find the research sequence of this paper
Related Papers
MATERIALS TODAY COMMUNICATIONS 2023
被引用10
Two-step Detection of Concrete Internal Condition Using Array Ultrasound and Deep Learning
NDT & E INTERNATIONAL 2023
被引用13
CSG Compressive Strength Prediction Based on LSTM and Interpretable Machine Learning
REVIEWS ON ADVANCED MATERIALS SCIENCE 2023
被引用1
ENGINEERING STRUCTURES 2024
被引用1
Concrete Acoustic Emission Signal Augmentation Method Based on Generative Adversarial Networks
MEASUREMENT 2024
被引用1
Ensemble Learning Model for Concrete Delamination Depth Detection Using Impact Echo
NDT & E International 2024
被引用0
Ultrasonic Synthetic Aperture Imaging for Inner Defect of Concrete Based on Delay-Multiple-and-Sum
Traitement du signal 2024
被引用0
Data Disclaimer
The page data are from open Internet sources, cooperative publishers and automatic analysis results through AI technology. We do not make any commitments and guarantees for the validity, accuracy, correctness, reliability, completeness and timeliness of the page data. If you have any questions, please contact us by email: report@aminer.cn
Chat Paper