Simple is good: Investigation of history-state ensemble deep neural networks and their validation on rotating machinery fault diagnosis
Neurocomputing(2023)
摘要
The present work is motivated by the desire to find an efficient approach that can improve the perfor-mance of deep neural networks in a general sense. To this end, an easy-to-implement ensemble approach is proposed in this paper leveraging the 'local sub-optima' of deep networks, which is referred as to history-state ensemble (HSE) method. We demonstrated that neural networks can naturally generate multiple 'local sub-optima' with diversity during training process, and their combination can effectively improve the accuracy and stability of the single network. The merits of HSE are twofold: (1) It does not require additional training cost in order to acquire multiple base models, which is one of the main draw-backs limiting the generalization of ensemble techniques in deep learning. (2) It can be easily applied to any types of deep networks without tuning of network architectures. We proposed the simplest way to perform HSE and investigated more than 20 ensemble strategies for HSE as comparison. Experiments are conducted on six datasets and eight popular network architectures for the case of rotating machinery fault diagnosis. It is demonstrated that the stability and accuracy of neural networks can be generally im-proved through the simplest ensemble strategy proposed in this paper.& COPY; 2023 The Author(s). Published by Elsevier B.V. This is an open access article under the CC BY license (http://creativecommons.org/licenses/by/4.0/).
更多查看译文
关键词
History-state ensemble (HSE),Ensemble learning,Deep neural networks,Average voting (AV),Fault diagnosis
AI 理解论文
溯源树
样例
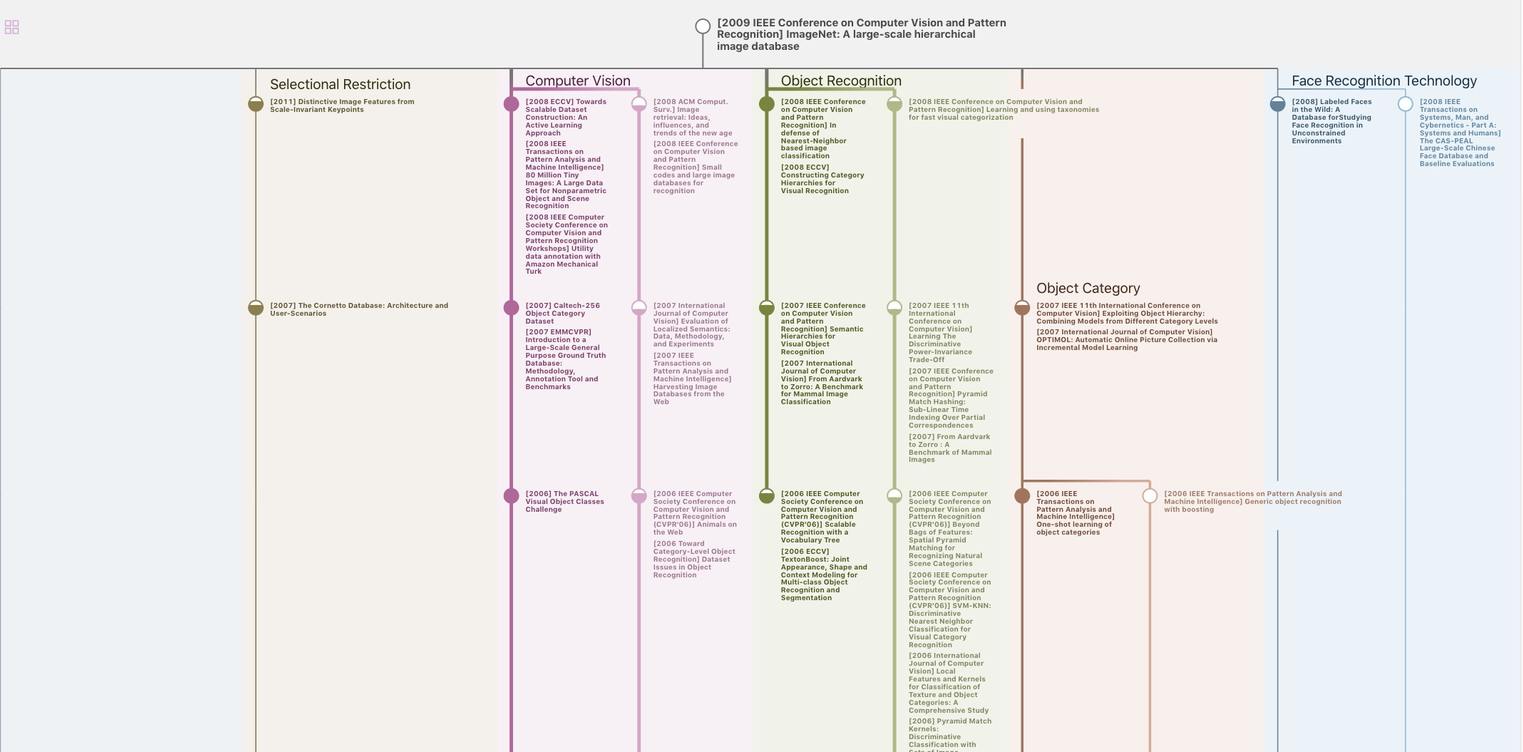
生成溯源树,研究论文发展脉络
Chat Paper
正在生成论文摘要