Combining CNN and transformers for full-reference and no-reference image quality assessment
Neurocomputing(2023)
摘要
Most deep learning approaches for image quality assessment use regression from deep features extracted by CNN (Convolutional Neural Networks). However, non-local information is usually neglected in exist-ing methods. Motivated by the recent success of transformers in modeling contextual information, we propose a hybrid framework that utilizes a vision transformer backbone to extract features and a CNN decoder for quality estimation. We propose a shared feature extraction scheme for both FR and NR set-tings. A two-branch structured attentive quality predictor is devised for quality prediction. Evaluation experiments on various IQA datasets, including LIVE, CSIQ and TID2013, LIVE-Challenge, KADID-10 K, and KONIQ-10 K, show that our proposed models achieve outstanding performance for both FR and NR settings.& COPY; 2023 Published by Elsevier B.V.
更多查看译文
关键词
Image quality assessment,Convolutional neural network,Transformers,Non-local information
AI 理解论文
溯源树
样例
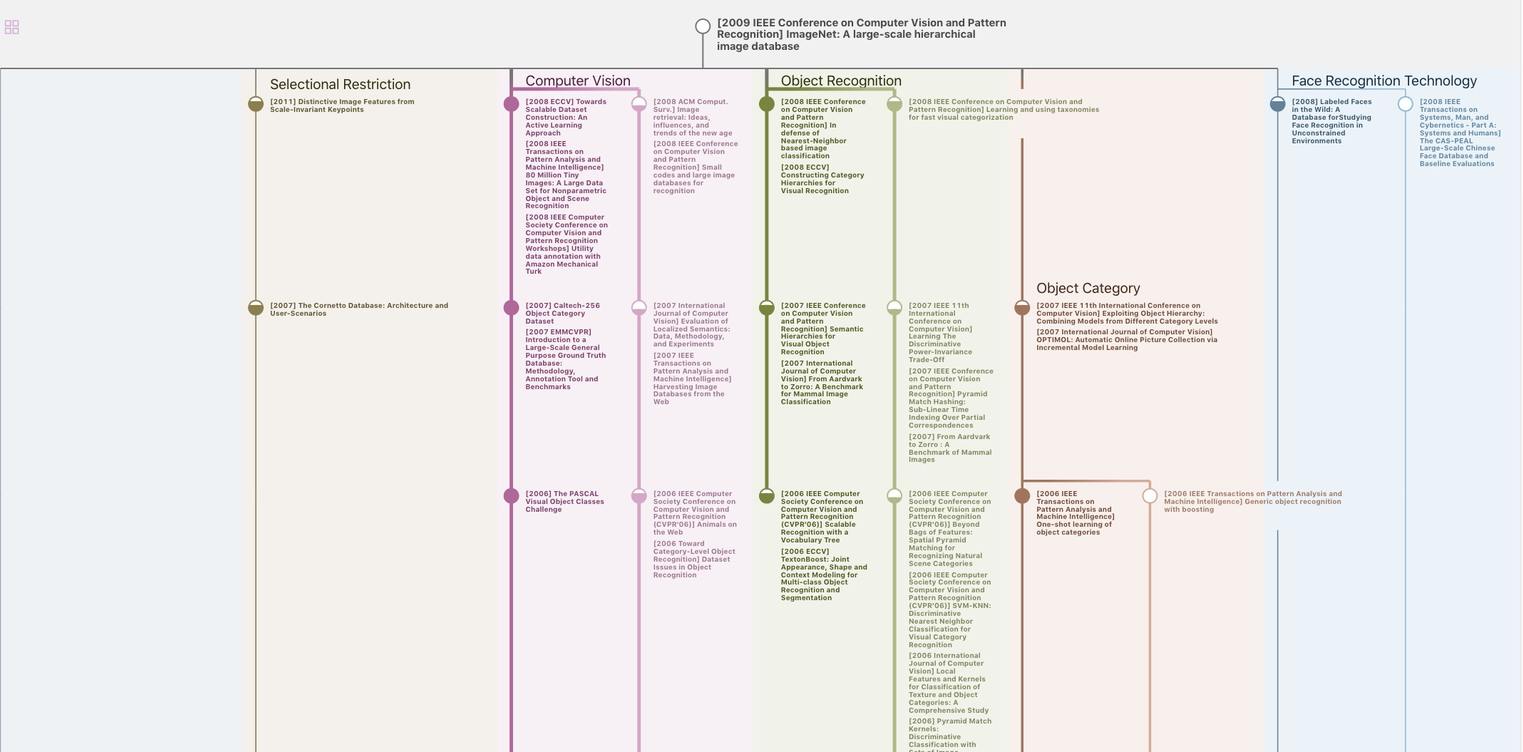
生成溯源树,研究论文发展脉络
Chat Paper
正在生成论文摘要