An ASM-CF model for anomalous trajectory detection with mobile trajectory big data
Physica A: Statistical Mechanics and its Applications(2023)
摘要
Anomalous trajectory detection is an essential research hotspot of mobile trajectory big data mining and analytics, significantly improving residents’ travel experience and strengthening urban public security management in intelligent transportation systems. Most of the existing anomalous trajectory detection methods only employ spatial features rather than temporal features to mine and analyze anomalous trajectories of passenger travel, especially with low efficiency and accuracy problems. To this end, this paper proposes a cost-factor-based anomaly score model (ASM-CF) to detect anomalous trajectories of detour behavior. Specifically, we first design an urban road network rasterization approach that expresses the trajectory in a grid sequence. The trajectory is enhanced to obtain a continuous grid sequence trajectory, which solves the problem of driving the same path but recoding different trajectory points. Then, a method for accurately detecting anomalous trajectories of taxi drivers’ detours is put forward to identify anomalous travel trajectories based on detours. Next, a cost factor based on distance and duration is constructed, and an ASM-CF model is established by the cost factor to improve the accuracy of anomalous trajectory detection. Finally, the experimental results from an empirical study demonstrate that the F1-score of ASM-CF is 0.960, 0.977, 0.927, 0.976, and 0.993 in different trajectory pair datasets based on the real-world taxi trajectory big data, which can effectively detect trajectory anomaly degree and accurately identify anomalous behaviors such as not following the normal route and malicious detours. In particular, compared with the iBAT, ATDC, XGBoost, and ATD-RNN models, the F1-score of ASM-CF is improved by at least 8.045%, 3.644%, 8.166%, and 12.835%, respectively.
更多查看译文
关键词
anomalous trajectory detection,mobile trajectory,big data
AI 理解论文
溯源树
样例
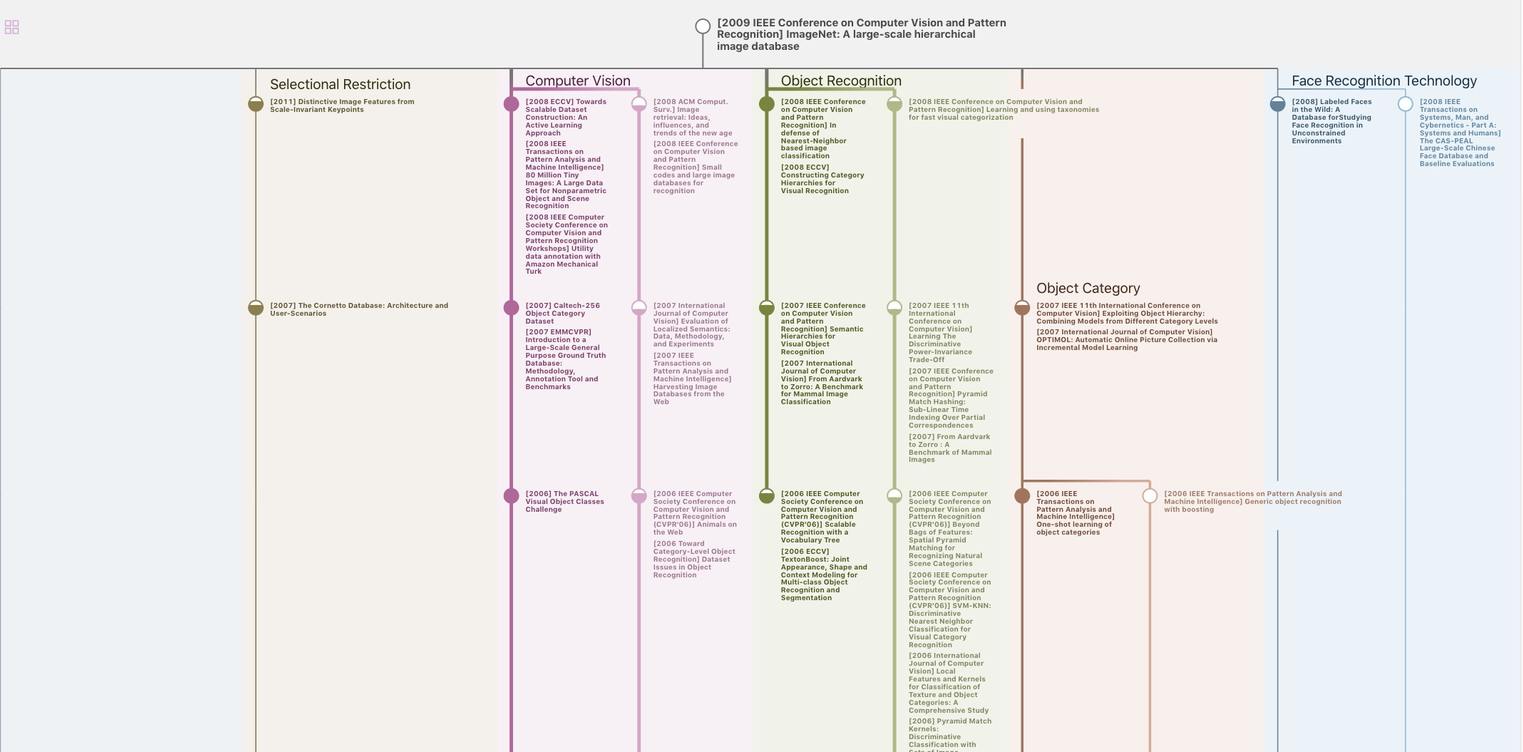
生成溯源树,研究论文发展脉络
Chat Paper
正在生成论文摘要