Automated Deep Learning Empowered Breast Cancer Diagnosis Using Biomedical Mammogram Images
CMC-COMPUTERS MATERIALS & CONTINUA(2022)
摘要
Biomedical image processing is a hot research topic which helps to majorly assist the disease diagnostic process. At the same time, breast cancer becomes the deadliest disease among women and can be detected by the use of different imaging techniques. Digital mammograms can be used for the earlier identification and diagnostic of breast cancer to minimize the death rate. But the proper identification of breast cancer has mainly relied on the mammography findings and results to increased false positives. For resolving the issues of false positives of breast cancer diagnosis, this paper presents an automated deep learning based breast cancer diagnosis (ADL-BCD) model using digital mammograms. The goal of the ADL-BCD technique is to properly detect the existence of breast lesions using digital mammograms. The proposed model involves Gaussian filter based pre-processing and Tsallis entropy based image segmentation. In addition, Deep Convolutional Neural Network based Residual Network (ResNet 34) is applied for feature extraction purposes. Specifically, a hyper parameter tuning process using chimp optimization algorithm (COA) is applied to tune the parameters involved in ResNet 34 model. The wavelet neural network (WNN) is used for the classification of digital mammograms for the detection of breast cancer. The ADL-BCD method is evaluated using a benchmark dataset and the results are analyzed under several performance measures. The simulation outcome indicated that the ADL-BCD model outperforms the state of art methods in terms of different measures.
更多查看译文
关键词
Breast cancer, digital mammograms, deep learning, wavelet neural network, Resnet 34, disease diagnosis
AI 理解论文
溯源树
样例
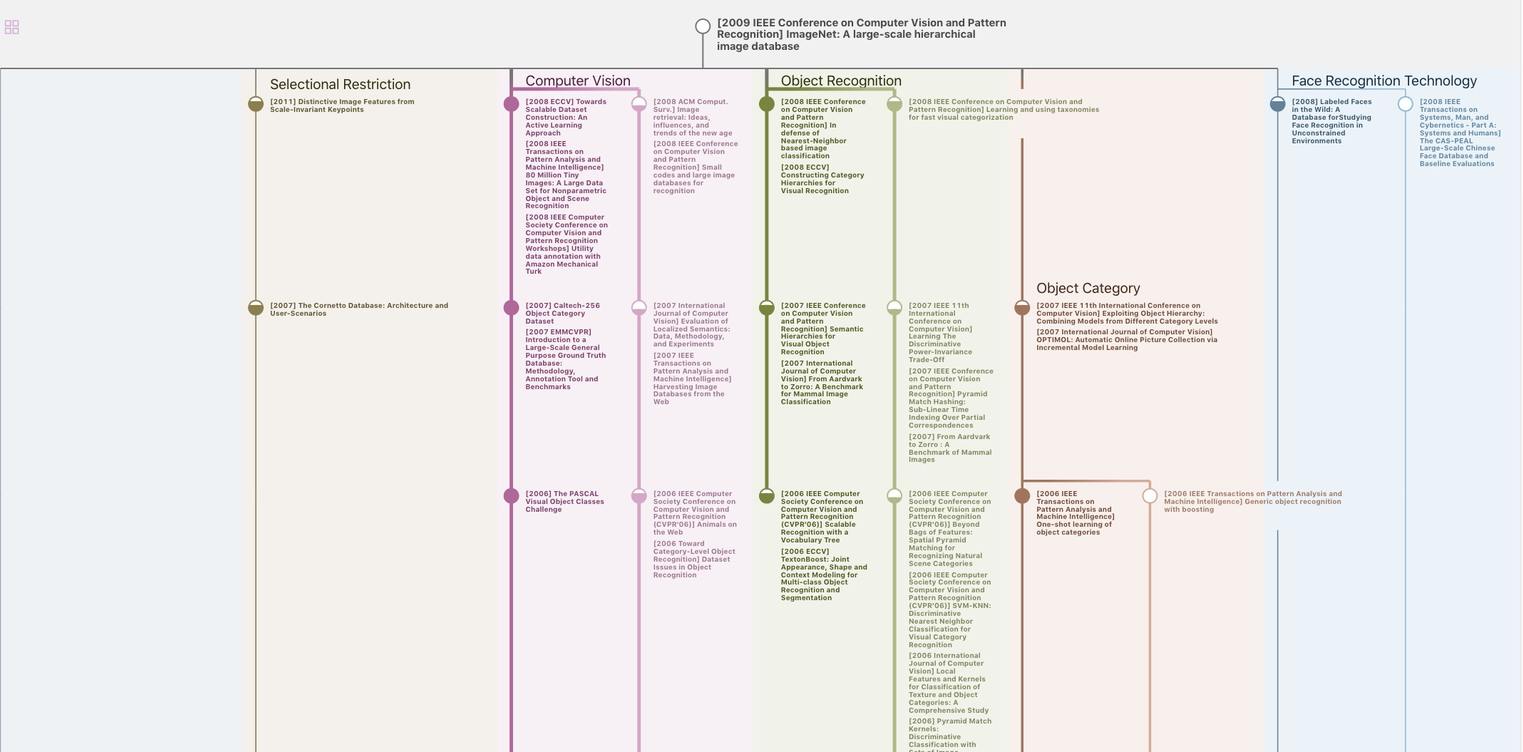
生成溯源树,研究论文发展脉络
Chat Paper
正在生成论文摘要