Forecasting Future Product Sequences To Be Processed In Tire Production Using Deep Learning Technique
Procedia Computer Science(2023)
摘要
Production sequencing methodology using DeepLearning Seq2Seq-LSTM is applied to a tire production case study in Quebec, Canada. Production and demand data are used to predict the most likely product sequences to operate. The comparison of 4 forecasting models, differing in consideration of demand and a statistical component, leads to nearly 70% of good prediction when all machines are studied and 10 production scenarios are considered. This performance reaches 92% for a specific class of machines. The analysis of the forecasts by class of machine allows highlighting 2 factors influencing the performance of the models, namely the ratio of product/machine by class and the total number of available records. The forecasts of possible production scenarios can then be used in a digital twin to evaluate a reasonable number of options and develop a decision support system for production sequencing.
更多查看译文
关键词
tire production,future product sequences,deep learning technique,deep learning
AI 理解论文
溯源树
样例
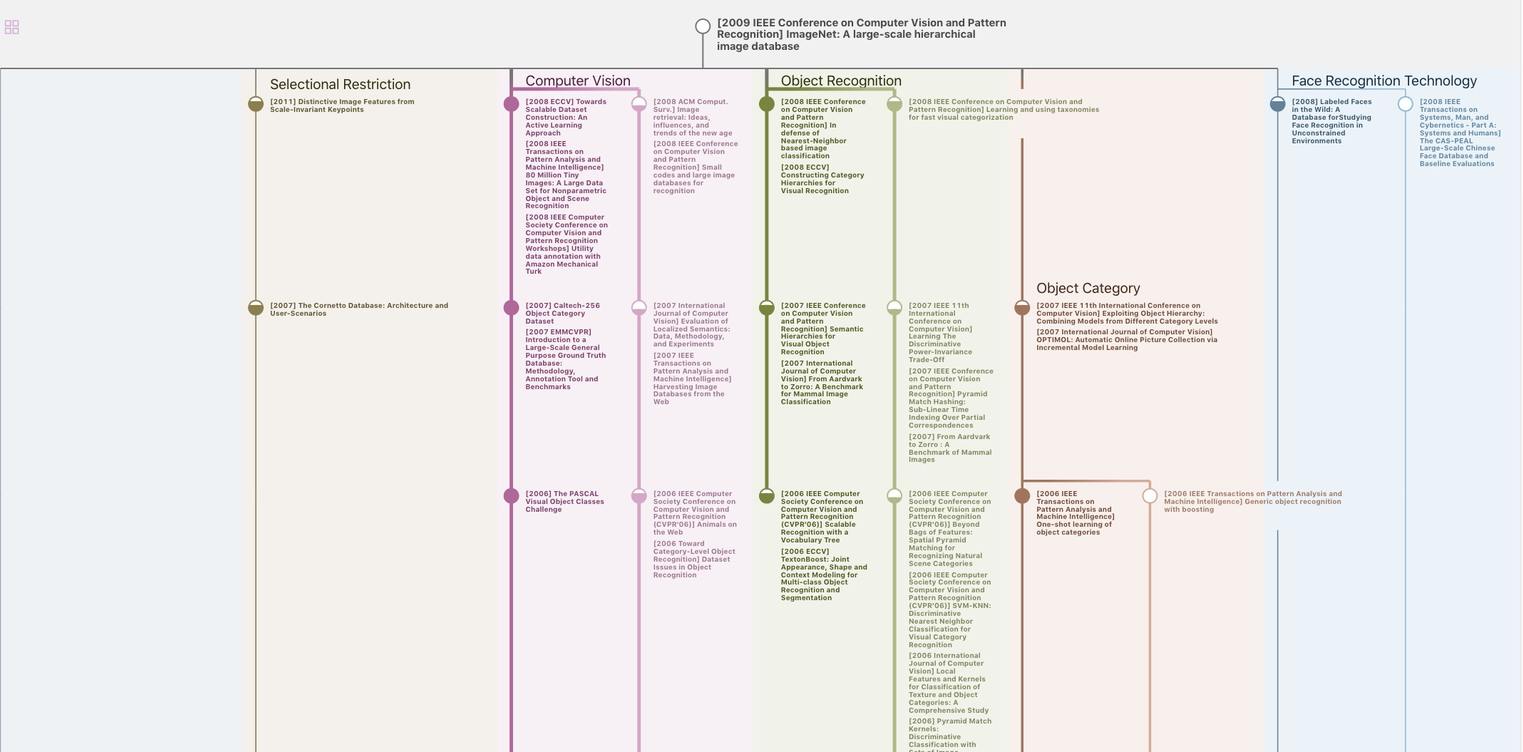
生成溯源树,研究论文发展脉络
Chat Paper
正在生成论文摘要