CausalViT: Domain Generalization for Chemical Engineering Process Fault Detection and Diagnosis
PROCESS SAFETY AND ENVIRONMENTAL PROTECTION(2023)
摘要
Fault detection and diagnosis (FDD) is a promising technology for safe operation, quality control, and profitability improvement in chemical process systems. In practice, chemical process systems usually switch among operating conditions in response to market demands, fatigue, malfunctions of components and environments. However, FDD models often deteriorate when transferred from one operating condition to another. Current widely used FDD models are trained and tested in identical domains (or operating conditions) without emphasis on the capability of domain generalization. Furthermore, they ignore the causality that remains invariant among domains but excessively rely on statistical correlation that varies from domain to domain. This paper presents an FDD model named CausalViT. Causality and a gradient reversal operation are designed to enhance domain generalization. In CausalViT, a Causal Layer is constructed to discover the causal mechanism in features to purify superfluous domain-specific statistical correlation. Then, a domain classifier cooperating with gradient reversal layers is developed to further enhance the domain invariance. To evaluate the CausalViT, comparisons have been made between it and other advanced FDD models in six domains/modes in Tennessee Eastman Process. CausalViT shows superiority from the perspectives of both fault detection ratio and domain generalization, obtaining an average 16% improvement on domain generalization. Dimension reduction visualization shows the significance of domain generalization and gives a deeper insight into CausalViT. Furthermore, the convergence of the model and the effectiveness of components in the model have been analyzed and demonstrated.
更多查看译文
关键词
Causality inference,Domain generalization,Fault Detection and Diagnosis,Gradient reversal operation,Tennessee Eastman process,Transformer
AI 理解论文
溯源树
样例
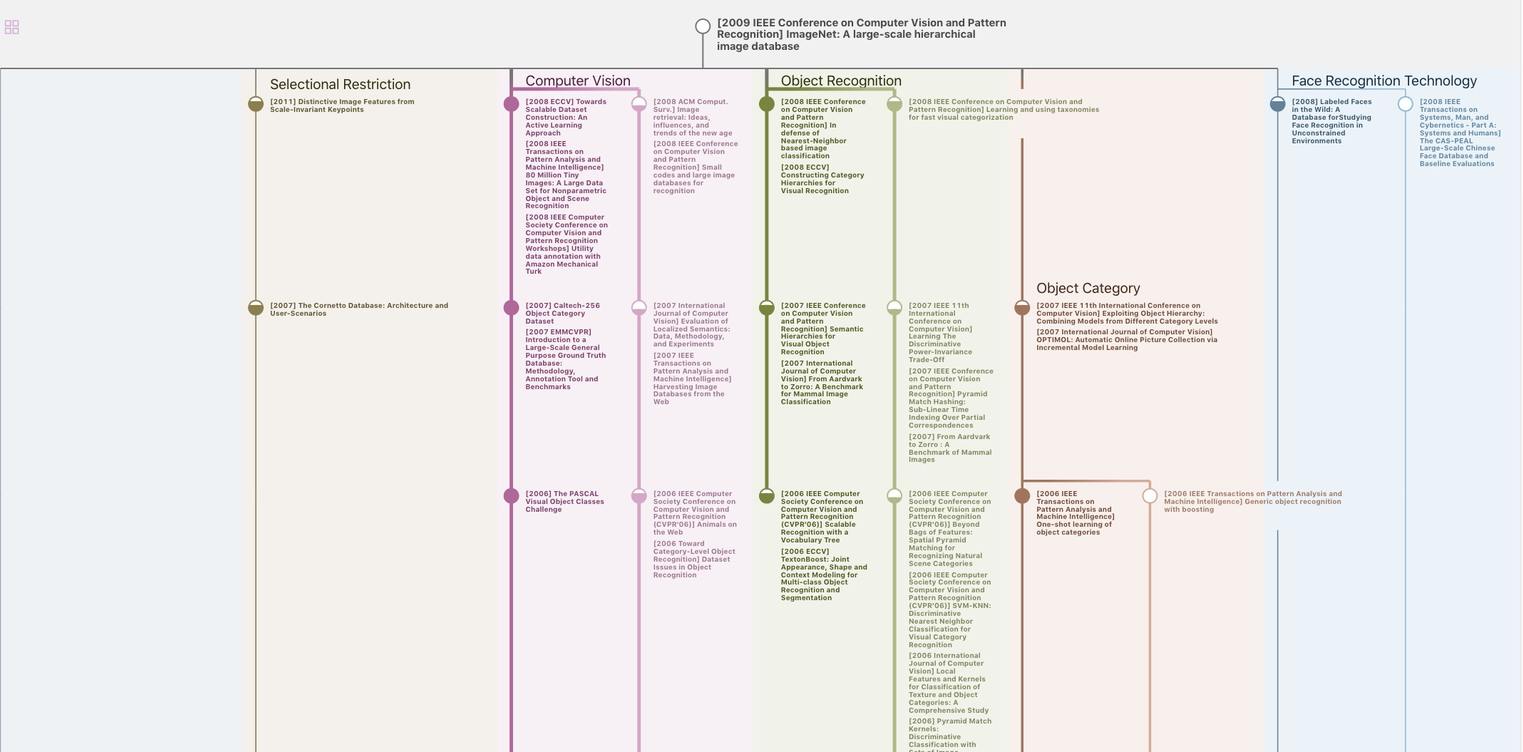
生成溯源树,研究论文发展脉络
Chat Paper
正在生成论文摘要