Gated recurrent unit-enhanced deep convolutional neural network for real-time industrial process fault diagnosis
Process Safety and Environmental Protection(2023)
摘要
When deep learning-based models are employed for the fault diagnosis of chemical processes, problems of poor calculation accuracy and efficiency often occur in the scenarios of high-dimensional, nonlinear, and time-varying data, which affects the overall robustness of the fault diagnosis model. This study proposes a novel Gated Recurrent Unit (GRU) - enhanced deep convolutional neural network (EDCNN) model for the improved fault detection and diagnosis of chemical processes. In the GRU-EDCNN model, a maximum smooth function (MSF) is developed and presented to replace the classical activation function for better matching the input data format, which can significantly improve the calculation accuracy and the overall efficiency of DCNN models. Moreover, the convolutional layers in DCNN are optimized by a decentralized convolutional structure, thereby reducing the problem of parameter redundancy. The issue of gradient disappearance is tackled by the embedment of GRU, whose ability to control time series features and gateway information can ensure that no overfitting occurs in the GRU-EDCNN training processes. In two case studies, the GRU-EDCNN model was applied to the benchmark Tennessee Eastman (TE) process and an acid gas absorption process, and the corresponding data of fault diagnosis rate (FDR), false positive rate (FPR), and fault diagnosis time (FDT) of the GRU-EDCNN model were analyzed and compared with those of the other deep learning-based models, verifying the effectiveness of the GRU-EDCNN model for fault diagnosis in both simulated and real-time industrial processes.
更多查看译文
关键词
deep convolutional neural network,neural network,unit-enhanced,real-time
AI 理解论文
溯源树
样例
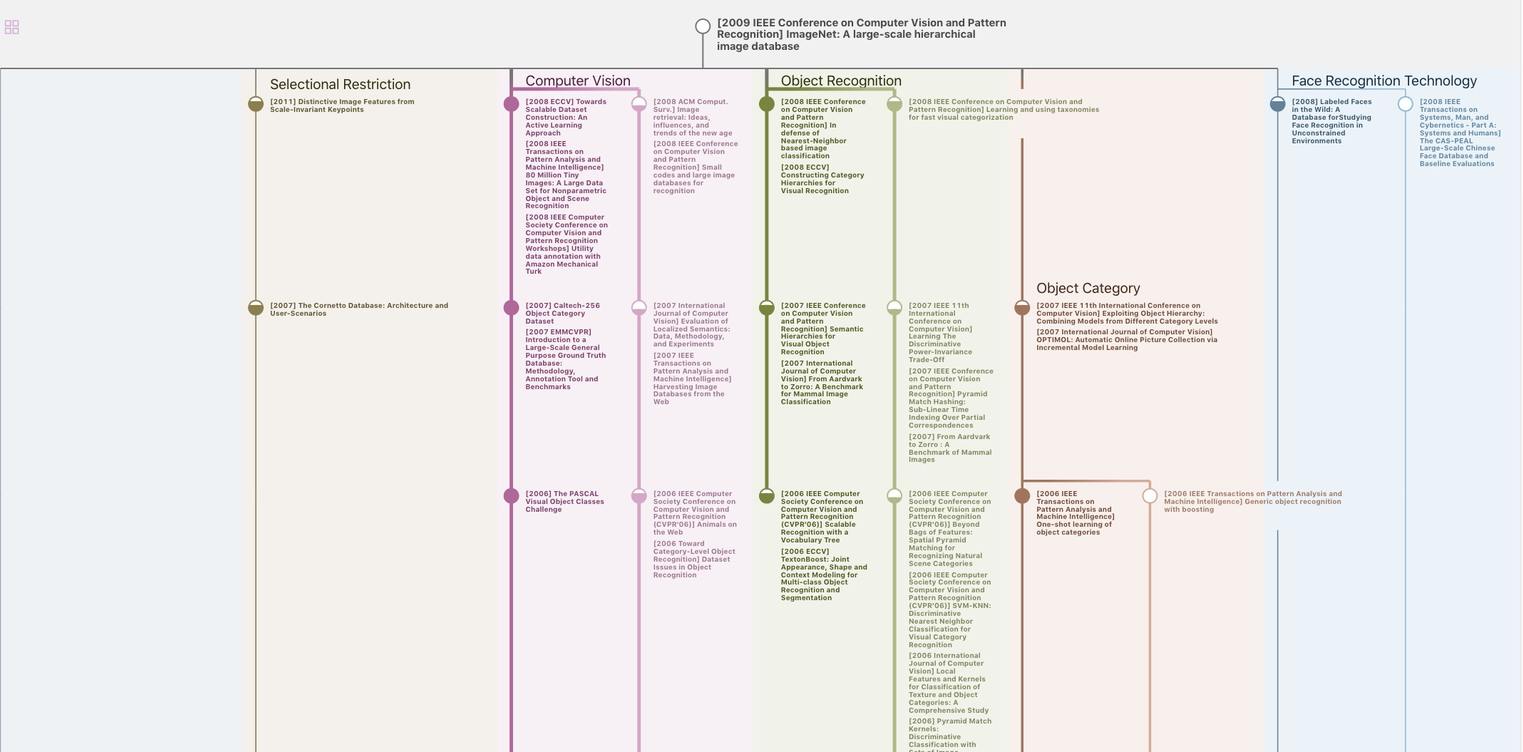
生成溯源树,研究论文发展脉络
Chat Paper
正在生成论文摘要