A generalized adaptive robust distance metric driven smooth regularization learning framework for pattern recognition
Signal Processing(2023)
摘要
•A novel generalized adaptive robust distance metric is presented, called Lδ(u).•The Lδ(u) has some desirable salient properties, such as symmetry, boundedness, robustness, nonconvexity, and adaptivity, with both first-order and higher-order moments from samples.•The Lδ(u) can pick different robust distance metrics for different learning tasks during the learning process by the adaptive parameter δ.•A smooth regularized TELM learning framework for supervised and semi-supervised classification is proposed by introducing Lδ(u), regularization and smoothing techniques.•The fast Newton-Armijo algorithm and DC (difference of convex functions) programming algorithm (DCA) are used to solve the proposed methods.
更多查看译文
关键词
Generalized adaptive robust distance metric, Twin extreme learning machine, Manifold regularization, Robust classification, Newton-Armijo algorithm, DC programming algorithm
AI 理解论文
溯源树
样例
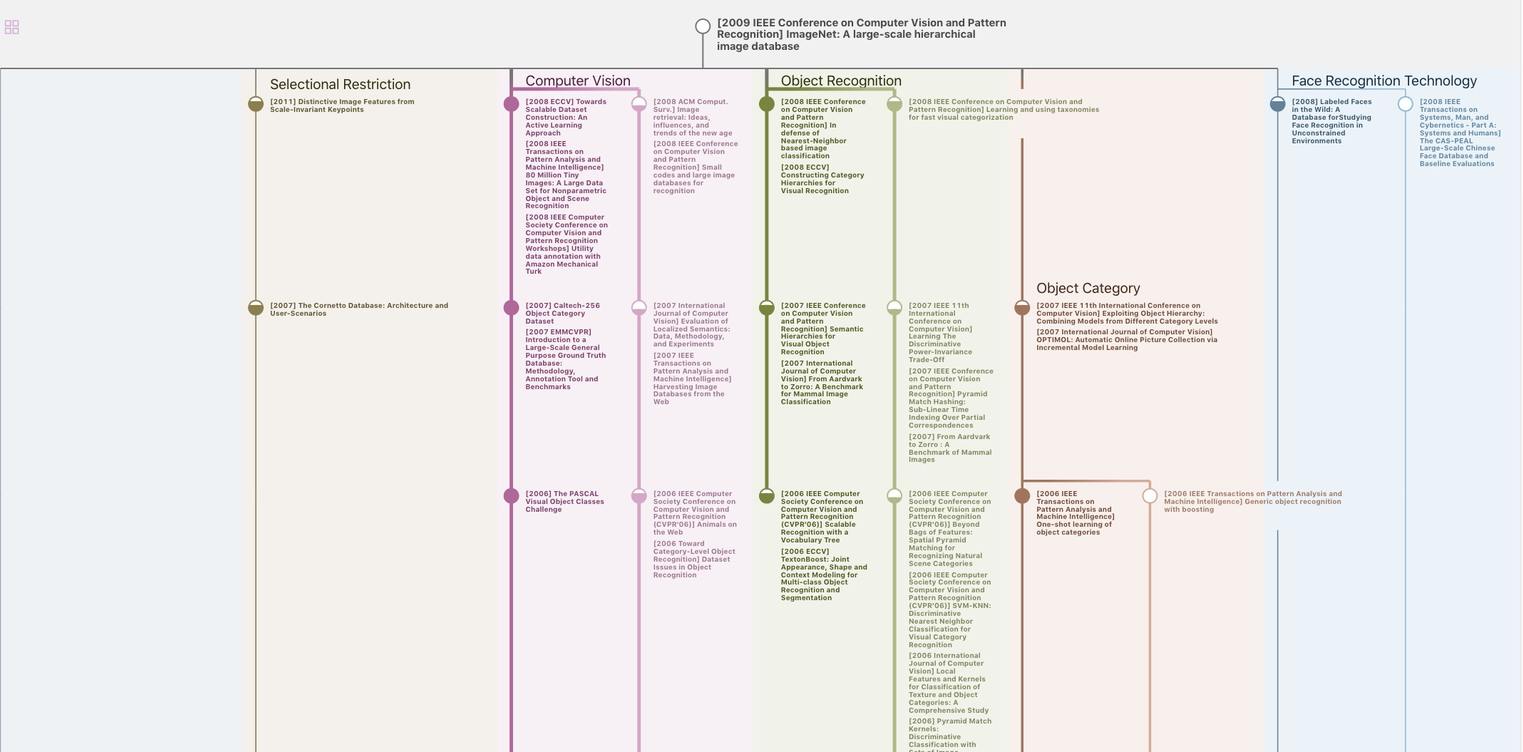
生成溯源树,研究论文发展脉络
Chat Paper
正在生成论文摘要