Ultra-short-term multi-step probability interval prediction of photovoltaic power: A framework with time-series-segment feature analysis
SOLAR ENERGY(2023)
摘要
Power prediction can effectively mitigate the uncertainty in photovoltaic power generation, enabling better operation and scheduling of power grids. Therefore, in this study, a multi-step interval prediction method for ultra-short-term photovoltaic power from time-series-segment (TSS) feature analysis is proposed. First, three TSS indicators are defined to determine the fluctuation characteristics of historical data and combined with fuzzy Cmeans clustering to address the time mismatch problem. Subsequently, a deterministic multi-step prediction method is proposed based on the optimal membership search using deep recurrent neural networks, improving the prediction stability. Finally, based on the difference in the TSS types, an improved interval prediction method is proposed in combination with Gaussian process regression, narrowing the average interval width. Experiments are conducted to compare the performances of the conventional and proposed methods using measured data from Australia. Compared with the baseline scheme, the proposed scheme enhances the accuracy of multi-step prediction by 19.7%, and the average error of each step does not exceed 5%.The average interval width is reduced by 45.6% while ensuring more than 95% interval coverage in the probability interval prediction. The experimental findings demonstrate that the TSS feature analysis can effectively reveal the potential patterns of PV power output under various weather conditions. This enables the algorithm to learn clearer sample features and thus enhances the performance of multi-step probability interval prediction.
更多查看译文
关键词
Photovoltaic power prediction,Probability interval prediction,Fuzzy C-means clustering,Deep recurrent neural network,Gaussian process regression
AI 理解论文
溯源树
样例
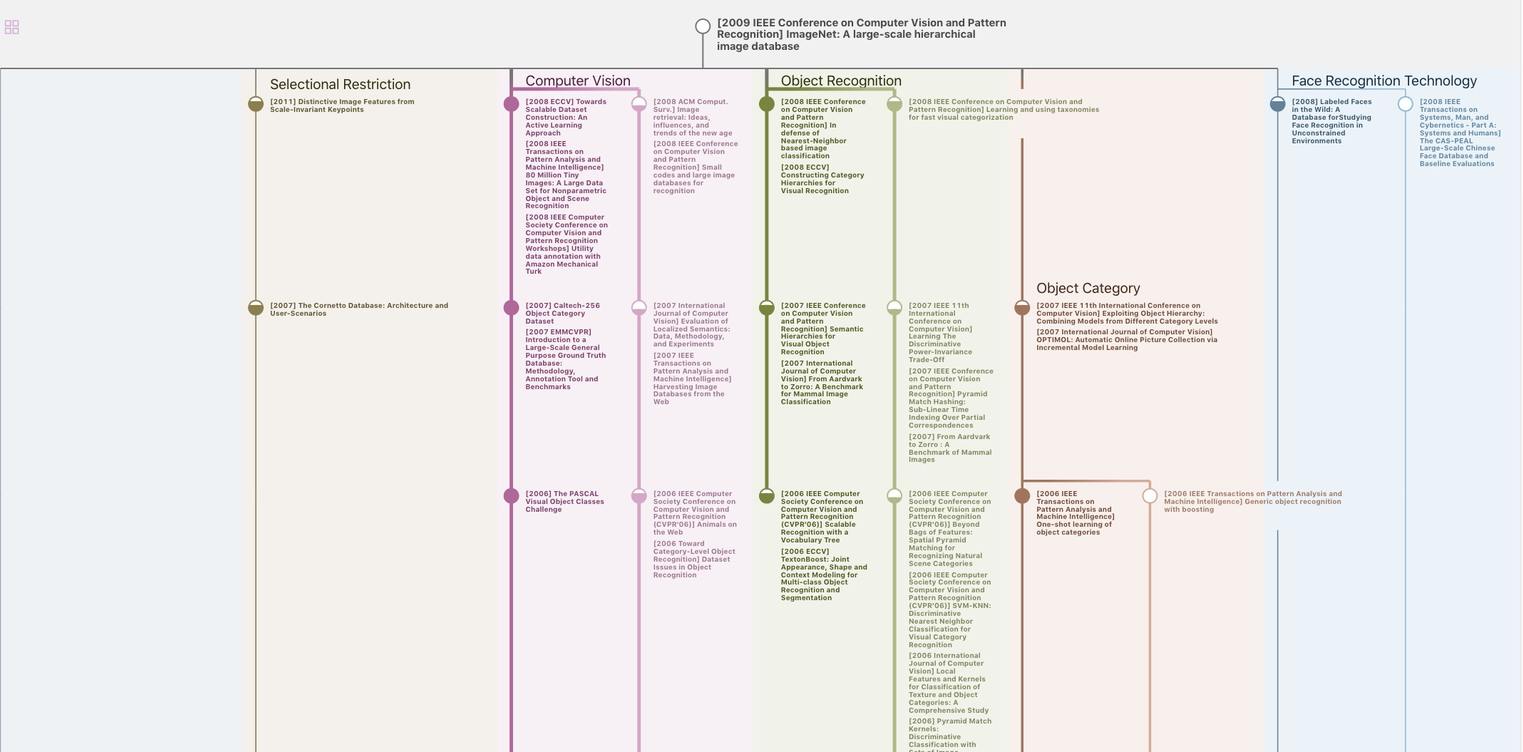
生成溯源树,研究论文发展脉络
Chat Paper
正在生成论文摘要