Noise effects analysis on subspace-based damage detection with neural networks
Structures(2023)
摘要
Structural health monitoring (SHM) is nowadays a significant and topical issue in the civil engineering field, whose first-level task is the damage detection job. Recent advances in artificial intelligence (AI) combined with non-destructive assessments based on output-only vibration signals represent the frontier of SHM. The present research study has handled an AI-based multi-class damage classification task on a numerical benchmark beam problem with three different procedures. The main purpose was to investigate the noise effects of the analyzed damage detection procedures simulating real-world accelerometer sensors. The first damage identification method leverages simple statistical features of vibration signals, whereas the second method additionally considers a subspace-based damage indicator. The third method relies on a dataset composed of a specific set of damage indicators. Two deep learning (DL) techniques have been adopted to compare and verify the results, i.e. a multi-layer perceptron (MLP) and a one-dimensional convolutional network (1D-CNN). Three groups of different reasonable signal-to-noise ratio (SNR) levels were set up to simulate micro electro-mechanical system (MEMS) accelerometer sensors in real-world conditions to evaluate noise effects. In a nutshell, the results demonstrated that the DL models based on stochastic subspace identification (SSI) methods have good noise immunity performance for damage detection purposes.
更多查看译文
关键词
Structural health monitoring,Subspace-based damage indicators,Signal-to-noise ratio,Multi-layer perceptron,Convolutional neural network
AI 理解论文
溯源树
样例
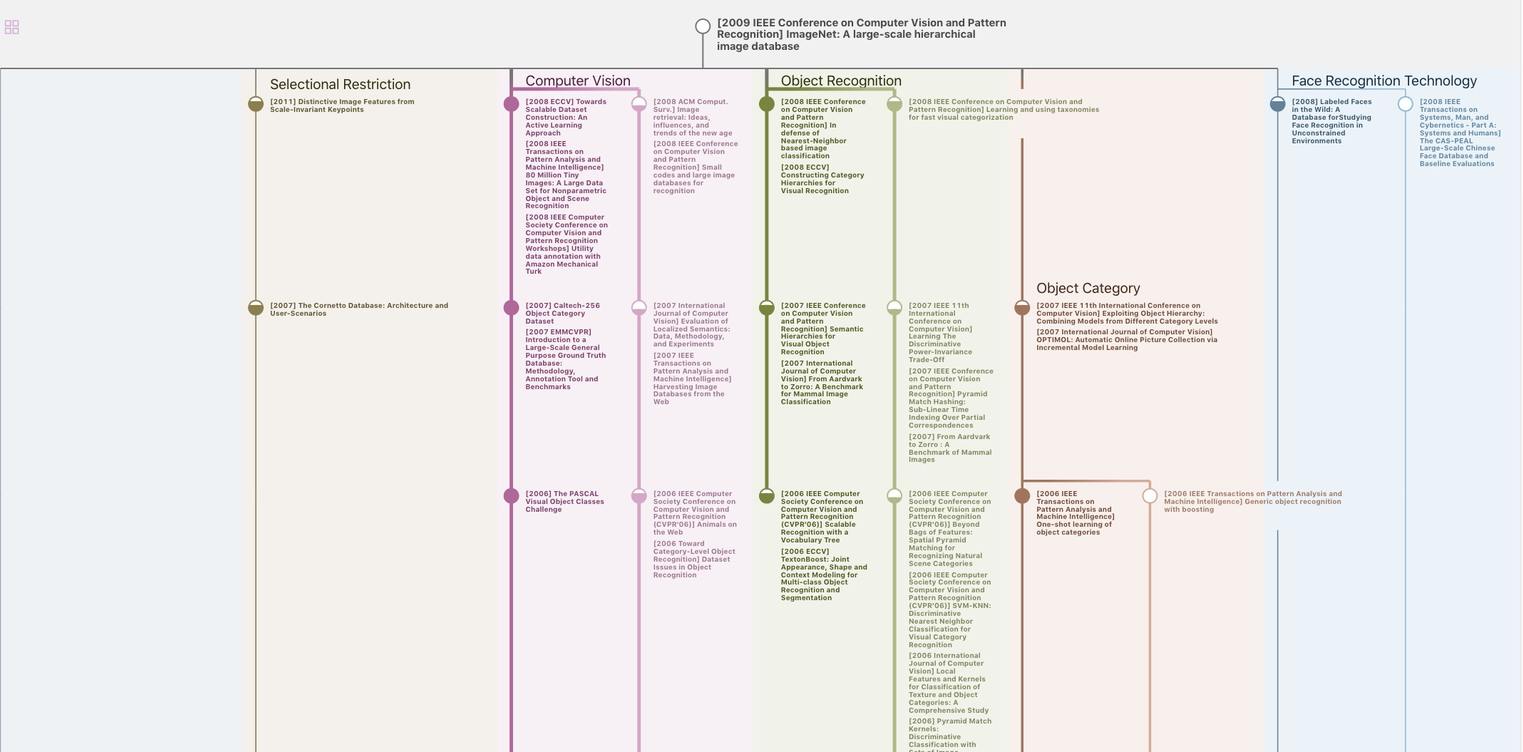
生成溯源树,研究论文发展脉络
Chat Paper
正在生成论文摘要