Development of Predictive Neural Network with Historical and Geometrical Inputs to Minimize Energy Consumption in Solar-Fed Wireless Sensor Networks
SUSTAINABLE ENERGY GRIDS & NETWORKS(2023)
摘要
The effective operation of solar-supplied wireless sensor nodes is reached when a constant function with minimum energy consumption occurs. Accordingly, energy consumption management requires prior prediction of solar energy income. The accuracy of prediction contributes largely to maximizing or minimizing the energy consumed in those nodes. The moving average prediction algorithms show considerable errors due to sudden weather fluctuations. Additionally, the neural networks are applied only with historical inputs till now, accompanied by insufficient precision. Thus, they all prevent working effectively. This paper develops a predictive neural network with higher accuracy than the moving average algorithms and the neural networks of historical inputs. The developed neural network uses the zenith angle as additional geometrical input to the existing historical ones. The results showed a Mean Bias Error (MBE) of (0.52%) for the developed neural network. while (EWMA, WCMA, Pro-Energy, NN of 2 historical inputs, NN of 5 historical inputs, and NN of 19 historical inputs) appeared at (11.92%, 8.59%, 6.34%, 4.33%, 2.56%, and 1.08%), respectively. For a simulation period of one week, the developed neural network eliminated three hours of operation within the high consumption state (S3) in favor of the lower consumption state (S2) which maintains the constant function.
更多查看译文
关键词
Wireless Sensor Networks (WSNs),Energy Management,Global Irradiance (GI),Solar Energy Harvesting,Neural Networks,Prediction Algorithms
AI 理解论文
溯源树
样例
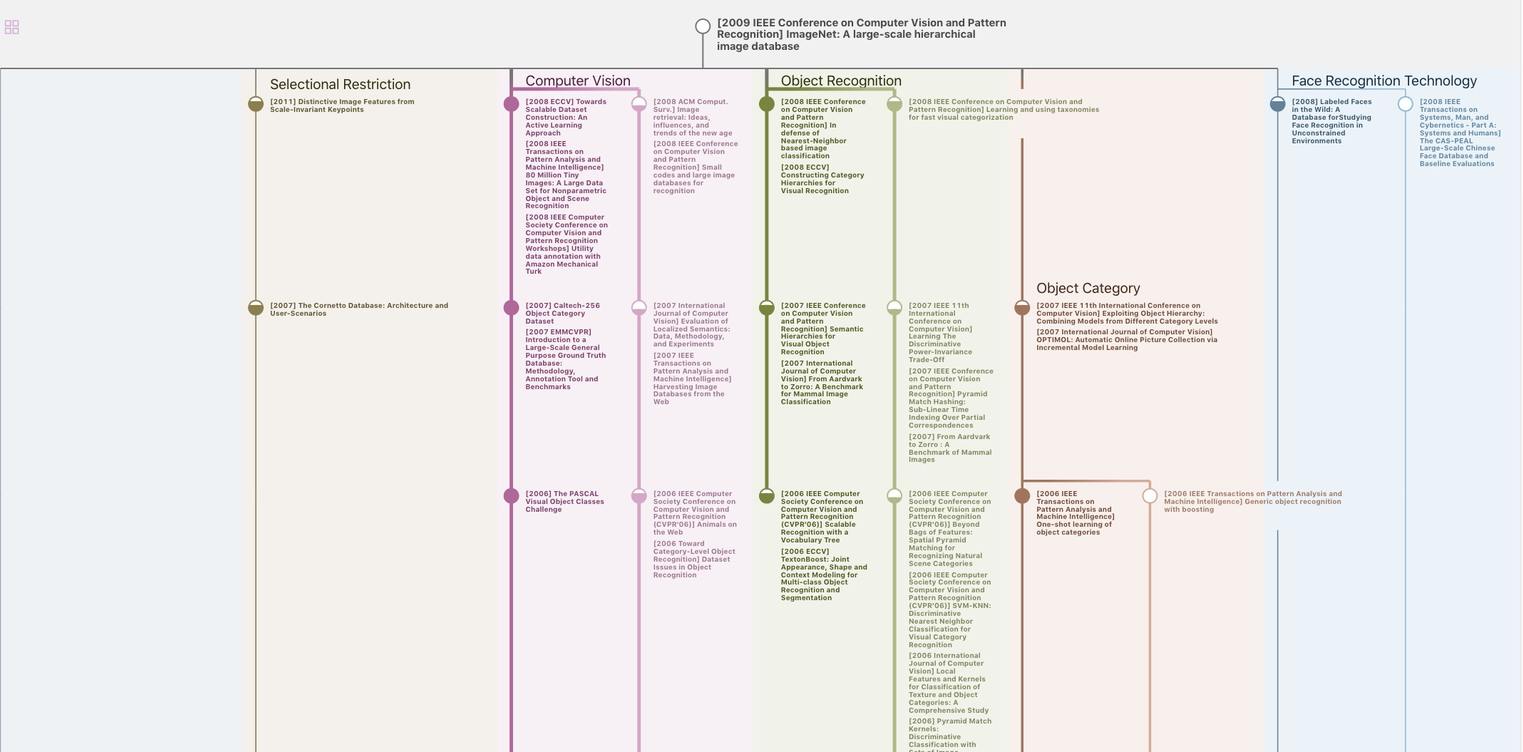
生成溯源树,研究论文发展脉络
Chat Paper
正在生成论文摘要