Empirical Analysis of a Segmentation Foundation Model in Prostate Imaging
MEDICAL IMAGE COMPUTING AND COMPUTER ASSISTED INTERVENTION, MICCAI 2023 WORKSHOPS(2023)
关键词
Foundation model,Medical Image Segmentation,Prostate MRI,In-context Learning
AI 理解论文
溯源树
样例
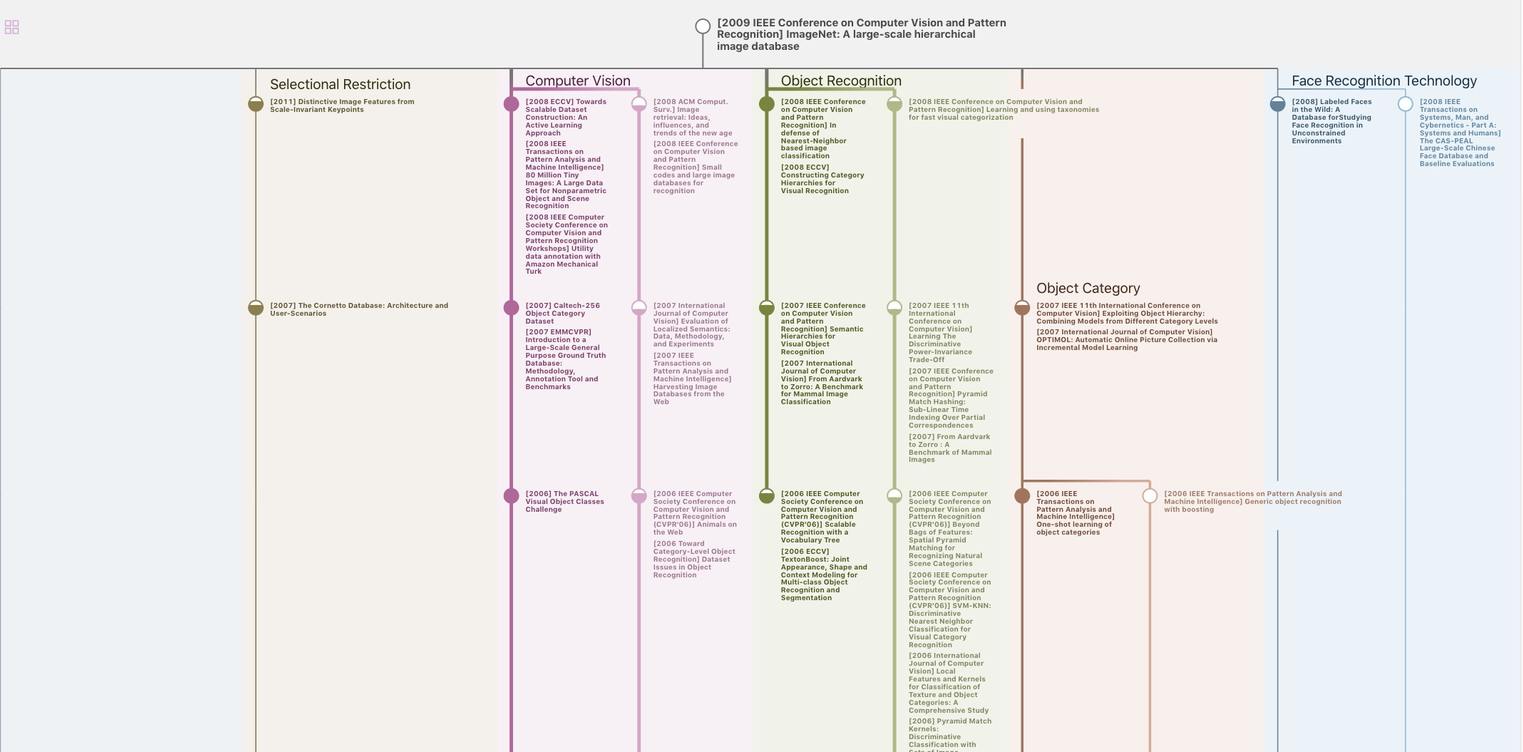
生成溯源树,研究论文发展脉络
Chat Paper
正在生成论文摘要