Spectrally Constrained Optimization
arXiv (Cornell University)(2023)
摘要
We investigate how to solve smooth matrix optimization problems with general linear inequality constraints on the eigenvalues of a symmetric matrix. We present solution methods to obtain exact global minima for linear objective functions, i.e., $F(X) = \langle C, X \rangle$, and perform exact projections onto the eigenvalue constraint set. Two first-order algorithms are developed to obtain first-order stationary points for general non-convex objective functions. Both methods are proven to converge sublinearly when the constraint set is convex. Numerical experiments demonstrate the applicability of both the model and the methods.
更多查看译文
关键词
optimization
AI 理解论文
溯源树
样例
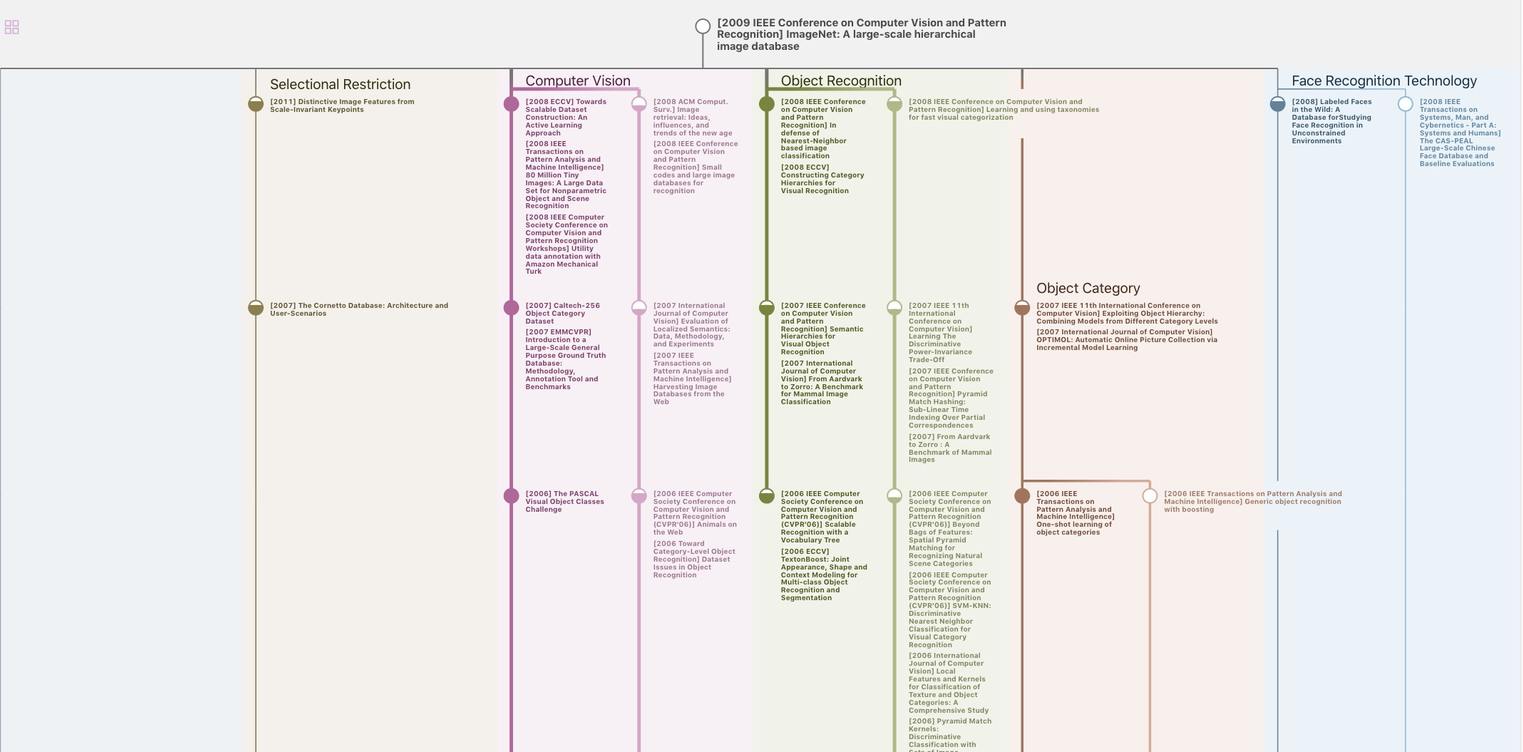
生成溯源树,研究论文发展脉络
Chat Paper
正在生成论文摘要