Can Variational Quantum Algorithms Demonstrate Quantum Advantages? Time Really Matters
arXiv (Cornell University)(2023)
摘要
Applying low-depth quantum neural networks (QNNs), variational quantum algorithms (VQAs) are both promising and challenging in the noisy intermediate-scale quantum (NISQ) era: Despite its remarkable progress, criticisms on the efficiency and feasibility issues never stopped. However, whether VQAs can demonstrate quantum advantages is still undetermined till now, which will be investigated in this paper. First, we will prove that there exists a dependency between the parameter number and the gradient-evaluation cost when training QNNs. Noticing there is no such direct dependency when training classical neural networks with the backpropagation algorithm, we argue that such a dependency limits the scalability of VQAs. Second, we estimate the time for running VQAs in ideal cases, i.e., without considering realistic limitations like noise and reachability. We will show that the ideal time cost easily reaches the order of a 1-year wall time. Third, by comparing with the time cost using classical simulation of quantum circuits, we will show that VQAs can only outperform the classical simulation case when the time cost reaches the scaling of $10^0$-$10^2$ years. Finally, based on the above results, we argue that it would be difficult for VQAs to outperform classical cases in view of time scaling, and therefore, demonstrate quantum advantages, with the current workflow. Since VQAs as well as quantum computing are developing rapidly, this work does not aim to deny the potential of VQAs. The analysis in this paper provides directions for optimizing VQAs, and in the long run, seeking more natural hybrid quantum-classical algorithms would be meaningful.
更多查看译文
关键词
quantum
AI 理解论文
溯源树
样例
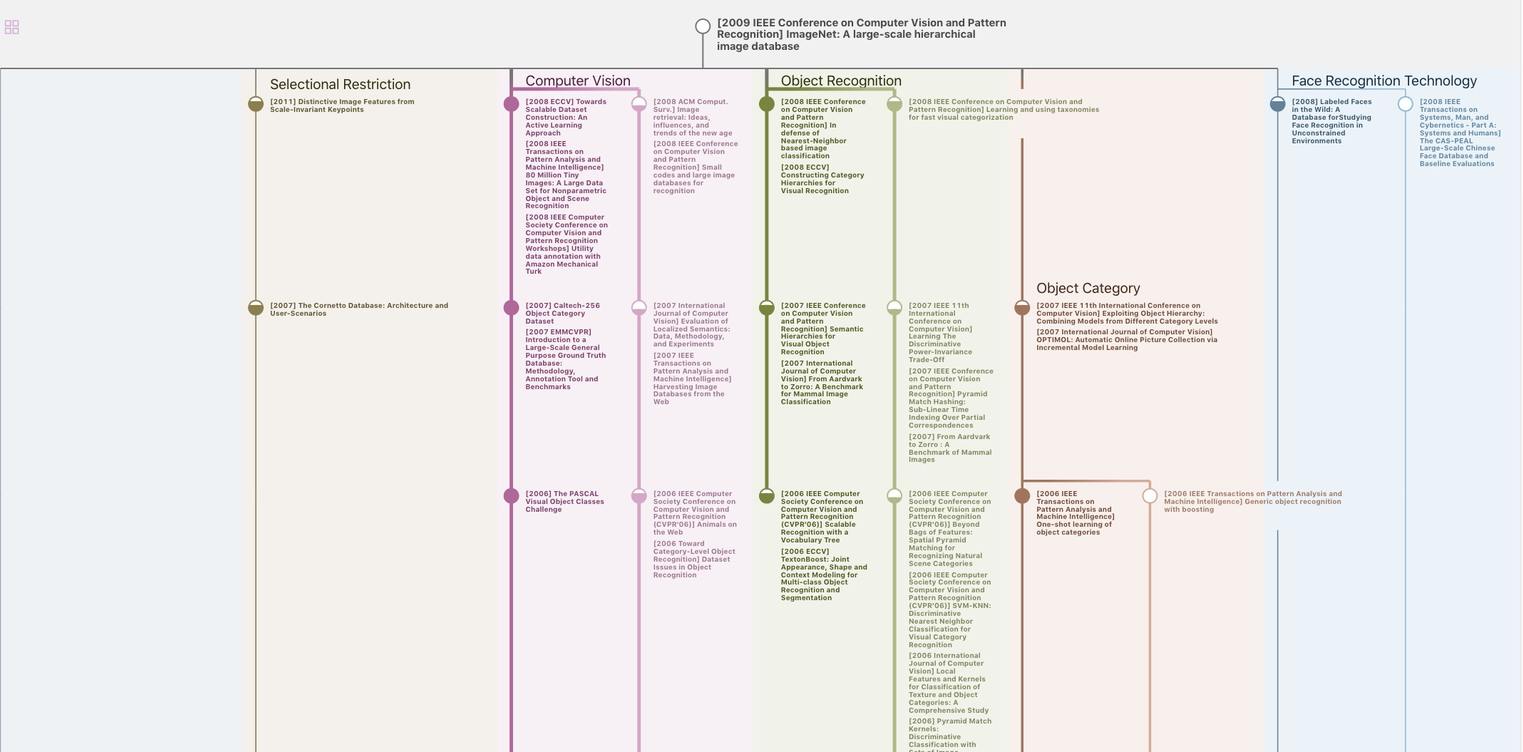
生成溯源树,研究论文发展脉络
Chat Paper
正在生成论文摘要