CoactSeg: Learning from Heterogeneous Data for New Multiple Sclerosis Lesion Segmentation
MEDICAL IMAGE COMPUTING AND COMPUTER ASSISTED INTERVENTION, MICCAI 2023, PT VIII(2023)
摘要
New lesion segmentation is essential to estimate the disease progression and therapeutic effects during multiple sclerosis (MS) clinical treatments. However, the expensive data acquisition and expert annotation restrict the feasibility of applying large-scale deep learning models. Since single-time-point samples with all-lesion labels are relatively easy to collect, exploiting them to train deep models is highly desirable to improve new lesion segmentation. Therefore, we proposed a coaction segmentation (CoactSeg) framework to exploit the heterogeneous data (i.e., new-lesion annotated two-time-point data and all-lesion annotated single-time-point data) for new MS lesion segmentation. The CoactSeg model is designed as a unified model, with the same three inputs (the baseline, follow-up, and their longitudinal brain differences) and the same three outputs (the corresponding all-lesion and new-lesion predictions), no matter which type of heterogeneous data is being used. Moreover, a simple and effective relation regularization is proposed to ensure the longitudinal relations among the three outputs to improve the model learning. Extensive experiments demonstrate that utilizing the heterogeneous data and the proposed longitudinal relation constraint can significantly improve the performance for both new-lesion and all-lesion segmentation tasks. Meanwhile, we also introduce an in-house MS-23v1 dataset, including 38 Oceania single-time-point samples with all-lesion labels. Codes and the dataset are released at https://github.com/ycwu1997/CoactSeg.
更多查看译文
关键词
Multiple Sclerosis Lesion,Longitudinal Relation,Heterogeneous Data
AI 理解论文
溯源树
样例
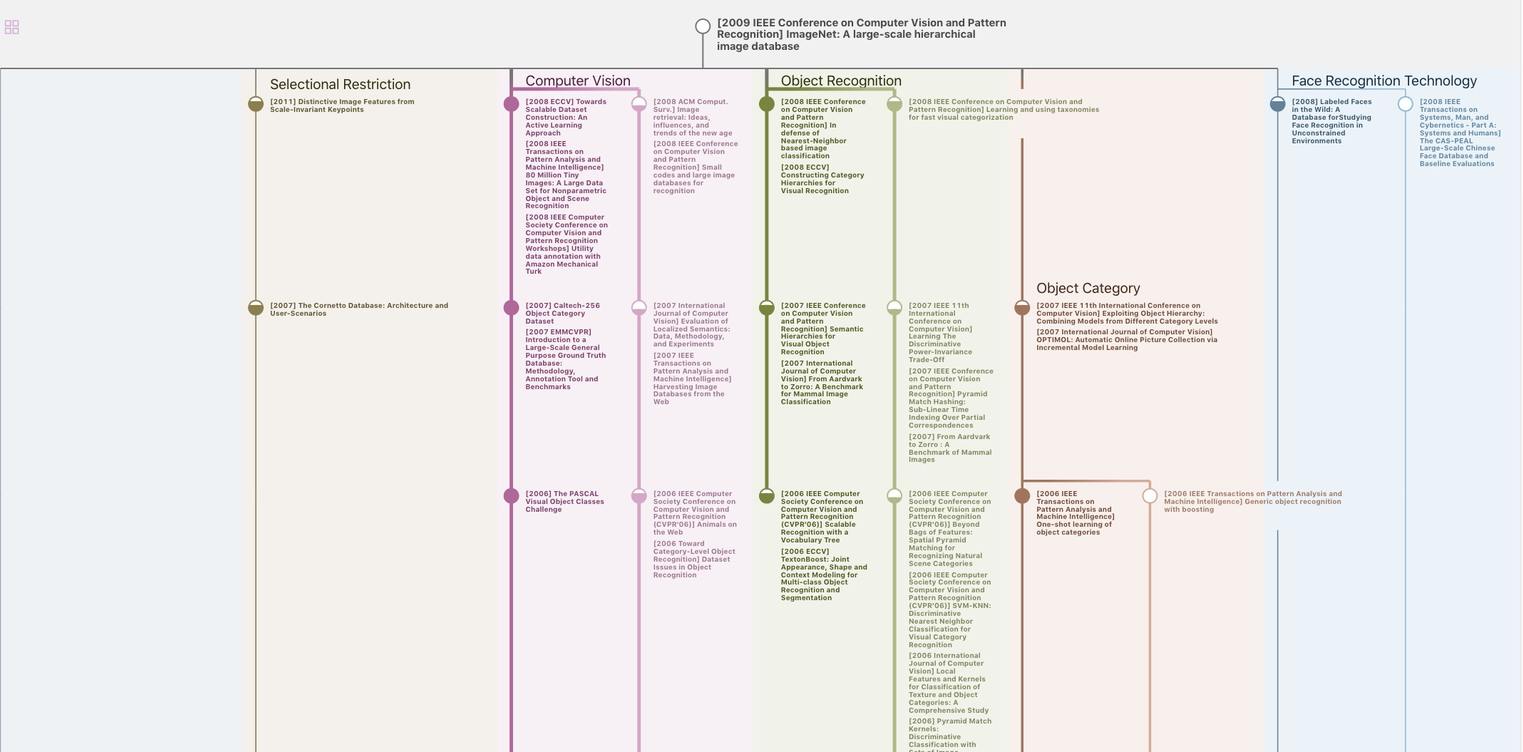
生成溯源树,研究论文发展脉络
Chat Paper
正在生成论文摘要